Faculty Profile
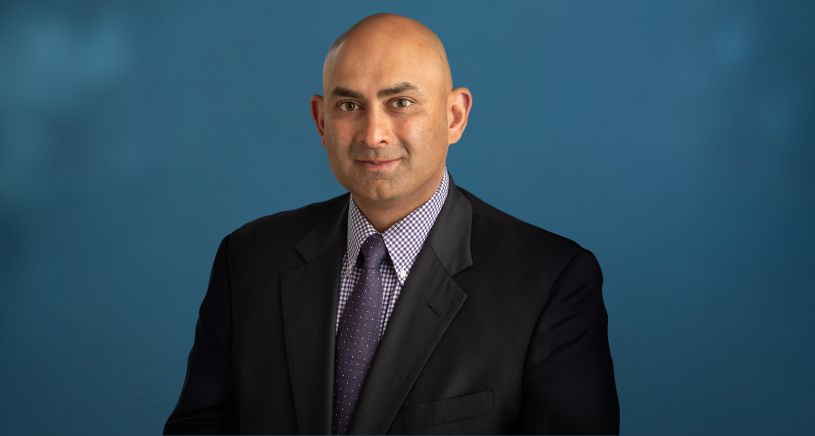
Akbar K. Waljee, MD, MS, AGAF
- Assistant Dean, Global Health Research
- Lyle C. Roll Professor, Learning Health Sciences and Internal Medicine
- Professor, Epidemiology, University of Michigan
- Research Scientist, VA HSR&D Center for Clinical Management Research
- Staff Physician, Gastroenterology VA Ann Arbor LTC Charles S. Kettles Healthcare System
- MS, University of Michigan, 2007
- MD, Emory University, 2002
- MPH, Emory University, 1998
- BS, Emory University, 1996
Dr. Waljee’s research focuses on tailoring care to individuals with gastrointestinal and liver diseases. He uses artificial intelligence and machine learning to extract knowledge from complex data to achieve better health outcomes. This impactful approach facilitates the delivery of efficient, effective, and equitable care, especially in resource-constrained settings.
Research Projects:
U54TW012089 (PIs: Amina Abubakar, Akbar Waljee) 09/15/2021 – 08/31/2026
NIH / Aga Khan University Total UM Award $1,299,985
UtiliZing health Information for Meaningful Impact in East Africa Total Award $6,489,641
Through Data Science (UZIMA-DS)
The UZIMA-DS research hub will create a data platform that will utilize artificial intelligence and machine learning methods to uncover early warning signals to address critical health issues impacting young Africans in two domains: maternal, newborn, and child health and mental health. The Hub will address three critical needs across the translational spectrum of data science: (1) Harmonization of multimodal data sources; (2) Leveraging temporal patterns of data to identify trajectories through prediction modeling using AI/ML-based methods; and (3) Engaging with key stakeholders to identify pathways for dissemination and sustainability of these models in target communities.
Role: Principal Investigator
IIR I01 HX003749-01A2 (PIs: Akbar Waljee, Jason Hou) 07/01/2024 – 06/30/2029
Department of Veterans Affairs HSR&D (Merit) Total Award $1,643,256
A Learning Health System Approach to Using Artificial Intelligence Enabled Decision Support (AEDS) for Medication Optimization in Veteran Care: An Immunosuppression Use Case
Aim 1: To adapt and validate AI/ML-based models to predict adequate immunosuppression in Veterans with IBD using real-world data from the VHA EHR (Data-to-Knowledge). Using two cohorts, we will adapt and (1) externally validate our previous model among Veterans with moderate-severe UC on thiopurine monotherapy and (2) internally validate a new model for Veterans with IBD who are on thiopurines and concomitant anti-TNF biologics (i.e., combination therapy), aligning with clinical practice guidelines. Aim 2: To assess the usability, acceptability, and feasibility of AEDS for thiopurine optimization in Veterans with IBD (Knowledge-to-Practice). Guided by the Unified Theory of User Acceptance, we will elicit behavioral, technological, and systems facilitators and barriers to successfully implementing the AEDS via semi-structured interviews with key stakeholders (e.g., providers, facility leadership, Community Veterans Engagement Board members), and findings will be fed back to key health system stakeholders. Aim 3: Evaluate the impact of an AEDS-optimized thiopurine treatment strategy on resource utilization (Practice-to-Data). Using historical VHA data, we will use reinforcement learning to evaluate resource utilization under the current care treatment strategy compared to an AEDS thiopurine optimization strategy for UC.
Role: Principal Investigator
U01CA287852 09/15/2023 – 08/31/2026 (PIs: Mansoor Saleh, Shahin Sayed, Akbar Waljee,
Ulysses Balis, Arvind Rao) Total UM Award $431,102
FIC-NIH / Aga Khan University Total award $749,997
Leveraging Artificial Intelligence/Machine Learning-Based Technology to Overcome Specialized Training And Technology Barriers for the Diagnosis and Prognostication of Colorectal Cancer in Africa
Our overall goal is to leverage our validated SIVQ/VIPR computational pipeline to develop and validate an AI-based diagnostic decision support (AI-DDS) tool for colorectal cancer (CRC) diagnosis and prognosis in an existing Kenyan cohort.
Role: Principal Investigator
Read AJ, Zhou W, Saini SD, Zhu J, Waljee AK. Prediction of gastrointestinal tract cancers using longitudinal electronic health record data. Cancers (Basel). 2023 Feb 22;15(5):1399. [PMCID: PMC10000707].
Noureldin M, Rubenstein JH, Kenney B, Waljee A. Re-evaluating early-onset OSCC in Africa: findings of minimal cumulative incidence. Gut. 2024 Feb 15:gutjnl-2023-331687 [Online ahead of print.] [PMID: 38360071]
Waljee AK, Weinheimer-Haus EM, Abubakar A, Ngugi AK, Siwo GH, Kwakye G, Singal AG, Rao A, Saini SD, Read AJ, Baker JA, Balis U, Opio CK, Zhu J, Saleh MN. Artificial intelligence and machine learning for early detection and diagnosis of colorectal cancer in sub-Saharan Africa. Gut. 2022 Jul;71(7): 1259-1265. [PMCID: PMC9177787].
Edwards DM, Pirzadeh M, Van T, Jiang R, Tate A, Schaefer G, James J, Bishop C, Wilson C, Nedzesky N, Alseri A, Leveque A, Malus A, Waljee A, Elliott DA, Deng J, Schwartz A, Schipper M, Bryant AK, Ramnath N, Green MD. Impact of lung cancer screening on stage migration and mortality among the national Veterans Health Administration population with lung cancer. Cancer. 2024 Sep 1; 130(17):2910-2917. [PMID: 38853532].
Rego RT, Kenney B, Ngugi AK, Espira L, Orwa J, Siwo GH, Sefa C, Shah J, Weinheimer-Haus E, Sophie Delius AJ, Pape UJ, Irfan FB, Abubakar A, Shah R, Wagner A, Kolars J, Boulton ML, Hofer T, Waljee AK. COVID-19 vaccination refusal trends in Kenya over 2021. Vaccine. 2023 Jan: 27;41(5):1161-1168. [PMCID: PMC9808414].
Areas of Expertise: Global Public Health, Health Care