The impact of race on data
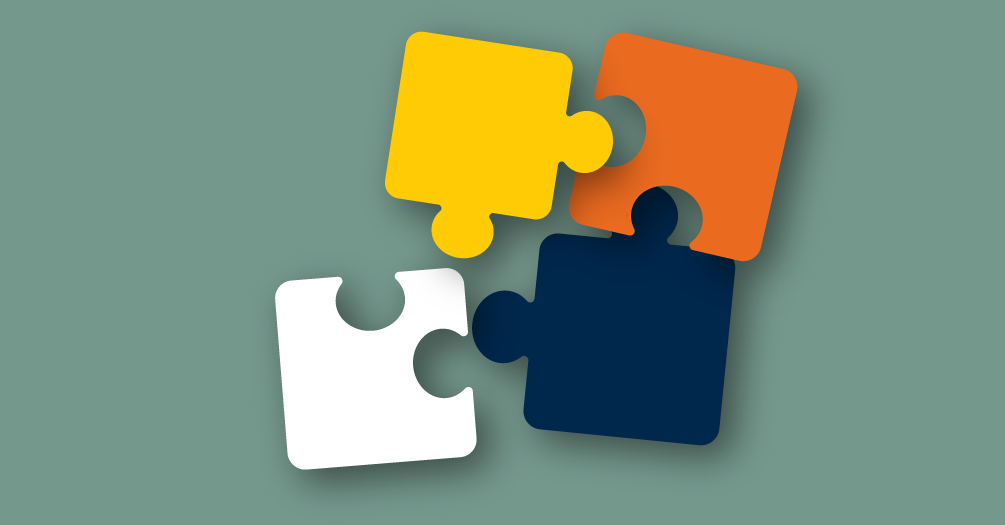
Data is a powerful, important thing. It can help researchers, practitioners, policymakers, and even frontline health workers discover new strategies that improve health, dictate things like resource and services allocation, and even save lives. But what happens when data is flawed, manipulated, or even weaponized? Can it worsen health inequities or harm populations?
In this episode of Population Healthy Season 3: Race, Inequity, and Closing the Health Gap, we talk with experts about the problematic history of racialized data in the United States, the dangers of “garbage data,” and the ways we can both gather better data, and improve collaborations with the populations we serve.
Listen to "The Impact of Race on Data" on Spreaker.
Subscribe and listen to Population Healthy on Apple Podcasts, Spotify, Google Podcasts, iHeartRadio, YouTube or wherever you listen to podcasts! Be sure to follow us at @umichsph on Twitter, Instagram, and Facebook, so you can share your perspectives on the issues we discussed, learn more from Michigan Public Health experts, and share episodes of the podcast with your friends on social media. |
[music]
00:04 Trivellore Raghunathan: Good data provides means for answering the questions, the data itself is not an answer, but I think the data is means towards creating the answers, the questions that you have. Now, good data is important, but otherwise you'll be garbage in, garbage out. So if I collect the data in a bad way, whatever inference that I draw from the data is going to be bad.
00:36 Narrator: Data is a powerful thing. It can help researchers, practitioners, policy makers, and even front-line health workers discover new strategies that improve health, dictate things like resource and services allocation and save lives, but beware of garbage data, which doesn't just disrupt the scientific process, it can be actively harmful, that's why good data is needed more than ever.
Hello and welcome to Population Healthy. A podcast produced by the University of Michigan School of Public Health. In this season of Population Healthy, we'll examine health inequities through the lens of race in America by talking to public health researchers, experts and others to learn more about what can be done to work toward health equity in our communities and across our country.
[music]
01:32 Narrator: We start this episode with Melissa Creary, an assistant professor of health management and policy at the University of Michigan School of Public Health. As the COVID-19 pandemic emerged in 2020, the pace of data collection and reporting in response to the crisis signaled a red flag for public health professionals like Creary, who understand how easy it can be for data to be misused or misinterpreted and racialized.
01:56 Melissa Creary: As we were learning more about this disease in the early days around March, April of 2020, we were really invested in trying to make sure that we knew about race-based data. And at the time, there were legislators and civic advocates and medical professionals all saying that this information was needed to ensure that African-Americans and other communities of color would have equal access to testing and treatment, and also to help develop public health strategies to protect those who are more vulnerable. And I think that when it comes to race-based data, the most important thing is that it can tell us where the burden is being felt, that can help us guide resources and messaging and money and personnel. There's this tension of the need for data specifically when it comes to race-based data, but then given our history in this country and how we have historically weaponized data, to make sure that that data is used properly, is interpreted properly, is disseminated properly, how that data is used in general. In general, we think that data is mundane, we think about age, we think about sex, we think about maybe zip code, maybe general measures around health behavior.
