Faculty Profile
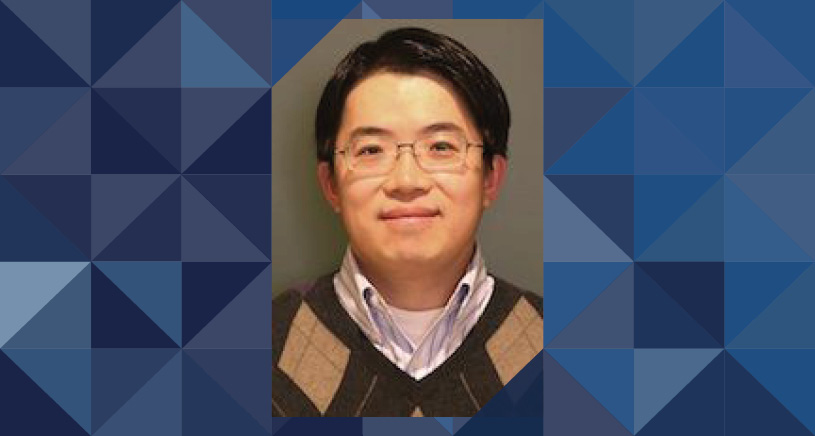
Hyun Min Kang, PhD
- Professor, Biostatistics
Dr. Kang's research focuses on developing robust, scalable, and practical methods and tools to analyze large-scale genomic data to help understand the etiology of complex traits. He received his PhD in Computer Science from University of California, San Diego in 2009 and joined the University of Michigan faculty in the same year. Prior to his doctoral studies, he worked as a research fellow at the Genome Research Center for Diabetes and Endocrine Disease in the Seoul National University Hospital for a year and a half, after completing his Bachelors and Master's degree in Electrical Engineering at Seoul National University. His research interest lies in big data genome science. Methodologically, his primary focus is on developing statistical methods and computational tools for large-scale genetic and genomic studies. Scientifically, he aims to understand the molecular basis of complex disease-related traits by leveraging cutting-edge genomic technologies including spatial transcriptomics, single-cell genomics, and whole genome sequencing.
- PhD, Computer Science, University of California, San Diego, 2009
- MS, Electrical Engineering, Seoul National University, 2000
- BS, Electrical Engineering, Seoul National University, 1998
Research Interests:
Spatial transcriptomics. Single-cell transcriptomics and epigenomics. Robust tools
for analyzing sequence data. Statistical methods for genome-wide association studies.
Research Projects:
Ultra-high resolution spatial transcriptomics: My recent research focus is to precisely
understand the mechanism of gene regulation through ultra-high-resolution spatial
transcriptomics. Dr. Jun Hee Lee and I developed SeqScope, a submicrometer resolution
spatial transcriptomics technology that repurposes Illumina sequencing platform to
profiling transcriptomes at submicrometer resolution. Using SeqScope, we are able
to understand transcriptional dynamics of individual cells and subcellular components.
I am developing software tools to leverage this ultra-high-resolution technology to
unravel the detailed mechanisms underlying complex diseases at scale.
Single cell transcriptomics and epigenomics: Over the past several years, I focused on developing methods to understand the molecular mechanism of gene regulation in single cell resolution at scale. I developed methods (demuxlet/popscle) to substantially reduce cost, time, effort, and batch effects in performing population-scale single-cell experiments. I am advancing these techniques and methods in multiple aspects to enable more scalable, accurate, and seamless single-cell profiling of transcriptomes and epigenomes across thousands of individuals.
