Biostatistics Courses
BIOSTAT501: Introduction to Biostatistics
- Graduate level
- Online MPH only
- This is a second year course for Online students
- Fall term(s) for online MPH students;
- 2 credit hour(s) for online MPH students;
- Instructor(s): Myra Kim (Online MPH);
- Prerequisites: SPH MPH or permission of instructor
- Description: Statistical methods and principles necessary for understanding and interpreting data used in public health and policy evaluation and formation. Topics include descriptive statistics, graphical data summary, sampling, statistical comparison of groups, correlation, and regression. Students will learn via lecture, group discussions, critical reading of published research, and analysis of data.
- This course is required for the school-wide core curriculum
- Residential Syllabus for BIOSTAT501
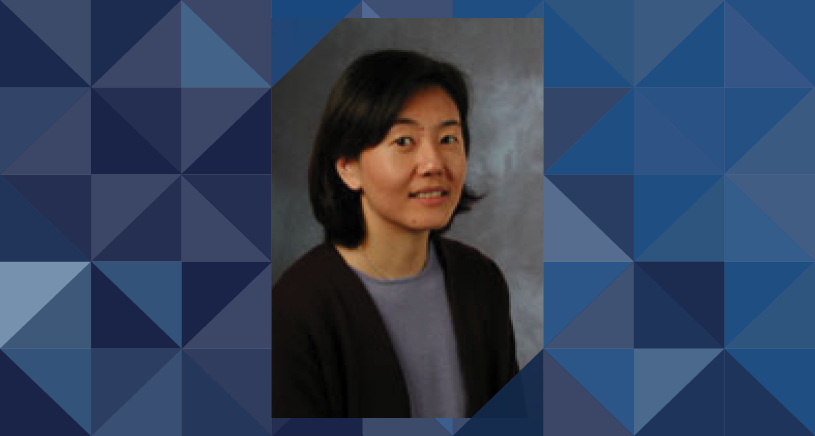
Department | Program | Degree | Competency | Specific course(s) that allow assessment | Population and Health Sciences | MPH | Compare population health indicators across subpopulations, time, and data sources | PUBHLTH515, BIOSTAT592, EPID590, EPID592, EPID643, BIOSTAT595, BIOSTAT501 | Population and Health Sciences | MPH | Estimate population health indicators from high quality data resources from diverse sources | PUBHLTH515, EPID643, NUTR590, BIOSTAT592, BIOSTAT501 |
---|
BIOSTAT512: Analyzing Longitudinal and Clustered Data Using Statistical Software
- Graduate level
- Residential
- Winter term(s) for residential students;
- 3 credit hour(s) for residential students;
- Instructor(s): Missy Plegue, Giselle Elise Kolenic, (Residential);
- Prerequisites: BIOSTAT 501 or 521
- Description: Longitudinal data sets occur often in a Public Health setting. This course will introduce students to methods for analyzing both clustered and longitudinal data using the statistical software packages SAS and Stata. Models for both continuous and discrete (e.g., binary, count) outcomes will be discussed and illustrated. The course will have one session of lecture and one session of lab per week. The course will be driven primarily by using both software packages to analyze real data sets.
- Syllabus for BIOSTAT512
BIOSTAT521: Applied Biostatistics
- Graduate level
- Residential
- Fall term(s) for residential students;
- 3 credit hour(s) for residential students;
- Instructor(s): Xiang Zhou (Residential);
- Prerequisites: Calculus
- Undergraduates are allowed to enroll in this course.
- Description: Biostatistical analysis provides the means to identify and verify patterns in this data and to interpret the findings in a public health context. In this course, students will learn the basic steps in analyzing public health data, from initial study design to exploratory data analysis to inferential statistics. Specifically, we will cover descriptive statistics and graphical representations of univariate and multivariate data, hypothesis testing, confidence intervals, t-tests, analysis of contingency tables, and simple and multiple linear regression.
- This course is required for the school-wide core curriculum
- Syllabus for BIOSTAT521
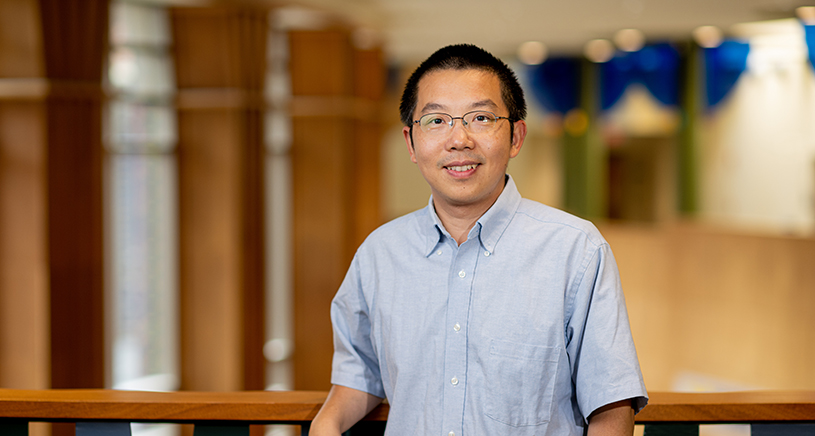
Department | Program | Degree | Competency | Specific course(s) that allow assessment | NUTR | MS | Analyze quantitative data using biostatistics, informatics, computer-based programming and software, as appropriate | BIOSTAT521 |
---|
BIOSTAT522: Biostatistical Analysis For Health-related Studies
- Graduate level
- Residential
- Winter term(s) for residential students;
- 3 credit hour(s) for residential students;
- Instructor(s): Gen Li (Residential);
- Prerequisites: BIOSTAT521; PUBHLTH 500
- Advisory Prerequisites: Calculus; R programming
- Description: A second course in applied biostatistical methods and data analysis. Concepts of data analysis and experimental design for health-related studies. Emphasis on categorical data analysis, multiple regression, analysis of variance and covariance.
