Faculty Profile
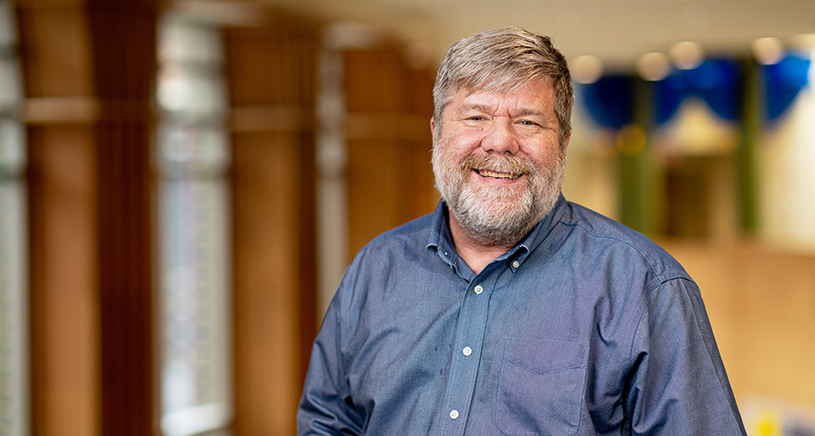
Michael R. Elliott, PhD
- Professor, Biostatistics
- Research Professor, Survey Methodology
Michael Elliott is a Professor of Biostatistics at the University of Michigan School of Public Health and Research Professor at the Institute for Social Research. He received his PhD in biostatistics in 1999 from the University of Michigan. Prior to joining the University of Michigan in 2005, he held an appointment as an Assistant Professor at the Department of Biostatistics and Epidemiology at the University of Pennsylvania School of Medicine. Dr. Elliott's statistical research interests focus around the broad topic of "missing data," including the design and analysis of sample surveys, causal and counterfactual inference, and latent variable models. He has worked closely with collaborators in injury research, pediatrics, women's health, the social determinants of physical and mental health, and smoking cessation research. Dr. Elliott has served as an Associate Editor for the Journal of the Royal Statistical Society, Series C and the Journal of the American Statistical Association, and as an Associate Editor and Editor of the Journal of Survey Statistics and Methodology. He was Associate Chair of Academic Affairs for the Department from 2018-2021.
- PhD, University of Michigan, 1999
- MS, University of Michigan, 1997
- BA, University of Chicago, 1985
- Survey design and analysis, missing data, causal inference, longitudinal data
- Developing model-based Bayesian approaches that complement traditional design-based analyses of complex sample survey data.
- Combining probability and non-probability samples.
- Developing methods to assess surrogate markers using causal inference (determining to what degree easy-to-observe biomarkers are on causal pathway for outcomes that are expensive or time-consuming to observe).
- Developing methods to adjust for bias due to "treatment by indication" in observational settings, where preliminary outcomes may drive treatment decisions.
- Improving the generalizability of clinical trials by incorproating information from relevant probability samples.
- Developing models that focus on variability structures rather than, or in addition to, mean structures to predict health outcomes.
Research Projects:
Lead biostatistician on the Michigan cohort for the Environmental Influences on Child
Health Outcomes (ECHO), a national study of children for the National Institutes of
Health.
Worked in women's health issues, including studies designed to understand the onset of menopause and to predict and ultimately treat health problems that accompany the menopausal transition.
I collaborate with researchers at the Institute for Social Research on environmental effects on health outcomes, including factors such as work stress and built environment on health in later life.
I collaborate with researchers at the University of Michigan Transportation Research Institute on a wide variety of topics related to driving behavior and driver safety, including work on a large naturalistic driving study funded though the Michigan Institute for Data Science (MIDAS).
I collaborate with smoking cessation researchers, in particular to assess the impact of various legal restrictions on smoking behavior.
Tan, Y. V., Flannagan, C.A.C., Elliott, M.R. (2021). "Accounting for selection bias due to death in estimating the effect of wealth shock on cognition for the Health and Retirement Study," Statistics in Medicine, 40, 2613-2625.
Elliott, M.R., Zhao, Z., Muhkerjee, B., Kayna, A., Needham, B.L. (2020). "Methods to Account for Uncertainty in Latent Class Assignments when using Latent Classes as Predictors in Regression Models, with Application to Acculturation Strategy," Epidemiology, 31, 194-204.
Zhou, T., Elliott, M.R., Little, R.J.A. (2019). "Penalized Spline of Propensity Methods for Treatment Comparison," Journal of the American Statistical Association (with discussion), 114, 1-38.
Elliott, M.R., Valliant, R. (2017). Inference for Non-probability Samples. Statistical Science, 32, 249-264.
Zhou, H., Elliott, M.R., Raghunathan, T.E. (2016). A Two-Step Semiparametric Method to Accommodate Sampling Weights in Multiple Imputation. Biometrics 72, 242-252.
Jiang, B., Elliott, M.R., Sammel, M.D., Wang, N. (2015). Joint Modeling of Cross-Sectional Health Outcomes and Longitudinal Predictors via Mixtures of Means and Variances. Biometrics, 71, 487-497.
Elliott, M.R., Conlon, A.S.C., Li, Y., Kaciroti, N., Taylor, J.M.G. (2015). Surrogacy Marker Paradox Measures in Meta-Analytic Settings. Biostatistics, 16, 400-12.
Elliott, M.R., Sammel, M.D., Faul, J. (2012). Variability as a Predictor of Health in Longitudinal Studies. Statistics in Medicine, 31, 2745-2756.
Elliott, M.R., Raghunathan, T.E., Li, Y. (2010). Bayesian Inference for Causal Mediation Effects Using Principal Stratification with Dichotomous Mediators and Outcomes. Biostatistics, 11, 353-372.
Lin, J.Y., Ten Have T.R., Elliott, M.R. (2009). Nested Markov Compliance Class Model in the Presence of Time-Varying Noncompliance. Biometrics, 65, 505-513.
Elliott, M.R. (2009). Model Averaging Methods for Weight Trimming in Generalized Linear Regression Models. Journal of Official Statistics, 25, 1-20.
Elliott, M.R., Little, R.J.A. (2005). A Bayesian Approach to 2000 Census Evaluation using A.C.E. Survey Data and Demographic Analysis. Journal of the American Statistical Association, 100, 380-388.
M4124 SPHII
1415 Washington Heights
Ann Arbor, MI 48109
Email: [email protected]
Office: 734-647-5160
For media inquiries: [email protected]
Areas of Expertise: Aging, Biostatistics, Child Health, Clinical Trials, COVID-19, Health Policy, Maternal Health, Substance Use, Women’s Health