Faculty Profile
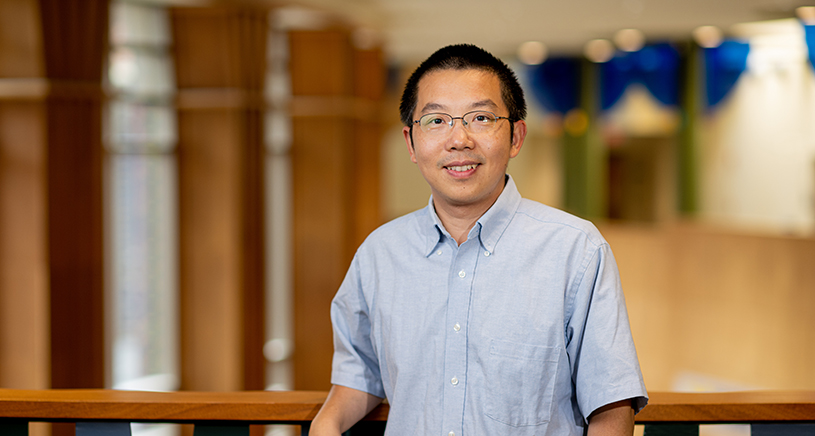
Xiang Zhou, PhD
- Professor, Biostatistics
Dr. Zhou's research is focused on developing statistical and computational methods for genetic and genomic studies. These studies often involve large-scale and high-dimensional data; examples include genome-wide association studies and various functional genomic sequencing studies such as bulk and single cell RNAseq, bisulfite sequencing, as well as spatially resolved transcriptomics studies. By developing novel analytic methods, Dr. Zhou seeks to extract important information from these data and to advance our understanding of the genetic basis of phenotypic variation for various human diseases and disease related quantitative traits.
- PhD, Duke University, 2010
- MS, Duke University, 2009
- BS, Peking University, 2004
Research Interests:
Bayesian methods, mixed effects models, spatial statistics, graphical models, integrative
modeling, non-parametric models, statistical computing, machine learning, deep learning,
health data science, and analysis of high dimensional genetic and genomic data including
genome-wide association studies, methylation studies, single cell RNA sequencing studies,
and spatial transcriptomics.
Research Projects:
Developing statistical methods and performing collaborative analyses for genome-wide
association studies (GWAS), transcriptome-wide association studies (TWAS), epigenome-wide
association studies (EWAS), expression quantitative trait loci (eQTL) mapping, methylation
quantitative trait loci (meQTL) mapping, allelic specific expression (ASE) and methylation
(ASM), and various other genetic and epigenetic studies. Methods and analyses also
include SNP heritability estimation and partitioning, controlling for population stratification,
genetic and environmental covariance estimation, causal inference with Mendelian randomization,
high dimensional mediation analysis, and polygenic risk score construction.
Developing statistical methods and performing collaborative analysis for single cell and multi-omics studies that include but not limited to differential expression (DE) analysis, gene set enrichment analysis (GSEA), batch effects removal, cell type clustering, and data integration across multiple data types and modalities.
Developing statistical methods and performing collaborative analysis for spatially resolved transcriptomics studies that include but not limited to identifying spatially variable genes with spatial expression patterns, detecting spatial domains, performing cell type deconvolution, and multi-scale and multi-sample analysis.
Ying Ma, and Xiang Zhou (2022). Spatially informed cell type deconvolution for spatial transcriptomics. Nature Biotechnology. 40: 1349-1359.
Jiaqiang Zhu, Shiquan Sun, and Xiang Zhou (2021). SPARK-X: Non-parametric modeling enables scalable and robust detection of spatial expression patterns for large spatial transcriptomic studies. Genome Biology. 22: 184.
Zhongshang Yuan, Huanhuan Zhu, Ping Zeng, Sheng Yang, Shiquan Sun, Can Yang, Jin Liu, and Xiang Zhou (2020). Testing and controlling for horizontal pleiotropy with the probabilistic Mendelian randomization in transcriptome-wide association studies. Nature Communications. 11: 3861.
Shiquan Sun, Jiaqiang Zhu, and Xiang Zhou (2020). Statistical analysis of spatial expression pattern for spatially resolved transcriptomic studies. Nature Methods. 17: 193-200.
Ping Zeng and Xiang Zhou (2017). Non-parametric genetic prediction of complex traits with latent Dirichlet process regression models. Nature Communications. 8: 456.
Xiang Zhou (2017). A unified framework for variance component estimation with summary statistics in genome-wide association studies. Annals of Applied Statistics. 11(4): 2027-2051.
View full list of publications at [https://scholar.google.com/citations?user=h4_CmPoAAAAJ&hl=en]
M4166 SPH II
1415 Washington Heights
Ann Arbor, MI 48109
Email: [email protected]
Office: 734-764-5722
For media inquiries: [email protected]
Areas of Expertise: Biostatistics, Cancer, Genetics, Health Informatics, Precision Health