- Learning Objectives: not applicable
- Syllabus for BIOSTAT522
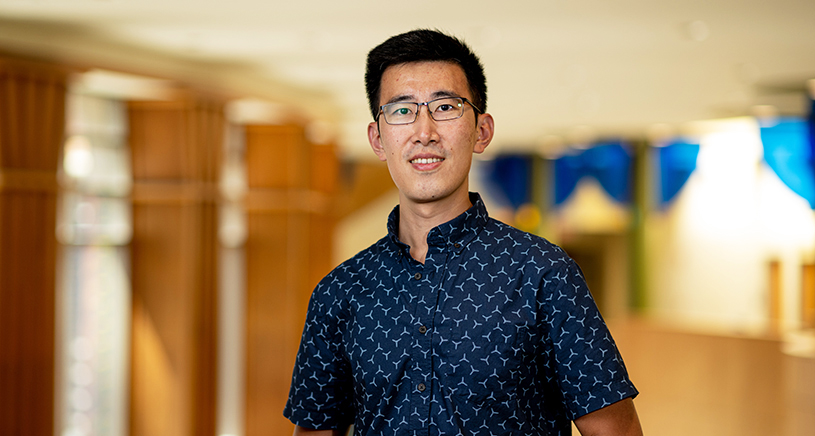
Department | Program | Degree | Competency | Specific course(s) that allow assessment | EPID | Clinical Research-Epidemiology | MS | Analyze research data and interpret these results from a population health or clinical-translational perspective | EPID602, BIOSTAT522 |
---|
BIOSTAT523: Statistical Methods in Epidemiology
- Graduate level
- Residential
- Fall term(s) for residential students;
- 4 credit hour(s) for residential students;
- Instructor(s): Staff (Residential);
- Prerequisites: EPID501 or EPID601 or EPID600; AND BIOSTAT522
- Description: Statistical methods commonly used in environmental epidemiology. Emphasis on choosing appropriate statistical methods and subsequent interpretation. Topics include probability, measures of association and risk, sample size calculations, SMR and PMR analysis, logistic regression and survival analysis.
- Syllabus for BIOSTAT523
BIOSTAT591: Introduction to R
- Graduate level
- Online MPH only
- This is a first year course for Online students
- Spring-Summer term(s) for online MPH students;
- 2 credit hour(s) for online MPH students;
- Instructor(s): Philip Boonstra (Online MPH);
- Prerequisites: None
- Description: This is a two-credit hour course preparing students enrolled in the online MPH and MS programs -- Biostatistics concentration to be 'data-ready' using the R statistical environment.
- Learning Objectives: Understanding the need to plot data Matching graphical techniques and data type Creating your set of 'go-to' graphical tools Understand the limitations of 'point and click' Incorporating the tidyverse into R Turning your "data" into data Learning R's capabilities Asking the right questions in R Writing reproducible R code Writing shareable R code CEPH learning objectives 1. Select quantitative and qualitative data collection methods appropriate for a given public health context 2. Analyze quantitative and qualitative data using biostatistics, informatics, computer-based programming and software, as appropriate 3. Interpret results of data analysis for public health research, policy or practice
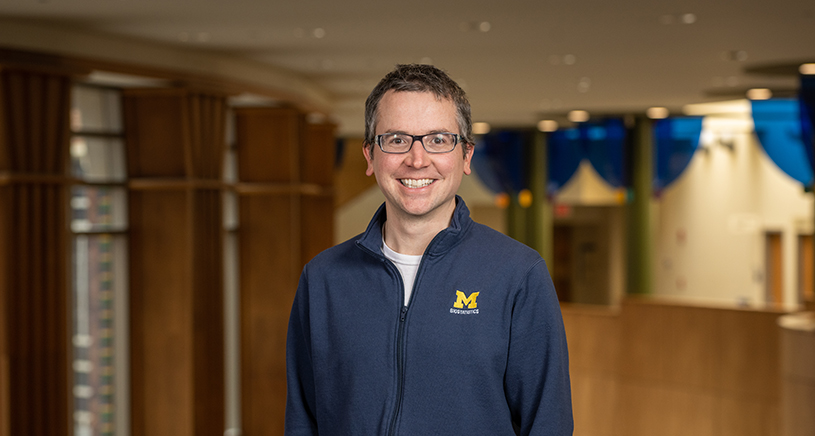
BIOSTAT592: Applied Regression
- Graduate level
- Online MPH only
- This is a first year course for Online students
- Spring-Summer term(s) for online MPH students;
- 3 credit hour(s) for online MPH students;
- Instructor(s): Kelley Kidwell (Online MPH);
- Prerequisites: BIOSTAT 501, BIOSTAT 591
- Advisory Prerequisites: None
- Description: This course is designed to introduce linear regression using multiple variables to predict a continuous outcome. This course emphasizes the application of multiple linear regression to substantive public health problems focusing on interpretation and inference. We use RStudio to analyze public health datasets, evaluate regression assumptions, and assess model fit.
- Learning Objectives: 1. Explain the critical importance of evidence in advancing public health knowledge 2. Interpret results of data analysis for public health research, policy or practice
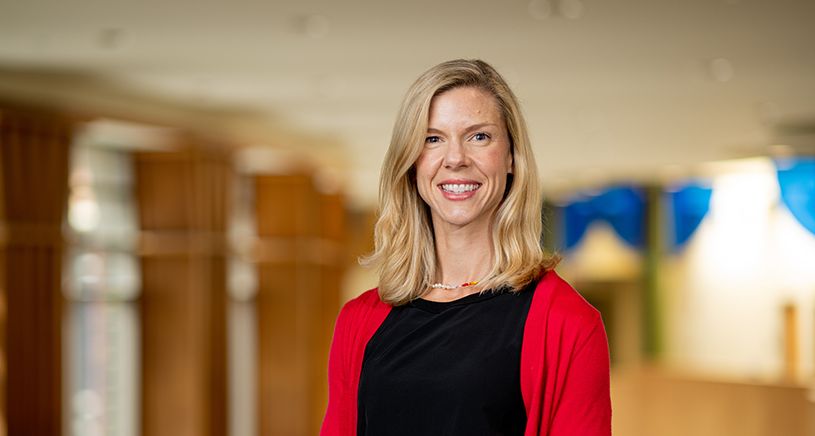
Department | Program | Degree | Competency | Specific course(s) that allow assessment | Population and Health Sciences | MPH | Compare population health indicators across subpopulations, time, and data sources | PUBHLTH515, BIOSTAT592, EPID590, EPID592, EPID643, BIOSTAT595, BIOSTAT501 | Population and Health Sciences | MPH | Estimate population health indicators from high quality data resources from diverse sources | PUBHLTH515, EPID643, NUTR590, BIOSTAT592, BIOSTAT501 |
---|
BIOSTAT593: Design for Health Studies
- Graduate level
- Online MPH only
- This is a first year course for Online students
- Spring-Summer term(s) for online MPH students;
- 1 credit hour(s) for online MPH students;
- Instructor(s): Roderick Little (Online MPH);
- Prerequisites: Biostat 501 and Pubhlth 512
- Description: Many courses in Biostatistics focus on how to analyze data, with little attention being paid to where the data came from and how it was collected. This course focuses on the design of health investigations, with particular attention to the role of randomization in the selection of units and the allocation of treatments. The first part will focus on probability sampling designs and alternatives for the selection of units from a population. The second part concerns study designs for comparing treatments or assessing potential risk factors for health outcomes. These designs include randomized clinical trials, prospective and retrospective observational studies, and clinical data bases. Key concepts include accuracy and precision of estimates, the definition of causal effects, internal validity and the role of measured and unmeasured confounders, and external validity and the role of effect modification on the generalizability of study findings. Examples of randomized and nonrandomized studies will be included to illustrate concepts. Students will be assigned readings and asked to assess design strengths and weaknesses. Quizzes will be assigned to assess knowledge of the key concepts.
