Faculty Profile
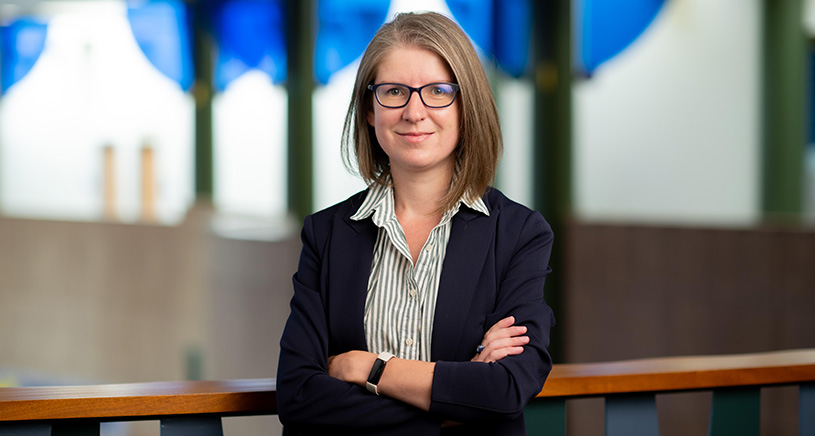
Irina Gaynanova, PhD
- Associate Professor, Biostatistics
Dr. Gaynanova’s research focuses on the development of statistical methods for analysis
of modern high-dimensional biomedical data. Her methodological interests are in data
integration, machine learning and high-dimensional statistics, motivated by challenges
arising in analyses of multi-omics data (e.g., RNASeq, metabolomics, micribiome) and
data from wearable devices (continuous glucose monitors, ambulatory blood pressure
monitors, activity trackers). Her research has been funded by the National Science
Foundation, and recognized with a David P. Byar Young Investigator Award and an NSF
CAREER Award.
- PhD, Statistics, Cornell University, 2015
- MS, Statistics, Cornell University, 2013
- Diploma (MS equivalent), Applied Math and Computer Science, Lomonosov Moscow State University, 2009
Methodological: high-dimensional data analysis (with a focus on sparsity regularization),
multivariate analysis (dimension reduction methods such as PCA, CCA, and LDA), data
integration/multi-view data analysis, statistical/machine learning, computational
statistics
Application interests: multi-omics data, microbiome data, data from wearable devices (continuous glucose monitors, ambulatory blood pressure monitors, activity trackers)
Research Projects
Application interests: multi-omics data, microbiome data, data from wearable devices (continuous glucose monitors, ambulatory blood pressure monitors, activity trackers)
Research Projects
- Developing innovative statistical methods for integration of high-dimensional disparate data sources
- Developing new machine learning methods for extracting features from digital health data
Zhang Y and Gaynanova I (2022). Joint association and classification analysis of multi-view data. Biometrics,
Vol. 78, No. 4, 1614-1625.
Broll S, Urbanek J, Buchanan D, Chun E, Muschelli J, Punjabi N and Gaynanova I (2021). Interpreting blood glucose data with R package iglu. PLoS One, Vol. 16, No. 4, e0248560.
Yoon G, Carroll R and Gaynanova I (2020). Sparse semiparametric canonical correlation analysis for data of mixed types. Biometrika, Vol. 107, No. 3, 609-625
Gaynanova I and Li G (2019). Structural learning and integrative decomposition of multi-view data. Biometrics, Vol. 75, No. 4, 1121-1132.
Yoon G, Gaynanova I and Müller C (2019). Microbial networks in SPRING - Semi-parametric rank-based correlation and partial correlation estimation for quantitative microbiome data. Frontiers in Genetics, Vol. 10, 516.
Broll S, Urbanek J, Buchanan D, Chun E, Muschelli J, Punjabi N and Gaynanova I (2021). Interpreting blood glucose data with R package iglu. PLoS One, Vol. 16, No. 4, e0248560.
Yoon G, Carroll R and Gaynanova I (2020). Sparse semiparametric canonical correlation analysis for data of mixed types. Biometrika, Vol. 107, No. 3, 609-625
Gaynanova I and Li G (2019). Structural learning and integrative decomposition of multi-view data. Biometrics, Vol. 75, No. 4, 1121-1132.
Yoon G, Gaynanova I and Müller C (2019). Microbial networks in SPRING - Semi-parametric rank-based correlation and partial correlation estimation for quantitative microbiome data. Frontiers in Genetics, Vol. 10, 516.
4602 SPH I
1415 Washington Heights
Ann Arbor, MI 48109
Email: irinagn@umich.edu
Phone: 734-746-5450
Media inquiries: sph.media@umich.edu
1415 Washington Heights
Ann Arbor, MI 48109
Email: irinagn@umich.edu
Phone: 734-746-5450
Media inquiries: sph.media@umich.edu
Areas of Expertise: Biostatistics, Diabetes, Health Informatics, Precision Health