Faculty Profile
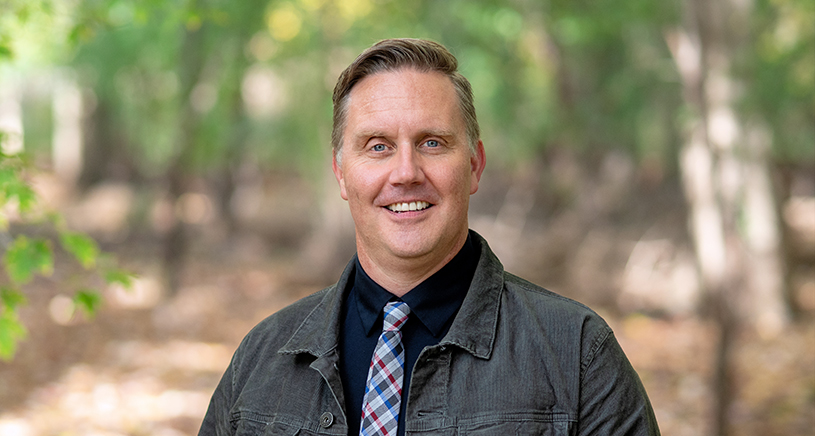
Thomas M Braun, PhD
- Professor, Biostatistics
- Member, Senate Advisory Committee on University Affairs (SACUA)
Dr. Braun is an international expert in the design and application of Bayesian adaptive clinical trials, primarily focused on discovery of safe and effective chemotherapy and immunotherapy regimens for treatment and prevention of cancer. Dr. Braun is also developing novel clinical trial designs for identifying new treatments for rare diseases, as well as exploring Bayesian methods for incorporating patient preference into traditional randomized clinical trials (RCTs) and sequential multiple assignment randomized trials (SMARTs). More recently, Dr. Braun is exploring how Bayesian methods can be applied to merging of electronic health records (EHR) with data collected from RCTs, with the goal of producing RCTs that are more efficient and require less patient enrollment.
- PhD, University of Washington, Seattle, 1999
- MS, University of Washington, Seattle, 1996
- BBA, University of Wisconsin, Madison, 1990
Research Interests:
Adaptive clinical trials, Bayesian methods, longitudinal data, oncology, rare diseases,
dyadic analyses, mobile health technology
Research Projects:
Through a funded contract with the Food and Drug Administration (FDA), Dr. Braun is
developing novel Bayesian clinical trial designs for discovering treatments for rare
diseases.
Through a funded contract with the Patient Centered Outcomes Research Institute (PCORI), Dr. Braun is developing Bayesian methods for incorporating patient preference in Sequential Multiple Assignment Randomized Trials (SMARTs).
Dr. Braun is collaborating with investigators on a study to examine potential disparities in the who is prescribed approved immunotherapy regimens for treatment of melanoma, lung, and genitourinary cancer.
Dr. Braun is collaborating on multiple projects that examine the use of Roadmap, which is a series of wellbeing apps developed at the University of Michigan, to improve the mental and physical health of recipients of stem-cell transplants, as well as their caregivers, and to explore the dyadic interactions between caregivers and their care recipients.
Braun TM. A Simulation-Free Approach to Assessing the Performance of the Continual Reassessment Method. Statistics in Medicine (39): 4651-4666, 2020.
Wei B, Braun TM, Tamura R, Kidwell K. Sample Size Determination for Bayesian Analysis of small n Sequential, Multiple Assignment, Randomized Trials (snSMARTs) with Three Agents. Journal of Biopharmaceutical Statistics. DOI: 10.1080/10543406.2020.1815032, 2020.
Braun TM and Mercier F. Extending the Continual Reassessment Method to Accommodate Step-Up Dosing. Statistics in Medicine, 2022 (in press).
Chao Y-C, Braun T, Tamura R, Kidwell, K. Power Prior Models for Treatment Effect Estimation in a Small n, Sequential, Multiple Assignment, Randomized Trial. Statistical Methods in Medical Research, 2022 (in press).
Fang F, Braun TM, Tamura R, and Kidwell K. Bayesian Methods to Compare Dose Levels to Placebo in a Small n, Sequential, Multiple Assignment, Randomized Trial (snSMART) with a Continuous Outcome. Statistics in Biopharmaceutical Research, 2022 (in press).
View full list of publications at PubMed (https://pubmed.ncbi.nlm.nih.gov/)
M4063 SPH II
1415 Washington Heights,
Ann Arbor, MI 48109
Email: [email protected]
Phone: 734-936-9844
Fax: 734-763-2215
For media inquiries: [email protected]
Areas of Expertise: Biostatistics, Cancer, Child Health, Clinical Trials, Maternal Health