- Learning Objectives: (a) Learn key features of probability sample designs -- random sampling, stratification, clustering, multistage sampling. Understand potential limitations of purposive sampling designs, and techniques to reduce the potential bias from such designs (b) Review the main study designs for the comparison of treatments and potential risk factors for a health outcome, including randomized clinical trials, prospective and retrospective longitudinal studies, case-control studies, analyses of clinical data bases. Understand the strengths and weaknesses of these alternative designs. (c) Understand how the interpretation of statistical inferences is affected by the choice of study designs.
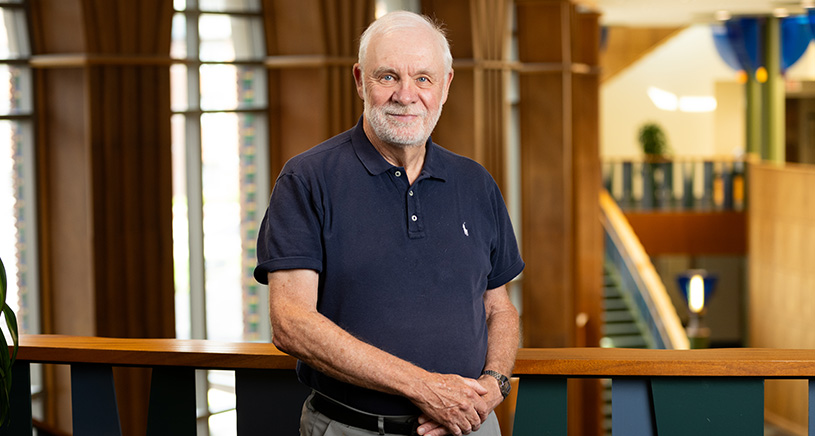
BIOSTAT594: Applied Generalized Linear Models
- Graduate level
- Online MPH only
- This is a second year course for Online students
- Fall term(s) for online MPH students;
- 3 credit hour(s) for online MPH students;
- Instructor(s): Trivellore Raghunathan (Online MPH);
- Prerequisites: BIOSTAT501, BIOSTAT591, BIOSTAT592
- Description: This course introduces public health Master's students to generalized linear models to analyze binary, discrete, ordinal, count, survival outcomes.The primary emphasis will be interpretation, inference and hands-on data analyses. We will use R to analyze public health datasets, evaluate regression assumptions, and assess model fit.
- Learning Objectives: 1. Understand the context where non-continuous outcome data are generated, identify the most relevant aspects of these data that require modeling and formulate a scientific question in terms of one or a few model parameters 2. To develop the ability to use R to analyze public health data using GLM 3. Interpret results of data analysis for public health research, policy or practice
- This course is cross-listed with .
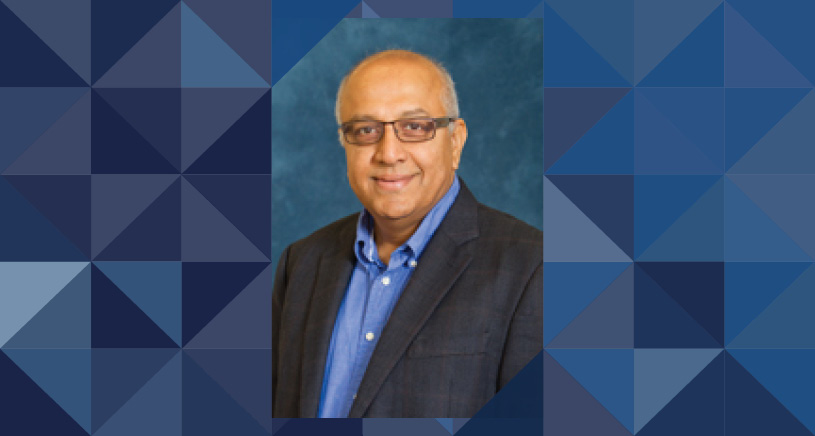
BIOSTAT595: Applied Longitudinal Analysis Using R
- Graduate level
- Online MPH only
- This is a first year course for Online students
- Fall term(s) for online MPH students;
- 2 credit hour(s) for online MPH students;
- Instructor(s): Matt Zawistowski (Online MPH);
- Prerequisites: Biostat 501, Biostat 591, Biostat 592
- Description: This course provides an overview of statistical methods for analyzing correlated data produced by longitudinal measurements taken over time. Topics include study design, exploratory data analysis techniques and linear mixed effects regression models. This course provides practical concepts and hands-on R computing skills to perform longitudinal data analysis.
- Learning Objectives: 1. Identify causes and patterns of correlated outcomes in health data 2. Perform exploratory data analysis of longitudinal outcomes 3. Fit linear mixed effects regression models 4. Interpret and perform hypothesis testing of regression parameters for mixed models
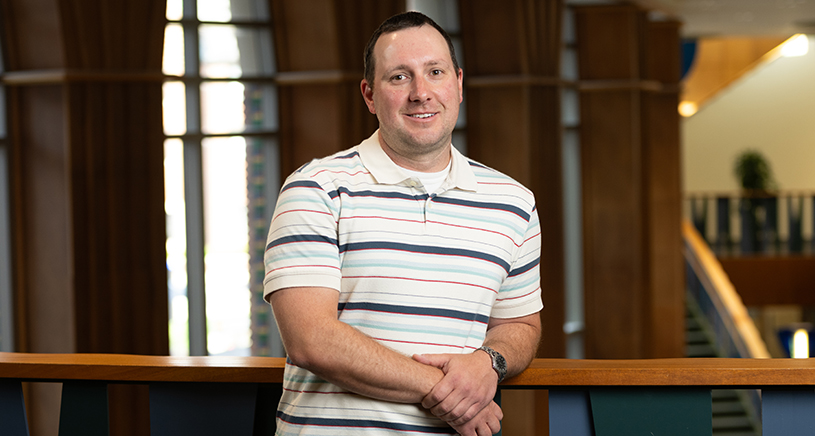
Department | Program | Degree | Competency | Specific course(s) that allow assessment | Population and Health Sciences | MPH | Compare population health indicators across subpopulations, time, and data sources | PUBHLTH515, BIOSTAT592, EPID590, EPID592, EPID643, BIOSTAT595, BIOSTAT501 |
---|
BIOSTAT600: Introduction to Biostatistics
- Graduate level
- Residential
- Fall term(s) for residential students;
- 2 credit hour(s) for residential students;
- Instructor(s): Hui Jiang (Residential);
- Prerequisites: Biostatistics students only
- Description: This course is planned as a refresher course in mathematical underpinnings that are key to statistics, like calculus, probability and linear algebra followed by discussion of basic statistical methods and concepts for all entering Biostatistics masters (and some doctoral) students. Its purpose is to prepare students for subsequent biostatistical courses.