03:27 Creary: In terms of an individual stance, it may not seem too troubling to answer any of these questions, race-based or not, but specifically when they get attached to a racialized population, I think that there's a chance for patterns to be made, whether there's our patterns that are manipulated to tell a certain story or they're patterns to actually address inequities. And that's the weariness, I think that needs to be uplifted when we're thinking about the tension of race-based data and the ways that it can be used against a community.
[music]
04:18 Narrator: Paul Fleming is an assistant professor of Health Behavior and Health Education at the University of Michigan School of Public Health, where he focuses on health inequities. In his work, he's seen firsthand how the data we do collect and the ways in which we collect it can not only exacerbate inequalities, but harm communities and entrenched racial inequity.
04:39 Paul Fleming: The data that we have available is really based on who gets to collect data and what types of relationships they have with different communities. So sometimes people talk about having a difficult time collecting data from hard to-reach populations, but it's important that we examine that term a bit. And what does that exactly mean? Because if you're a part of a certain community that's been considered hard to reach, the community members within that aren't hard to reach to you, they're hard to reach for some of the researchers. So it's important we think about those different power dynamics about who gets to be a researcher, who gets to collect the data, and then that's really what determines who is hard to reach, and that's based on the relationships that institutions have with those communities. So over time, there's been numerous things that have caused certain communities to question researchers or questioned government agencies. The Tuskegee Experiment that was conducted by the US government that caused a lot of harm against African-American communities, that legacy has resulted in a lot of mistrust over time, along with other mistreatments by the US government of African-American populations.
05:50 Fleming: A lot of times when certain communities or certain groups are wary of reporting their own data to a government agency or to researchers, there's really good reason for that based on things that have happened in the past that have sowed distrust between the communities and the researchers. Another example of this would be with the census data. So in 1940, census data was used as part of the internment of Japanese-Americans during World War II, and so that legacy also lasts, that people think about the census data that they're reporting, that how might the government used that against them at some point in the future. The other important thing to think about, particularly when we're talking about survey data, is that necessarily survey researchers need to create categories for people to respond to, so either yes or no, or categories, for example, racial categories, but oftentimes, those categories do not reflect people's lived experience, and so sometimes respondents end up choosing a certain category that somewhat fits, but maybe doesn't fully represent who they think they are.
07:00 Fleming: We're seeing this more and more with the racial categories that the US government has. A lot of people are feeling like it doesn't actually reflect who they feel like as a person, because there's five different race categories. And of course, it's important to note that the racial categories that the US government has had in the US census has changed drastically over time. So if we look back to the 1920 census, the racial categories were white, black, mulatto, Chinese, Japanese, American Indian, Filipino, Hindu, and other. And that looks very different from today, where there's five racial categories, and then we also ask about Hispanic origin. The categories that the people who are creating the survey come up with, really dictate what your findings are gonna be. If you use those categories from 1920, then our demographic statistics are gonna look a lot different in the US right now than the categories that we're using, so it's important to consider that how the survey researchers are framing the question, how they're creating the categories, and whether or not they consulted the communities that they're researching or researching with, all determines how good a quality that data is gonna be and how representative it is of the people that are responding.
[music]
08:23 Narrator: So how do we build quality data, and what do we do about missing data, the data that Fleming explained so often goes unrecorded. For answers, we turn to Trivellore Raghunathan, a professor of biostatistics at the University of Michigan School of Public Health, whose area of research is missing data.
08:41 Raghunathan: You are studying a population, now you are to study the population, you want to create a sample as similar as population, the sample is a miniature version of the population, that's the best way to make a projection that is reliable. Really, I think if you want to create an appropriate tool for studying the population, it should be as similar to the population as possible and as unbiased way in which it is constructed, we have to have the representative sample from the population in order to make inference about the population. It might be easier for me to collect data from my friends and make a national estimate, but that's not going to work because my friend circle may be quite different than the population that we are trying to study. So in a survey word, in the biostatistics world, this is a basic principle that must be satisfied by every study. I was involved in a National Center for Health Statistics panel that was assembled to measure diversity inequalities, there were developer disparity index, so what is the way to measure the disparities that is there along the race ethnic lines or the social economic status lines and so on.
