Courses Taught by Jean Morrison
BIOSTAT699: Analysis of Biostatistical Investigations
- Graduate level
- Residential
- Winter term(s) for residential students;
- 4 credit hour(s) for residential students;
- Instructor(s): Jeremy Taylor, Irina Gaynanova, Jean Morrison, Philip Boonstra, (Residential);
- Prerequisites: Registration for last term of studies to complete MS or MPH
- Description: Identifying and solving design and data analysis problems using a wide range of biostatistical methods. Written and oral reports on intermediate and final results of case studies required.
- Syllabus for BIOSTAT699
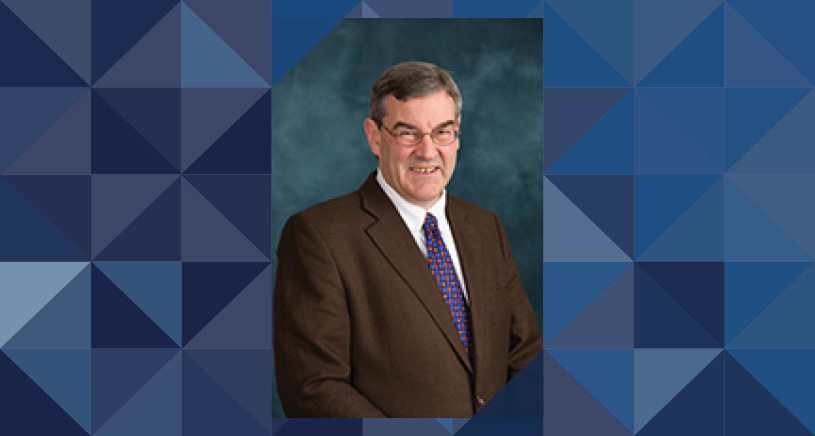
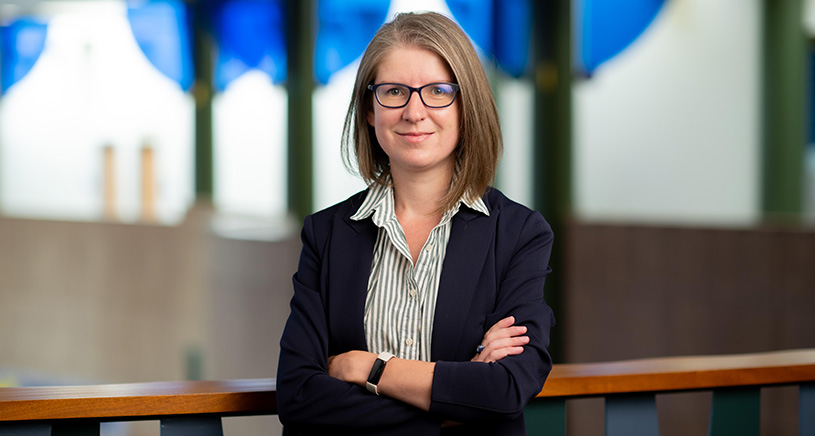
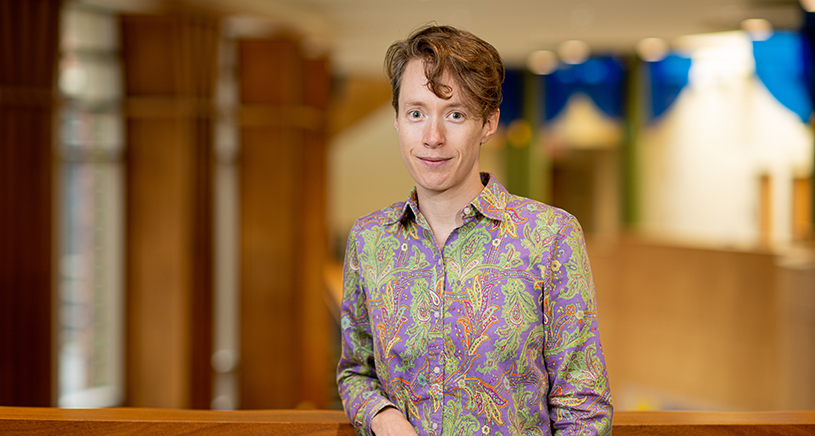
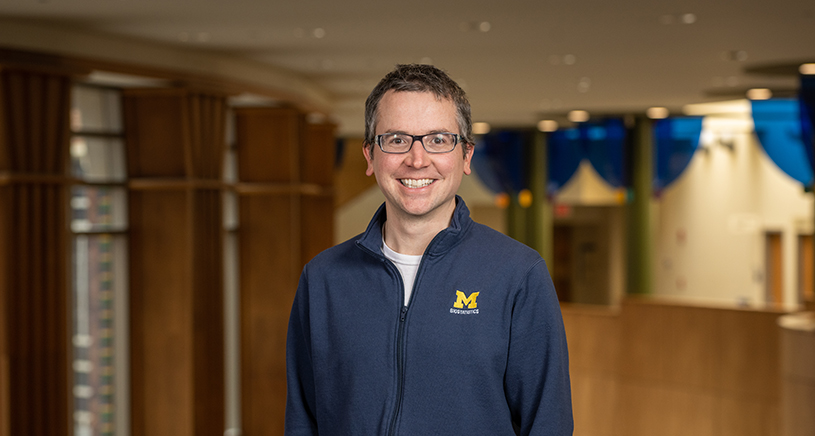
Department | Program | Degree | Competency | Specific course(s) that allow assessment | BIOSTAT | MS | Interpret the results of statistical analysis in a variety of health-related areas (e.g. public health, medicine, genetics, biology, psychology, economics, management and policy, nursing, or pharmacy) for the broad scientific community | BIOSTAT699 | BIOSTAT | MS | Communicate statistical analysis through written scientific reporting for public health, medical, and basic scientists, and/or educated lay audiences | BIOSTAT699 | BIOSTAT | PhD | Communicate through written and oral presentation based on statistical analysis for audience from a variety of health-related areas (e.g. public health, medicine, genetics, biology, psychology, nursing, or pharmacy) and for the broad scientific community | BIOSTAT699 |