- Syllabus for BIOSTAT600
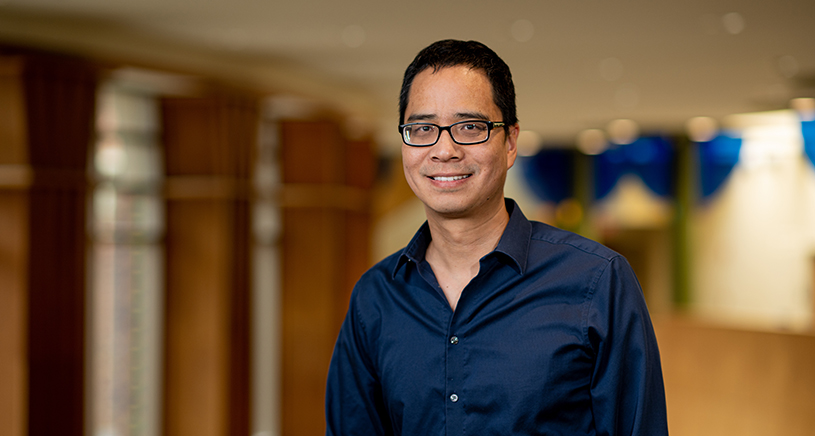
BIOSTAT601: Probability and Distribution Theory
- Graduate level
- Residential
- Fall term(s) for residential students;
- 4 credit hour(s) for residential students;
- Instructor(s): Gen Li, Thomas Braun, (Residential);
- Prerequisites: Three terms of calculus
- Description: Fundamental probability and distribution theory needed for statistical inference. Probability, discrete and continuous distributions, expectation, generating functions, limit theorems, transformations, sampling theory.
- Syllabus for BIOSTAT601
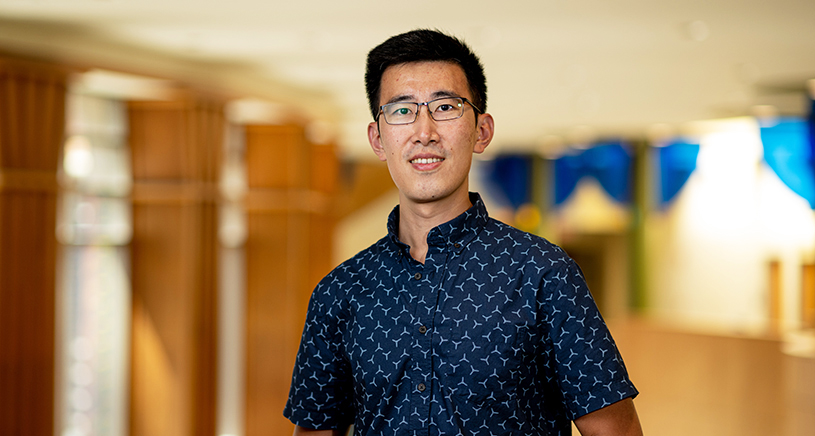
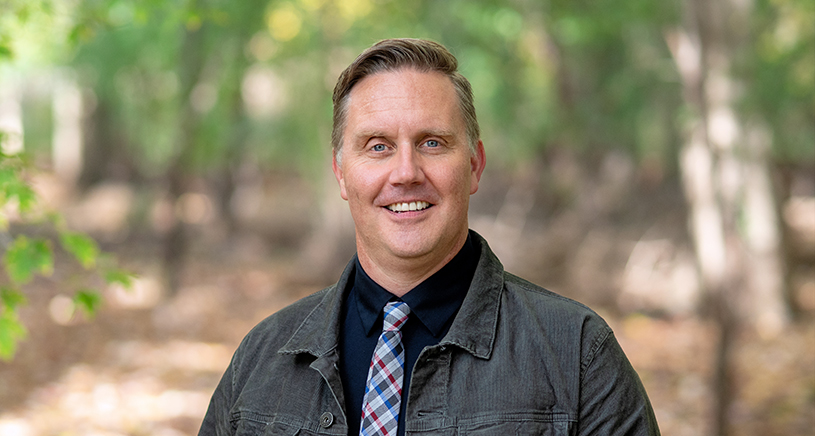
Department | Program | Degree | Competency | Specific course(s) that allow assessment | BIOSTAT | MS | Apply the theoretical foundations of probability theory and distribution theory | BIOSTAT601 |
---|
BIOSTAT602: Biostatistical Inference
- Graduate level
- Residential
- Winter term(s) for residential students;
- 4 credit hour(s) for residential students;
- Instructor(s): Xu Shi, Fan Bu, (Residential);
- Prerequisites: Biostat 601
- Description: Fundamental theory that is the basis of inferential statistical procedures. Point and interval estimation, sufficient statistics, hypothesis testing, maximum likelihood estimates, confidence intervals, criteria for estimators, methods of constructing test and estimation procedures.
