Faculty Profile
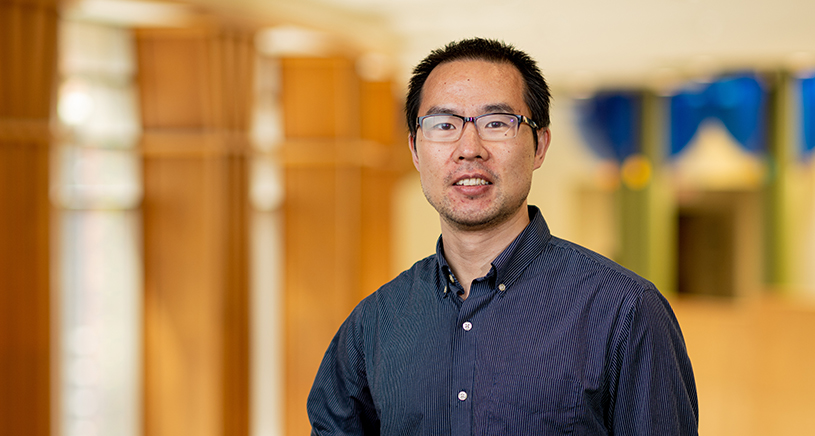
Peisong Han, PhD
- Associate Professor, Biostatistics
Dr. Han is interested in both statistical methodology development and applications. One of his primary research focuses is data integration in the presence of study population heterogeneity. He is interested in developing methods that can improve estimation efficiency and statistical power without introducing estimation bias. Another primary focus is missing data and biased sampling problems in public health studies and survey sampling. Dr. Han is also interested in interdisciplinary collaborations. Some of his collaborations include bipolar disorder research and kidney disease quality measure development, maintenance and support.
- PhD, University of Michigan, 2013
- MS, Michigan State University, 2008
- BS, University of Science and Technology of China, 2006
Research Interests:
Data integration, Missing data and biased sampling problems, Bipolar disorder research,
Kidney disease quality measure
Research Projects:
Data integration. Projects cover a variety of different data integration settings
where the study populations, models, study designs, variables collected may be different.
Missing data and biased sampling problems. Projects are to develop estimation procedures that can simultaneously accommodate multiple working models for both the missingness probability and the data distribution to reduce the impact of possible model misspecification.
Bipolar disorder research. Projects are to discover the fundamental biological changes that cause bipolar disorder and develop new interventions to treat and prevent the illness.
Kidney disease quality measure. Projects are related to developing, maintaining and updating the technical specifications for current and future quality measures for chronic kidney disease and end-stage renal disease (ESRD).
Taylor, J.M.G., Choi, K., and Han, P. (2022) Data Integration - Exploiting Ratios of Parameter Estimates from a Reduced External Model. Biometrika. To appear.
Zhai, Y., and Han, P. (2022) Data Integration with Oracle Use of External Information from Heterogeneous Populations. Journal of Computational and Graphical Statistics. To appear.
Yocum, A. K., Zhai, Y., McInnis, M. G., and Han, P. (2021) COVID-19 Pandemic and Lockdown Impacts: a Description in a Longitudinal Study of Bipolar Disorder. Journal of Affective Disorders, 282, 1226-1233
Han, P., Kong, L., Zhao, J., and Zhou, X. (2019) A General Framework for Quantile Estimation with Incomplete Data. Journal of the Royal Statistical Society - Series B. 81, 305-333.
Han, P. (2014). Multiply Robust Estimation in Regression Analysis with Missing Data. Journal of the American Statistical Association, 109, 1159-1173.
Han, P. and Wang, L. (2013). Estimation with Missing Data: Beyond Double Robustness. Biometrika, 100 (2), 417-430.
Email: [email protected]
Office: 734-615-7439
Address:
M4531 SPH II
1415 Washington Heights
Ann Arbor, MI 48109
For media inquiries: [email protected]
Areas of Expertise: Biostatistics, Brain Disorders, COVID-19, Mental Health