Robust tools for analyzing sequence data: Rapid, accurate, and robust analysis of sequence reads is very important for successful genetic analysis in population scale. I developed many software tools to enable high-quality analysis of DNA sequence reads, including, but not limited to verifyBamID, verifyBamID2, GotCloud, RUTH, FastQuick, vt, cramore, cleanCall, popscle. The verifyBamID and verifyBamID2 tools are now the standards for estimating DNA contamination from the large-scale sequencing data across various genetic ancestries. GotCloud (Genomes On The Cloud) sequence processing and variant calling pipeline produces high quality variant calls from high-throughput DNA sequence reads. It has been applied to sequence data across hundreds of thousands of human genomes. I continually develop software tools to enable specific analytic tasks forDNA sequence and single-cell genomic data into cramore, RUTH, and vt software tools. My current research focus in this area lies in comprehensive and accurate characterization and visualization of short insertion and deletions, including short tandem repeats and variable nucleotide tandem repeats (VNTR) in a unified framework.
Statistical methods for genome-wide association studies: I develop various statistical methods for accurate, efficient, and robust genome-wide association studies (GWAS), capitalizing on hidden relatedness or DNA sequencing. I pioneered GWAS with a linear mixed model to account for hidden relatedness and population structure altogether using EMMA and EMMAX. Each of these papers are together cited thousands of times and motivated the development of many other association analysis tools under linear mixed models. With the advent of sequencing technologies, I implemented existing GWAS methods for large-scale sequence data into a scalable software package called EPACTS. I also developed many methods including GeneVetter, GIMS, GAMBIT, and emeraLD, for efficient analysis and comprehensive interpretation from GWAS data.
Kwong A, Boughton AP, Wang M, VandeHaar P, Boehnke M, Abecasis G, Kang HM. (2022) FIVEx: an interactive eQTL browser across public datasets. Bioinformatics. 38(2):559-561 PMCID:PMC8723151 https://www.ncbi.nlm.nih.gov/pmc/articles/PMC8723151
Cho CS, Xi J, Si Y, Park SR, Hsu JE, Kim M, Jun G, Kang HM, Lee JH. Microscopic examination of spatial transcriptome using Seq-Scope. (2021) Cell. 184(13):3559-3572.e22. PMCID:PMC8238917. https://www.ncbi.nlm.nih.gov/pmc/articles/PMC8238917
Zhang F, Flickinger M, InPSYght Psychiatric Genetics Consortium, Abecasis GR, Boehnke M, Kang HM, (2019) Ancestry-agnostic estimation of DNA sample contamination from sequence reads. Genome Res. 30(2):185-194. PMCID:PMC7050530 https://www.ncbi.nlm.nih.gov/pmc/articles/PMC7050530
Kang HM, Subramaniam M, Targ S, Nguyen M, Maliskova L, Wan E, Wong S, Byrnes L, Lanata C, Gate R, Mostafavi S, Marson A, Zaitlen NA, Criswell LA, Ye CJ (2018) Multiplexing droplet-based single cell RNA-sequencing using natural genetic barcodes, Nat Biotechnol, 36(1):89. PMID: 29227470; PMCID: PMC5784859. https://www.ncbi.nlm.nih.gov/pmc/articles/PMC5784859
1000 Genomes Project Consortium, Auton A, Brooks LD, Durbin RM, Garrison EP, Kang HM, Korbel JO, Marchini JL, McCarthy S, McVean GA, Abecasis GR, The 1000 Genomes Project Consortium (2015) A global reference for human genetic variation, Nature. 526(7571):68-74. PMID: 26432245; PMCID: PMC4750478. https://www.ncbi.nlm.nih.gov/pmc/articles/PMC4750478
Kang HM, Sul JH, Service SK, Zaitlen NA, Kong SY, Freimer NB, Sabatti C, Eskin E (2010) Variance component model to account for sample structure in genome-wide association studies, Nat Genet 42(4):348-354. PMCID: PMC3092069 https://www.ncbi.nlm.nih.gov/pmc/articles/PMC3092069
4623 SPH I
1415 Washington Heights
Ann Arbor, MI 48109-2029
Email: [email protected]
Office: 734-647-1980
Fax: 734-763-2215
For media inquiries: [email protected]
Areas of Expertise: Biostatistics, Genetics, Genomics, Health Informatics, Precision Health