- Syllabus for BIOSTAT602
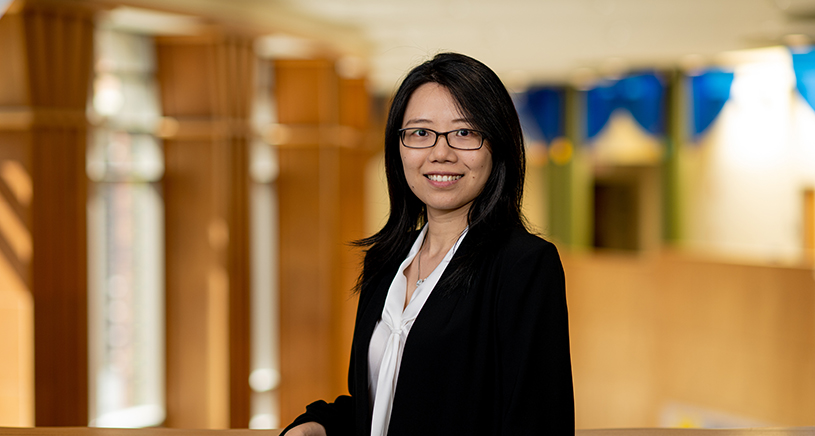
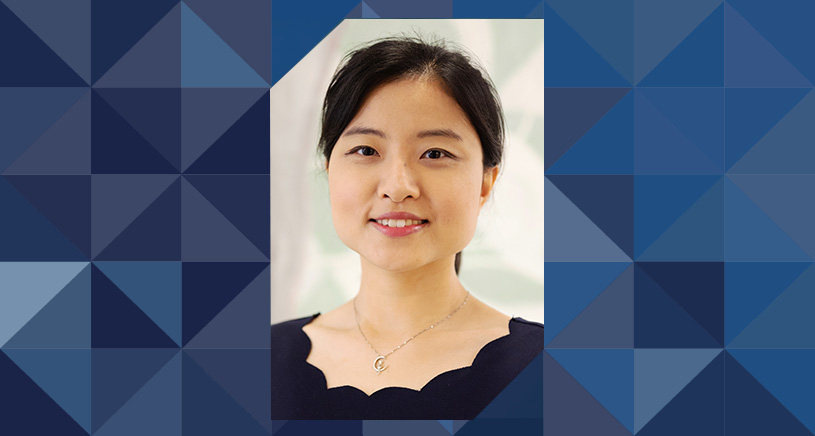
Department | Program | Degree | Competency | Specific course(s) that allow assessment | BIOSTAT | MS | Derive the theoretical mathematics of statistical inferences | BIOSTAT602 |
---|
BIOSTAT607: Basic Computing For Data Analytics
- Graduate level
- Residential
- Fall term(s) for residential students;
- 1-3 credit hour(s) for residential students;
- Instructor(s): Nicholas Henderson (Residential);
- Prerequisites: No other courses
- Advisory Prerequisites: It is recommended that students with no programming knowledge co-enroll in Biostat 600 or contact the instructor to discuss the best options
- Description: This course is designed as a 3-credit modular course focusing on basic programming skills, including Python (1 credit), R (1 credit), and C++ (1 credit). The course covers key features of each programming language, data structures, basic data processing skills, basic data visualization skills (for Python and R), and basic UNIX skills. Students are allowed to take one or more modules according to the need basis.
- Learning Objectives: (a) To understand key features of R, python, and C++ programming languages in a modular way. (b) To understand basic data structures, basic data processing skills, basic data visualization skills (for Python and R modules), and basic UNIX skills
- Syllabus for BIOSTAT607
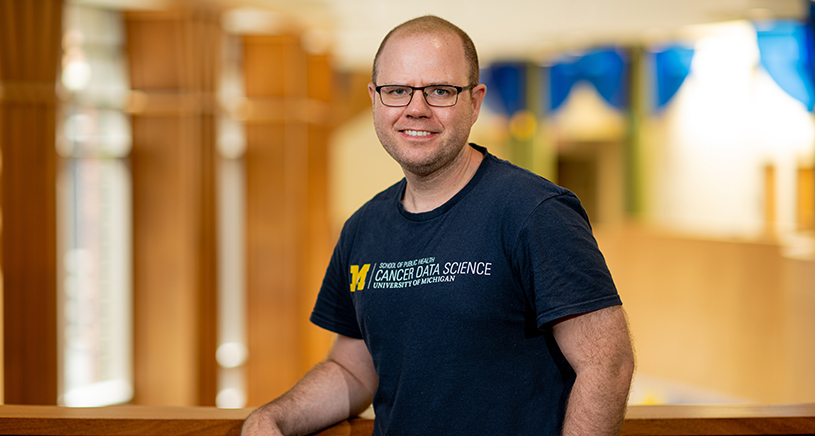
BIOSTAT610: Readings in Biostatistics
- Graduate level
- Residential
- Fall, Winter term(s) for residential students;
- 1-4 credit hour(s) for residential students;
- Instructor(s): Staff (Residential);
- Prerequisites: One of Biostat 503, Biostat 524, Biostat 553 or Biostat 601/Biostat 602
- Description: Independent study in a special topic under the guidance of a faculty member. May be elected more than once. Enrollment is limited to biostatistics majors.
BIOSTAT611: Grad School And Professional Success Skills
- Graduate level
- Residential
- Fall term(s) for residential students;
- 1 credit hour(s) for residential students;
- Instructor(s): Kelley Kidwell, Jennifer Daniels, (Residential);
- Prerequisites: None
- Advisory Prerequisites: N/A
- Description: This course complements the Biostatistics curriculum in providing more tailored content to succeed as a student, on the job market, and professionally on the job. This discussion includes didactic lectures and activities led by instructors and guest speakers.
- Learning Objectives: Learn skills to survive and thrive as a Biostatistics MS student. Discuss the variety of career opportunities for biostatisticians. Prepare for internship and job opportunities. Learn skills to be a successful professional across a variety of fields.
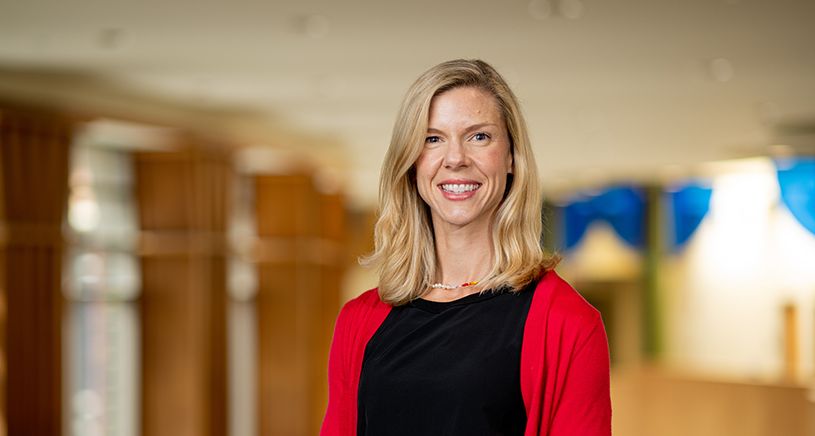
BIOSTAT612: Grad School And Professional Success Skills
- Graduate level
- Residential
- Winter term(s) for residential students;
- 1 credit hour(s) for residential students;
- Instructor(s): Jennifer Daniels, Hui Jiang, (Residential);
- Prerequisites: None
- Description: This course complements the Biostatistics curriculum in providing more tailored content to succeed as a student, on the job market, and professionally on the job. This discussion includes didactic lectures and activities led by instructors and guest speakers.
