Faculty Profile
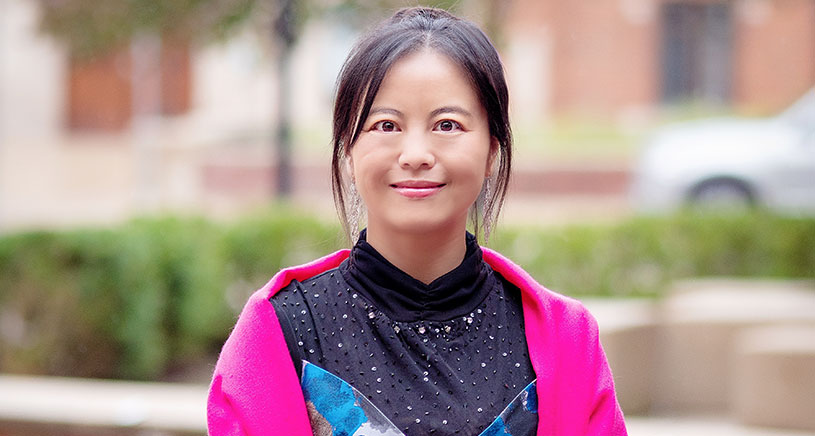
Lu Wang, PhD
- Professor, Biostatistics
Dr. Lu Wang received her Ph.D in Biostatistics from Harvard University in 2008 and joined the faculty at the University of Michigan in the same year. Her research focuses on statistical methods for evaluating dynamic treatment regimes, personalized health care, nonparametric and semiparametric regressions, missing data analysis, functional data analysis, and longitudinal (correlated/clustered) data analysis. Dr. Wang has been collaborating with investigators at M.D. Anderson Cancer Center, University of Michigan Medical School, University of Michigan School of Public Health, University of Michigan School of Kinesiology, University of Michigan Institute of Social Research, and Harvard School of Public Health. Dr. Wang is an Associate Editor of JASA and Biometrics. She also serves as the Core Co-Leader of the Integrated Health Sciences Core (IHSC), Michigan Center on Lifestage Environmental Exposures and Disease (M-LEEaD).
Professional affiliations:
Associate Editor, Biometrics
Member, American Statistical Association
Member, Eastern North American Region, International Biometric Society
Associate Editor, Journal of the American Statistical Association: Applications and
Case Studies
- PhD, Biostatistics, Harvard University, 2008
- MA, Biostatistics, Harvard University, 2007
- MS, Biostatistics, University of Michigan, 2004
- BS, Statistics, Peking University, Beijing, China, 2002
Zhou* N, Wang L. and Almirall D. (2022) Estimating Tree-based Dynamic Treatment Regimes Using Observational
Data With Restricted Treatment Sequences. Biometrics, https://doi.org/10.1111/biom.13754
Speth* K, and Wang L. (2021) Restricted Sub-Tree Learning (ReST-L) to Estimate an Optimal Dynamic Treatment
Regime Using Observational Data. Stat in Medicine, 40(26): 5796-5812.
Sun, Y., and Wang, L. (2020) Stochastic Tree Search for Estimating Optimal Dynamic Treatment Regimes.
Journal of the American Statistical Association, 116(533): 421-432.
Tao, Y., Wang, L., and Almirall, D. (2018). Tree-Based Reinforcement Learning for Estimating Optimal Dynamic Treatment Regimes. The Annals of Applied Statistics 12 (3): 1914-38
Tao, Y., and Wang, L.. (2017). Adaptive Contrast Weighted Learning for Multi-Stage Multi-Treatment Decision-Making. Biometrics 73 (1): 145-55
Shen, J., Wang, L., and Taylor JMG. (2017). Estimation of the Optimal Regime in Treatment of Prostate Cancer Recurrence From Observational Data Using Flexible Weighting Models. Biometrics, 73(2): 635-645
Wang, F., Wang, L., and Song P. (2016). Fused Lasso With the Adaptation of Parameter Ordering in Combining Multiple Studies With Repeated Measurements. Biometrics, 72(4): 1184-1193.
Wang, F., Song, P., and Wang, L. (2015). Merging Multiple Longitudinal Studies With Study-specific Missing Covariates: A Joint Estimating Function Approach. Biometrics, 71: 929-940.
Wang, L., Shen J, and Thall P. (2014). A modified Adaptive LASSO for Identifying Interactions in the Cox Model with Heredity Constraint. Statistics and Probability Letters, 93: 126-133.
Han, P. and Wang, L. (2013). Estimation with missing data: beyond double robustness. Biometrika 417-430.
Wang, F., Wang, L., and Song, P.X.K. (2012). Quadratic Inference Function Approach to Merging Longitudinal Studies: Validation Test and Joint Estimation. Biometrika 755-762.
Wang, L., Rotnitzky, A., Lin, X., Millikan, R., and Thall, P. (2012). Evaluation of Viable Dynamic Treatment Regimes in a Sequentially Randomized Trial of Advanced Prostate Cancer. Journal of the American Statistical Association (with Discussion) 493-508.
Wang, L., Rotnitzky, A., Lin, X., Millikan, R., and Thall, P. (2012). Rejoinder of Discussions on "Evaluation of Viable Dynamic Treatment Regimes in a Sequentially Randomized Trial of Advanced Prostate Cancer". Journal of the American Statistical Association 518-520.
Wang, L., Rotnitzky, A., and Lin, X. (2010). Nonparametric Regression with Missing Outcomes Using Weighted Kernel Estimating Equations. Journal of the American Statistical Association 1135-1146.
M4132 SPH II
1415 Washington Heights
Ann Arbor, MI 48109-2029
Phone: 734-647-6935
Fax: 734-763-2215
Email: [email protected]
For media inquiries: [email protected]
Areas of Expertise: Biostatistics