---|
BIOSTAT881: Topics In Advanced Causal Inference
- Graduate level
- Residential
- Winter term(s) for residential students;
- 3 credit hour(s) for residential students;
- Instructor(s): Jean Morrison (Residential);
- Prerequisites: BIOSTAT 601, BIOSTAT 602, BIOSTAT 650, BIOSTAT 651, BIOSTAT 653, BIOSTAT 801, and BIOSTAT 802 (concurrent also accepted).
- Description: This course covers statistical theory and methodology for drawing causal conclusions from observational and experimental data. We will cover theoretical foundations including DAGs and SEMs, followed by special topics, which may include instrumental variable analysis, causal inference in high dimensions, and causal inference with longitudinal data.
- Learning Objectives: At the end of the course the students will be able to: 1. Translate a scientific question into a causal contrast to be estimated. 2. Derive graphical models for investigating the conditions under which the causal contrasts of interest are identified from data collected under specific study designs. 3. Formulate adequate structural models for making inference about the causal contrasts of interest. 4. Implement simulations appropriate for investigating the properties of causal estimators.
- Syllabus for BIOSTAT881
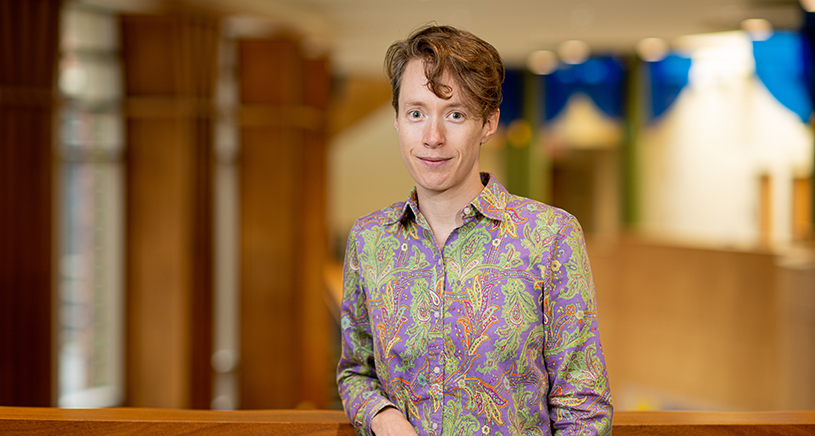