- Learning Objectives: Continuation of Biostat 611: Learn skills to survive and thrive as a Biostatistics MS student. Discuss the variety of career opportunities for biostatisticians. Prepare for internship and job opportunities. Learn skills to be a successful professional across a variety of fields.
- Syllabus for BIOSTAT612
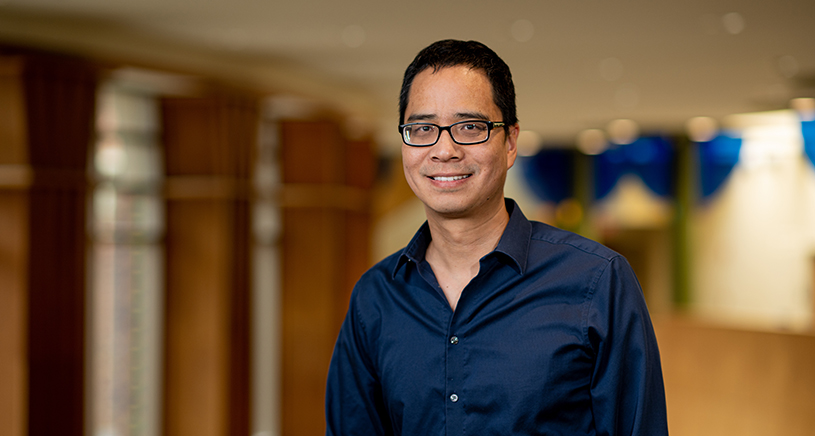
BIOSTAT615: Statistical Computing
- Graduate level
- Residential
- Fall term(s) for residential students;
- 3 credit hour(s) for residential students;
- Instructor(s): Hyun Min Kang (Residential);
- Prerequisites: None
- Description: A survey of key algorithms for statistical computing and its applications in Biostatistics. The course will cover fundamental computational techniques for dynamic programming, sorting, and searching, as well statistical methods for random number generation, numerical integration, function optimization, Markov-Chain Monte Carlo, and the E-M algorithm. Enables students to understand numerical results produced by a computer and to implement their own statistical methods.
- Syllabus for BIOSTAT615
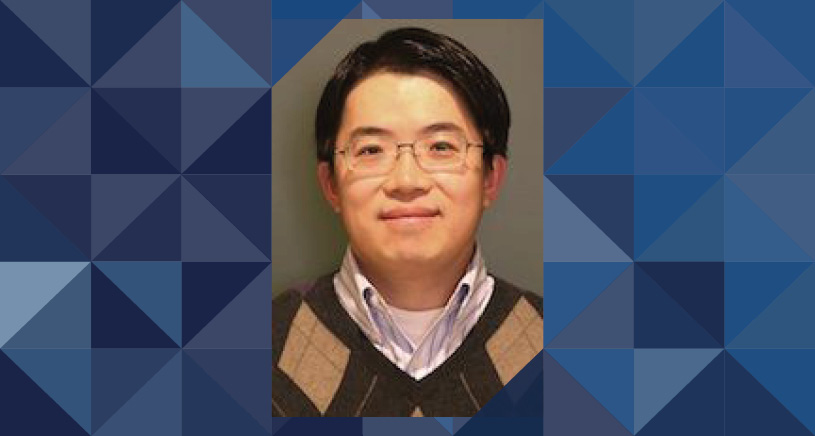
BIOSTAT617: Theory and Methods of Sample Design (Soc 717 and Stat 580 and SurvMeth 617)
- Graduate level
- Residential
- Winter term(s) for residential students;
- 3 credit hour(s) for residential students;
- Instructor(s): Trivellore Raghunathan (Residential);
- Prerequisites: Three or more courses in statistics, and preferably a course in methods of survey sampling
- Description: Theory underlying sample designs and estimation procedures commonly used in survey practice.
- This course is cross-listed with Stats 580 Soc 717 SurvMeth617 in the Rackham department.
- Syllabus for BIOSTAT617
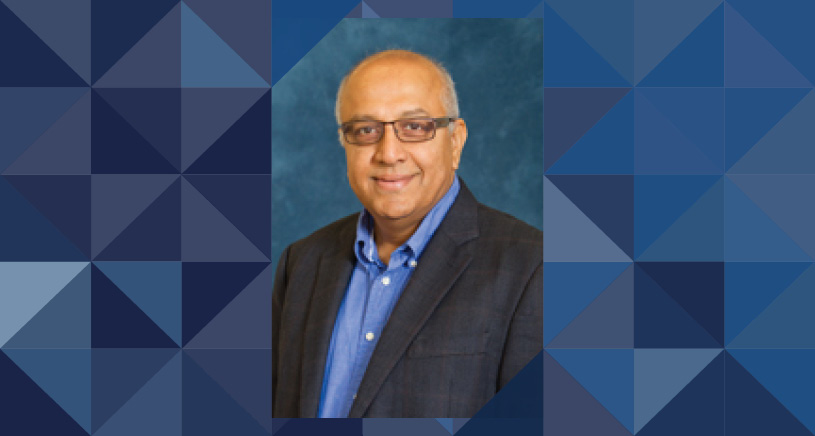
BIOSTAT619: Clinical Trials
- Graduate level
- Residential
- Fall term(s) for residential students;
- 3 credit hour(s) for residential students;
- Instructor(s): Staff (Residential);
- Prerequisites: Biostatistics 601 and Biostatistics 651
- Description: This course is designed for individuals with a strong quantitative background who are interested in the statistical design and analysis aspects of clinical trials and the interface between statistics and policy in this area
- Syllabus for BIOSTAT619
BIOSTAT620: Introduction to Health Data Science
- Graduate level
- Residential
- Winter term(s) for residential students;
- 3 credit hour(s) for residential students;
- Instructor(s): Staff (Residential);
- Prerequisites: BIOSTAT 607, BIOSTAT 601, BIOSTAT 650
- Advisory Prerequisites: No other courses
- Description: This course offers a systematic introduction to the scope and contents of health data arising from public health and the biomedical sciences. It focuses on rules and techniques for handling health data. Through both regular lectures and guest lectures, this course covers a broad range of health data.