10:11 Raghunathan: Then at that point, I said, "How are these indices estimated?" And many of them are based on the questions like the doctor ever tell you that you have diabetes. So it's a survey question that is asked of people. Then the answer maybe yes or no. Now, then I thought about that, I said, the answer may be no because you may never have seen a doctor, therefore, you may say that no, not because you don't have the diabetes because you may have never seen a doctor so that you don't have an opportunity for being said yes or no. That led me to think about, is there any way that I can measure this kind of issue where people say, no, but actually they have the disease. Based on that work, I develop a method for adjusting our estimates of disparity to account for the fact that answers to the question that, did doctor ever tell you that you have a diabetes, might be no, that doesn't mean that that person doesn't have a disease, but that person never had an occasion to see the doctor so that he or she can come to know about the disease.
11:27 Raghunathan: Although the survey was a representative sample, and it was done very perfectly, but the measurement issue is there, because the way I think the question was asked that might create the inequities. That's a one example where the biostatistics can help in terms of trying to find out, are we asking the questions that is really measuring what we want to measure?
[music]
11:59 Raghunathan: Many times people ask about the solution to the problem after they have collected the data, but I think the most important thing is to think about these kinds of problems before you collect the data, so that you can make a decision about what additional data has to be collected. I'll give you an example of one of these steps that we took to make a correction for the missing data, this was a survey that was conducted many years ago, and people were asked about their income, and often people don't want to release that information or don't want to give that information.
12:38 Raghunathan: So that creates biases because we have insufficient data on income from people in the sample. That's my speciality. I find ways in which I can adjust for the missing data, so we ask interviewers to collect data from the neighborhood, what kind of housing structure was it, what kind of car people had in their driveway? Was it a two-car garage? Was it a no garage? What kind of a neighborhood was that? Was it a side walks in the neighborhood? So these are additional information that you can try to collect through the interviewer observations that might provide some way of predicting what the income is, so that's what we call imputation. So you kind of impute the missing values. The missing data can introduce biases because some people are giving information, some people are not giving information, and especially the people who are giving information might be quite different from the people who are not willing to give information. So those biases has to be accounted for, but in order to account for, you need additional variables, so you need to think in two theory and think kind of normal ways in which you want to collect that information beforehand so that you can have the necessary information to make this adjustment.
14:09 Narrator: Eliminating the biases from data is so important because at its core, data paints the picture of a community, what its needs are, what ailments they're affected by, their habits, if we don't understand those questions, we are limited in the ways we can help them effectively make meaningful change.
14:26 Creary: Anyone who's interested in public health has to understand the need for data and has to understand the good that can come from data. The data is such a huge piece of trying to paint a story, and there are many ways to create this narrative for certain populations, for certain diseases. So I think data collection on its own absolutely has merit. In fact, race-based data collection has an extreme amount of merit as well. The studies that were created, the scales and measures that we created, I'm thinking specifically by our own pioneers at University of Michigan, like James Jackson who created a scale specifically about the experiences of black lives as it related to health, these kinds of things were crucial in the development of eventual programs, policies, interventions. In 1985, we have the first federal turn to be thinking about Minority Health with the Heckler report, that was the beginning of, I think, some intentional call to pay attention to the ways that difference plays itself out, and how those differences in lived experiences lead to differences in health outcomes for different populations.
15:57 Creary: So here we are many years later, and this calling out of racism as a public health issue means that that naming gets to be paired with accountability and some sort of data, so we can call it out and now I can collect data around it, and so now maybe we can get an idea of what racism looks like when we're talking about a public health issue, then we can begin to use this data about race and racism to then figure out a public health solution to racism. The data collection piece is important. I do think that we should be careful how we collect that data, that we have to be sure that we include the communities that are involved in very thoughtful ways that we are not paternalistic when it comes to the data collection, that we center the voice of the community as we're developing and designing the research so that they are part of the data collection process. There are ways that I think we can be more responsible, but I think that we should always be attentive to the importance of data collection.
0:17:10 Creary: And then we have interpretation, and I do think that while we should not let data collection off the hook, that there is, I think more danger in the interpretation of said data, making sure that once it's collected, it's then presented in a way that if we're talking about its utility towards equity, that it's presented in a way that actually helps us gain strongholds towards actually getting that equity. I have read studies where the participants have noted quite sadly, how their black bodies are needed to give certain power to research projects, but the treatment that they receive in the healthcare system don't necessarily match up with the amount of attention that is often given as a result of wanting to make sure that there are enough of different populations in a research study. And people who are part of research projects, they know this, they understand that the data they provide as a black or brown person is important data, but they also understand this legacy that the Tuskegee experiment really wove throughout a conversation that has not really come to completion.
