Faculty Profile
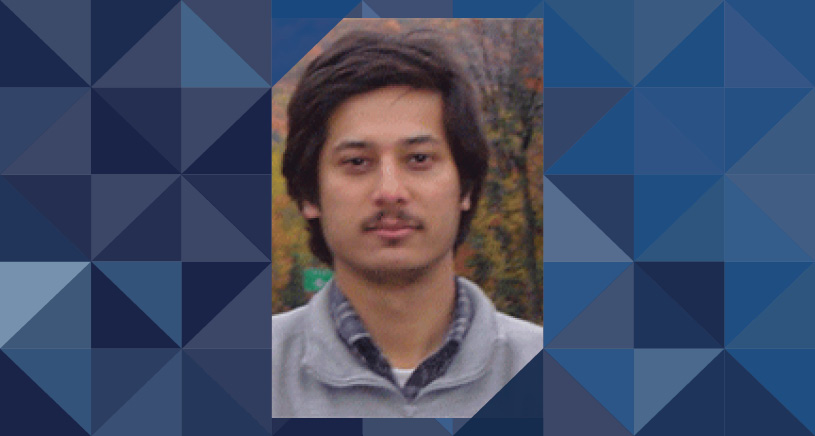
Arvind Rao, PhD
- Associate Professor, Biostatistics
- Associate Professor, Computational Medicine and Bioinformatics
- Associate Professor, Radiation Oncology
My graduate work in bioinformatics aimed to develop a framework that identifies tissue-specific enhancers by integrating multi-modal genomic (gene expression, methylation and interaction) data. As a postdoctoral fellow in Carnegie Mellon University, I obtained training in data mining methods for image analysis. Previously, at the MD Anderson Cancer Center, I developed methods to analyze the relationship between image-derived phenotypic attributes and genotypic attributes for cancer patients, focusing most of the work on datasets from the Cancer Genome Atlas (TCGA). Such image data is obtained from multiple modalities including, HandE whole slide data, immunohistochemistry, high-content microscopy, and radiology. At the University of Michigan, the primary focus of our group is to develop algorithms to quantify tumor phenotypes (from radiology, histopathology and microscopy data) and to relate those phenotypic aspects with genetic alterations like expression changes, mutation or copy number alterations. Further, we also develop methodologies (using statistical methods and machine learning) to integrate these diverse modalities (imaging, genomics etc.) to develop decision algorithms for prognosis and treatment selection. Looking forward, we are interested to develop analysis methodologies (based on spatial statistics, image analysis and machine learning) as well as tools for "spatial cancer systems biology" by leveraging spatial transcriptomics, spatial proteomics and multiplex imaging strategies of the tumr microenvironment to obtain a deeper characterization of regulatory networks underlying oncogenesis.
As part of community science initiatives, I have been a participant in Analysis Working Groups (AWGs) for the Cancer Genome Atlas (TCGA) -- I have been privileged to contribute directly or indirectly to multiple TCGA manuscripts for multiple disease sites and the Pan-Cancer efforts (through bioinformatics analyses and creating bioinformatics infrastructure for work in batch effects, data integration, immune infiltration and regulatory program analyses). This community-wide effort, we believe, has strongly improved our understanding of the cancer genome and created many new exciting tools for cancer bioinformatics research.
- PhD (University of Michigan, Ann Arbor), Electrical Engineering: Systems and Bioinformatics, 2008
Transcriptional Genomics: A bioinformatics framework that identifies tissue-specific enhancers by integrating multi-modal genomic data has been developed previously [Rao2010]. There is interest to integrate other sources of information (like epigenomic and ChIP datasets) to improve the efficacy of enhancer prediction. We have also participated in the TCGA Glioma groups' work [Brat2015, Ceccarelli2016] on identifying transcriptional regulators underlying gliomagenesis.
Image Informatics: In order to quantify the phenotypic aspects of disease and their relationships with outcome and their genetic context, we have developed methods for the analysis of histopathology [ Mousavi2015, Vu2016] and radiology [Yang2015] images, focusing on tumor heterogeneity. One direction of our group is to develop image analysis tools to delineate tumor image features from radiology data and to develop predictive models to relate them along with underlying genomic measurements to outcomes in low grade gliomas. Further, we have also investigated methodologies to link tumor imaging, genetics and immune status in gliomas. More recently, my group has been studying the relationship between image-derived features, genetics and cognitive status in glioblastoma patients. Further, we have also developed methods for the analysis of multiparametric MR datasets in Radiation Oncology.
Heterogeneous Data Integration: Integrative decision making in the clinical domain involves the need for principled formalisms that can integrate pathology, imaging and genomic data sets to drive hypothesis generation and clinical action. We have focused on developing high throughput measurement pipelines from this diverse array of data sources and methods for their integration. Simultaneously, methods for visualization are also under investigation. A more recent interest of our group is to integrate genomics, imaging and (online) behavioral data from patient to assess their evolving response to treatment, in the context of learning healthcare platforms. This could also enable the development of hybrid diagnostics.