- Learning Objectives: (a) To understand the foundation and rules for handling big health data. (b) To develop a practical knowledge and understanding of important statistical issues and relevant data analytics for health big data analysis. (c) To learn and master basic software and programming skills for data cleaning and data processing.
- Syllabus for BIOSTAT620
Department | Program | Degree | Competency | Specific course(s) that allow assessment | BIOSTAT | Health Data Science | MS | Understand the roles and principles when a biostatistician conducts the analysis of biomedical or public health data | BIOSTAT620 | BIOSTAT | Health Data Science | MS | Distinguish among the different measurement scales and data quality, as well as their implications for selection of statistical methods and algorithms to be used based on these distinctions | BIOSTAT620 |
---|
BIOSTAT625: Computing with Big Data
- Graduate level
- Residential
- Fall term(s) for residential students;
- 3 credit hour(s) for residential students;
- Instructor(s): Hui Jiang (Residential);
- Prerequisites: R module in BIOSTAT 607 or equivalent.
- Description: This course will cover techniques for computing with big data. The topics include programming, data processing, debugging, profiling and optimization, version control, software development, interfacing with databases, interfacing between programming languages, visualization, high performance and cloud computing. Hands-on experience will be emphasized in lectures, homework assignments and projects.
- Learning Objectives: (a) To master techniques for manipulating and processing big data by writing customized computer programs. (b) To understand the foundation for the computing aspects of data science. (c) To have a practical understanding of important computing issues for health big data analysis.
- Syllabus for BIOSTAT625
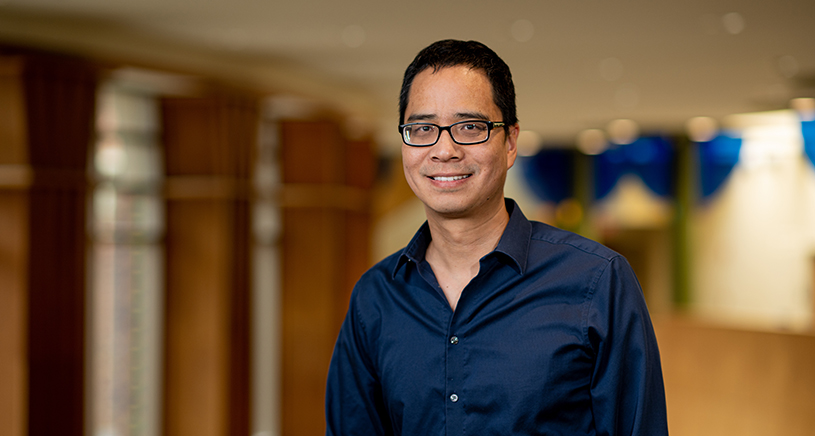
Department | Program | Degree | Competency | Specific course(s) that allow assessment | BIOSTAT | Health Data Science | MS | Apply basic informatics and computational techniques in the analysis of big health data, and interpret results of statistical analysis | BIOSTAT625 |
---|
BIOSTAT626: Machine Learning For Health Sciences
- Graduate level
- Residential
- Winter term(s) for residential students;
- 3 credit hour(s) for residential students;
- Instructor(s): William Wen (Residential);
- Prerequisites: BIOSTAT 601
- Description: This is a 3-credit course introducing modern machine learning algorithms and data analytics for prediction, classification and data pattern recognition, with an emphasis on their applications in health data sciences.
- Learning Objectives: (a) To understand the foundation and rules to use machine learning techniques for handling data from the health sciences (b) To develop practical knowledge and understanding of modern machine learning techniques for health big data analysis. (c) To learn and master basic software and programming skills to apply machine learning algorithms in analyzing data arising from the health sciences.
- Syllabus for BIOSTAT626
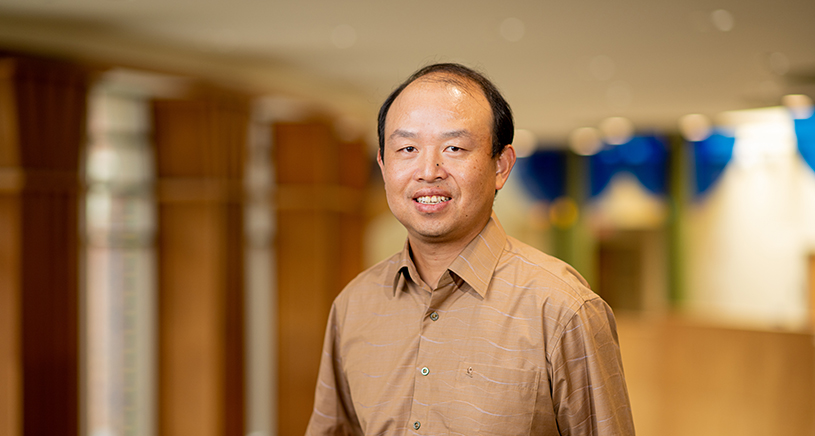
BIOSTAT629: Case Studies In Health Big Data
- Graduate level
- Residential
- Winter term(s) for residential students;
- 3 credit hour(s) for residential students;
- Instructor(s): Nicholas Henderson, Walter Dempsey, (Residential);
- Prerequisites: Biostatistics or Health Data Science students only
- Description: Being a project-based course, it integrates all competencies learned in HDS MS program to provide a culminating research experience. Students will work on two to three health big data projects, through which they learn to identify scientific objectives and analytical strategies and report findings through oral presentation and written documents.
- Learning Objectives: Students will learn how to identify a scientific goal of the project and to develop analytic strategies. Students will learn to integrate and apply quantitative skills to handle real-world health big data, including data modification and cleaning, data visualization and scalable computing. From presentations, students will improve their communication skills.