18:43 Creary: Even if we go to 2020, and we think about the vaccine for COVID-19, and we think about the conversations that are already being held within communities of color of this resistance to the vaccine. Again, I think as a result of it being connected to the federal government, we can see how these patterns are playing themselves out over and over again from this incident that happened in the 1930s and the legacy efforts to now in 2020.
19:15 Narrator: Better data starts with new innovative ways to collect it. With community buy-in, that data can turn into meaningful action and activism.
19:24 Fleming: So when we think about research, it's important to consider that there's a lot of different ways to collect data, there's survey research. There's other types of quantitative research, there's qualitative focus groups or interviews, and there's a lot of other ways that we can collect data and information from people. Ultimately, the best way to understand something or answer a question is to look at it from multiple angles, so survey data can certainly give us a lot of important information, and if it's done well, can give us a sense of the prevalence of a certain issue or demographic characteristics of a certain population, but if we wanna dig in more to lived experiences or get into some of the nuances, or in other words, the ways in which maybe survey categories don't exactly fit people's lives, qualitative data can be really important for that. Really good qualitative research does look at things from multiple angles, and it's important that we consider that and don't use any one single data point or one single source of information to feel like we completely understand the story on something.
20:30 Fleming: Data can be a really important piece of the puzzle in terms of policy changes that are gonna improve health for communities and activism around what changes are needed. So sometimes communities themselves lead the efforts to collect the necessary data to advocate for a certain change, sometimes they partner with researchers or sometimes government agencies themselves collect data to better understand a problem so that they can fix some of the internal policies or fix some of the laws. Data can be a really important tool when we're talking about social change, and because it's so important for how our policies and high level decisions get made, it can also be manipulated, which is why we all need to take a critical lens through the data that we're seeing, think about how it was collected, who it's representing and what type of biases may exist within that data.
[music]
21:26 Fleming: Some researchers like to think once they collect the data and publish it in an academic journal, then change may magically happen, but in reality, there's a substantial effort that needs to happen between the data collection and policy changes, and that really involves communication with key decision makers and using that data to inform community members, inform the building of coalitions and inform groups that are gonna be working collectively in support of a certain change of a certain policy. In public health, we see examples where people collect data on air quality, for example, and then report it to their local government and that can lead to changes in laws related to pollution or laws related to what types of businesses can operate within a certain jurisdiction. If we think about the Flint water crisis, that's another really important example where community activists, locals were ringing the alarm bells about the water situation, and then ultimately we're able to partner with some researchers who are able to collect data on the harms that the water contained and that activism combined with data led to substantial changes and greater awareness in what was happening and greater response, but that didn't happen on its own, right, there wasn't just a collection of data, and then change magically happened.
22:48 Fleming: It takes people building relationships with politicians, it takes people knocking on doors to share with other community members about the information they've learned and mobilizing folks to demand certain changes. So this is all part of an advocacy-based or activism-based public health. Taking the data that we collect or the things that we know and supporting the local initiative, supporting the grassroots efforts and the connections to policy makers to make sure that the policies we have in our communities are evidence-based, they're informed by the data that we are collecting. And that's really how to make change in our society and in our communities. So if we think about how to do data collection more equitably, the really key thing is that we think about trust and prioritize that from the very, very start. We should be building trusting relationships with communities that we're seeking to research with, that trust should be built based on the fact that we are creating research questions together and in partnership, making sure that any concerns are being addressed quickly, and that again, it's an equitable partnership that the research is happening within, not just a researcher coming into a community and trying to extract data.
24:08 Fleming: Some of the research I do is with undocumented immigrants, and so we very much know that the partnership is essential to do this work, so we partner with key organizations that are trusted by undocumented community members and have undocumented community members as part of their leadership or part of their group. We also make sure to recognize that they may be concerned if we're asking questions related to citizenship status, they may be concerned if we're asking them certain questions that feel too personal, if we make sure that we're thinking about that trusting relationship, and that we don't wanna be asking any questions that's gonna make them feel uncomfortable, then we really shape our research around that. The other piece of that is making sure that we're taking all of the measures we can to protect that data, so even if people are willing to tell us sensitive information that that information will not get shared, what we want is the best, highest quality data that we can get. And really the true way to do that is by forming equitable partnerships with communities and building that trust at the outset.
25:16 Narrator: Data is extremely valuable when created and deployed with care. With community voices and community investment, Public health can give so much back.
25:26 Creary: I have found that there are a lot of communities and community partners that would benefit from being directly involved in the collection of data about their own communities, but don't really understand that research can serve as a tool for empowerment for their communities, that there is a way being involved with research can be profitable for community-based organizations, when it comes to a partnership with institutes that are invested in investing in communities, and that's not just investing of their time, but it's an investment of resources and it's coming together so that it's not being spent in irresponsible ways, but it's being spent towards a shared goal of collecting data that will then be translated to go back into the community. So what that means is that we have to take the time that's necessary to invest in communities, which I think as researchers, we often don't have enough time.