Informatics for Combinatorial Drug Screens: the availability of multimodal data sources (cell line genomics, drug assays) coupled with high throughput, high content imaging platforms have created the need for informatics frameworks to identify rational drug combinations capable of modulating disease-associated phenotype. In this context, we have worked with the Gulf Coast Consortium to create analysis platforms that jointly mine imaging and genomics data for combinatorial drug discovery.
Kuthuru S, Dedrick W, Bai H, Su C, Vu T, Monga V, Rao A. A Visually Interpretable, Dictionary-Based Approach to Imaging-Genomic Modeling, With Low-Grade Glioma as a Case Study. Cancer informatics. Forthcoming;
Kim D, Wang N, Ravikumar V, Raghuram DR, Li J, Patel A, Wendt RE 3rd, Rao G, Rao A. Prediction of 1p/19q Codeletion in Diffuse Glioma Patients Using Pre-operative Multiparametric Magnetic Resonance Imaging. Front Comput Neurosci. 2019;13:52. doi: 10.3389/fncom.2019.00052. eCollection 2019. PubMed PMID: 31417387; PubMed Central PMCID: PMC6682685.
Kuthuru S, Szafran AT, Stossi F, Mancini MA, Rao A. Leveraging Image-Derived Phenotypic Measurements for Drug-Target Interaction Predictions. Cancer Inform. 2019;18:1176935119856595. doi: 10.1177/1176935119856595. eCollection 2019. PubMed PMID: 31217689; PubMed Central PMCID: PMC6563400.
Aung PP, Parra ER, Barua S, Sui D, Ning J, Mino B, Ledesma DA, Curry JL, Nagarajan P, Torres-Cabala CA, Efstathiou E, Hoang AG, Wong MK, Wargo JA, Lazar AJ, Rao A, Prieto VG, Wistuba I, Tetzlaff MT. B7-H3 Expression in Merkel Cell Carcinoma-Associated Endothelial Cells Correlates with Locally Aggressive Primary Tumor Features and Increased Vascular Density. Clin Cancer Res. 2019 Jun 1;25(11):3455-3467. doi: 10.1158/1078-0432.CCR-18-2355. Epub 2019 Feb 26. PubMed PMID: 30808776.
Latha K, Yan J, Yang Y, Gressot LV, Kong LY, Manyam G, Ezhilarasan R, Wang Q, Sulman EP, Eric Davis R, Huang S, Fuller GN, Rao A, Heimberger AB, Li S, Rao G. The Role of Fibrinogen-Like Protein 2 on Immunosuppression and Malignant Progression in Glioma. J Natl Cancer Inst. 2019 Mar 1;111(3):292-300. doi: 10.1093/jnci/djy107. PubMed PMID: 29947810; PubMed Central PMCID: PMC6410946.
Bharath K, Kurtek S, Rao A, Baladandayuthapani V. Radiologic image-based statistical shape analysis of brain tumours. J R Stat Soc Ser C Appl Stat. 2018 Nov;67(5):1357-1378. doi: 10.1111/rssc.12272. Epub 2018 Mar 15. PubMed PMID: 30420787; PubMed Central PMCID: PMC6225782.
Krafft SP, Rao A, Stingo F, Briere TM, Court LE, Liao Z, Martel MK. The utility of quantitative CT radiomics features for improved prediction of radiation pneumonitis. Med Phys. 2018 Nov;45(11):5317-5324. doi: 10.1002/mp.13150. Epub 2018 Sep 24. PubMed PMID: 30133809.
Moriguchi T, Hoshino T, Rao A, Yu L, Takai J, Uemura S, Ise K, Nakamura Y, Lim KC, Shimizu R, Yamamoto M, Engel JD. A Gata3 3' Distal Otic Vesicle Enhancer Directs Inner Ear-Specific Gata3 Expression. Mol Cell Biol. 2018 Nov 1;38(21). doi: 10.1128/MCB.00302-18. Print 2018 Nov 1. PubMed PMID: 30126893; PubMed Central PMCID: PMC6189460.
Kuthuru S, Deaderick W, Bai H, Su C, Vu T, Monga V, Rao A. A Visually Interpretable, Dictionary-Based Approach to Imaging-Genomic Modeling, With Low-Grade Glioma as a Case Study. Cancer Inform. 2018;17:1176935118802796. doi: 10.1177/1176935118802796. eCollection 2018. PubMed PMID: 30305794; PubMed Central PMCID: PMC6174641.
El Naqa I, Ruan D, Valdes G, Dekker A, McNutt T, Ge Y, Wu QJ, Oh JH, Thor M, Smith W, Rao A, Fuller C, Xiao Y, Manion F, Schipper M, Mayo C, Moran JM, Ten Haken R. Machine learning and modeling: Data, validation, communication challenges. Med Phys. 2018 Oct;45(10):e834-e840. doi: 10.1002/mp.
Email: [email protected]
Phone:734-647-1289
Address:
1415 Washington Heights
Ann Arbor MI 48109-2029
Areas of Expertise: Biostatistics