26:34 Creary: If you get a grant, that grant is supposed to be deployed in two to three years, when in actuality, it should take a year or two years to actually be embedded in the community to do the kind of work that you would need in order to get the best data at the end of the research project. The time that's necessary is something that I wish could be better built into the design and timeline process of research, because I think the more time that we invest upfront, it means that we are able to obtain that accurate, reliable and useful data on the back end. The reality is that the academy and the research apparatus just isn't infused with enough communities of color for it to be a reality for us to say that all of the research that's done in communities of color needs to be led by practitioners or researchers who are also of color or who have that same identity match. I think that that is something that we should be aspiring to when we think about racism as a public health crisis, it's not just that COVID has shown us that there are disproportionate health outcomes in black populations.
27:54 Creary: I think racism as a public health issue means that we attend the same anti-racist strategies towards populations that we're studying to the researcher community that those anti-racist practices of making sure some of the structural and systemic barriers that keep researchers and physicians and doctoral students out of this research apparatus that that's something that needs just as much attention. And I think it's unrealistic to say there has to be this one-to-one identity match, but I also think that that's why it's so important to invest in the time to include the voices that you are studying as part of your research team, so even if that means that the PI, and the PI is a principal investigator, the person that is the lead manager of the research project, even if that means that the PI isn't necessarily matched by race and culture and ethnicity, I think that's perfectly fine. I think that the research team needs to be reflective of the community, and that the research team should include the community members themselves.
[music]
29:23 Narrator: On the next edition of Population Healthy.
29:25 Speaker: I first began to think about what I would come to call weathering when I was in college in the mid-1970s. I worked part-time at a school and clinic for pregnant teenagers in Trenton, New Jersey, which was a working class city with a large population of color, and I noticed that the teenagers in Trenton suffered from chronic health conditions that were unheard of in my generally better off and less diverse group of Princeton classmates of about the same age. But no one was talking about this.
[music]
30:00 Narrator: Thanks for listening to this episode of Population Healthy: Race, Inequity and Closing the Health Gap from the University of Michigan School of Public Health. I hope you learn something that will help you make the world a healthier place. Please subscribe or follow our podcast on Apple Podcast, Google Play, Stitcher, Spotify, or wherever you listen to podcasts. Interested in studying public health with us? Join our interest list by going to our homepage, publichealth.umich.edu and check out our programs and degrees and other helpful resources across our website.
30:29 Narrator: Be sure to follow us @umichsph on Twitter, Instagram and Facebook. To join the conversation, learn more from Michigan public health experts and share episodes of the podcast with your friends and followers. You can also check out the show notes on our website, publichealth.umich.edu/podcast for more resources about the topics discussed in this episode. If you wanna stay up date with the latest research and expertise from Michigan Public Health, subscribe to our weekly newsletter, Population Healthy. Head to publichealth.umich.edu/news/newsletter to sign up and be sure to join us next time. Thanks for listening and doing your part to make the world a healthier place for all.
In This Episode
Melissa S. Creary, PhD, MPH
Assistant Professor, Department of Health Management and Policy
Senior Director, Office of Public Health Initiatives, American Thrombosis & Hemostasis
Network
Dr. Creary’s research and teaching interests lie at the intersection of public health, science and technology studies, and medical anthropology. As Senior Director, Office of Public Health Initiatives, at the American Thrombosis & Hemostasis Network (ATHN), Melissa is responsible for supporting efforts to consolidate federal programs and strengthen partnerships, establish a Health Equity Program, and leverage ATHN’s capabilities to support medically underserved populations. Learn more.
Paul J. Fleming, PhD, MPH
Assistant Professor, Department of Health Behavior & Health Education
Paul Fleming is an Assistant Professor in Health Behavior and Health Education at the University of Michigan School of Public Health. Currently, his mixed-methods research focuses on the root causes of health inequities, with a particular focus on developing and evaluating interventions in poor and marginalized communities in Michigan and abroad. Learn more.
Trivellore Eachambadi Raghunathan, PhD
Professor, Department of Biostatistics
Research Professor, Survey Research Center, Institute for Social Research
Dr. Raghunathan’s research interests are in the analysis of incomplete data, multiple imputation, Bayesian methods, design and analysis of sample surveys, small area estimation, confidentiality and disclosure limitation, longitudinal data analysis and statistical methods for epidemiology. Learn more.