- Syllabus for BIOSTAT629
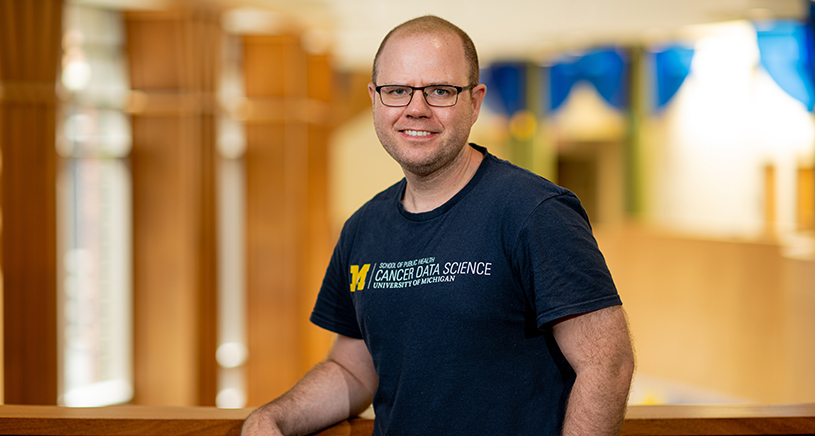
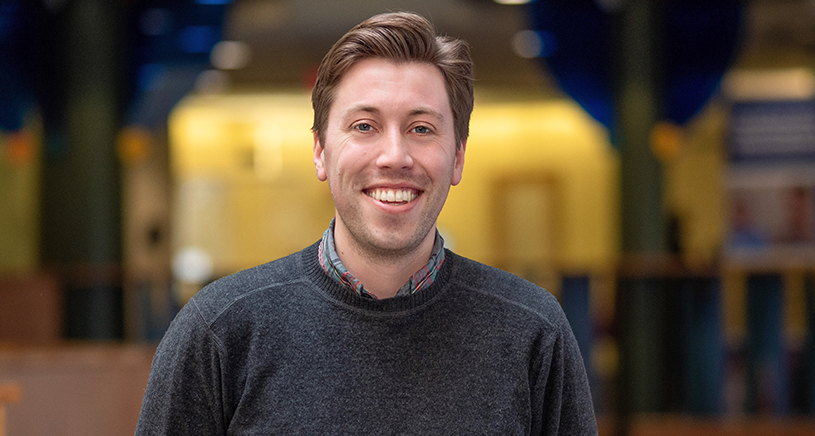
Department | Program | Degree | Competency | Specific course(s) that allow assessment | BIOSTAT | Health Data Science | MS | Apply quantitative techniques commonly used to summarize and display big public health data | BIOSTAT629 | BIOSTAT | Health Data Science | MS | Apply descriptive and inferential methodologies according to the type of study design or sampling technique for answering a particular public health question | BIOSTAT629 |
---|
BIOSTAT640: Data Science Seminar
- Graduate level
- Residential
- Fall term(s) for residential students;
- 1 credit hour(s) for residential students;
- Instructor(s): H.V. Jagadish (Residential);
- Not offered 2024-2025
- Prerequisites: None
- Description: The MIDAS Seminar Series features leading data scientists from around the world and across the U-M campuses addressing a variety of topics in data science, and sharing their vision regarding the future of the field. These thought leaders are invited from academia, industry and government.
- Learning Objectives: To learn about the a wide variety of research and application in data science in both academic and business settings.
- This course is cross-listed with EECS409 in the Engineering/LSA/SOI department.
BIOSTAT642: Introduction to Functional MRI
- Graduate level
- Residential
- Fall term(s) for residential students;
- 3 credit hour(s) for residential students;
- Instructor(s): Staff (Residential);
- Not offered 2024-2025
- Prerequisites: None
- Description: This course presents the basic skills to design and analyze functional magnetic resonance imaging (fMRI) experiments. We start by reviewing the basic Matlab and Unix skills necessary to manipulate image data. Next we introduce the principles of MRI and the nature of the Blood Oxygenation Level Dependent (BOLD) effect, including artifacts that corrupt the BOLD signal. We cover blocked and event-related designs, and how to optimize statistical power of design. We cover subject safety.
- Syllabus for BIOSTAT642
BIOSTAT645: Time Series Analysis with Biomedical Applications
- Graduate level
- Residential
- Fall term(s) for residential students;
- 3 credit hour(s) for residential students;
- Instructor(s): Staff (Residential);
- Not offered 2024-2025
- Prerequisites: Biostat 602, Biostat 650 or Perm. Instr
- Description: Introduction to statistical time series analysis with an emphasis on frequency domain (spectral) methods and their applications to biomedical problems. Topics include autocorrelation, stationarity, autoregressive and moving average processes, power spectra, periodgrams, spectral estimation, linear filters, complex demodulation, autoregressive integrated moving average (ARIMA) models, cross-correlation, cross-spectra, coherence, time and frequency domain linear regression. The methods will be illustrated in applications to various areas of public health and medical research such as environmental health, electrophysiology, and endocrinology.
- Syllabus for BIOSTAT645
BIOSTAT646: High Throughput Molecular Genetic and Epigenetic Data Analysis
- Graduate level
- Residential
- Winter term(s) for residential students;
- 3 credit hour(s) for residential students;
- Instructor(s): Alex Tsoi (Residential);
- Prerequisites: Graduate Standing and STAT400, BIOSTAT522, or BIOSTAT521 or permission of instructor
- Description: The course will cover statistical methods used to analyze data in experimental molecular biology. The course will primarily cover topics relating to gene expression data analysis, but other types of data such as genome sequence and epigenomics data that is sometimes analyzed in concert with expression data will also be covered.
- Syllabus for BIOSTAT646
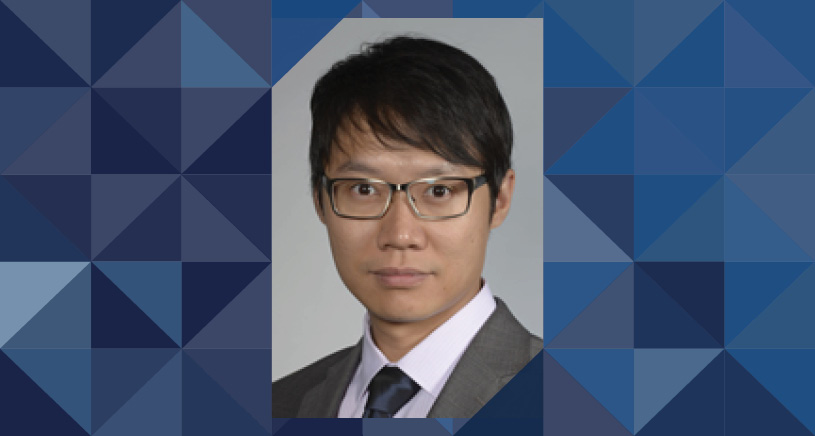
BIOSTAT650: Theory and Application of Linear Regression
- Graduate level
- Residential
- Fall term(s) for residential students;
- 4 credit hour(s) for residential students;
- Instructor(s): Lu Wang, Mousumi Banerjee, (Residential);
- Prerequisites: BIOSTAT601
- Description: Graphical methods, simple and multiple linear regression; simple, partial and multiple correlation; estimation; hypothesis testing, model building and diagnosis; introduction to nonparametric regression; introduction to smoothing methods (e.g., lowess) The course will include applications to real data.
- Syllabus for BIOSTAT650
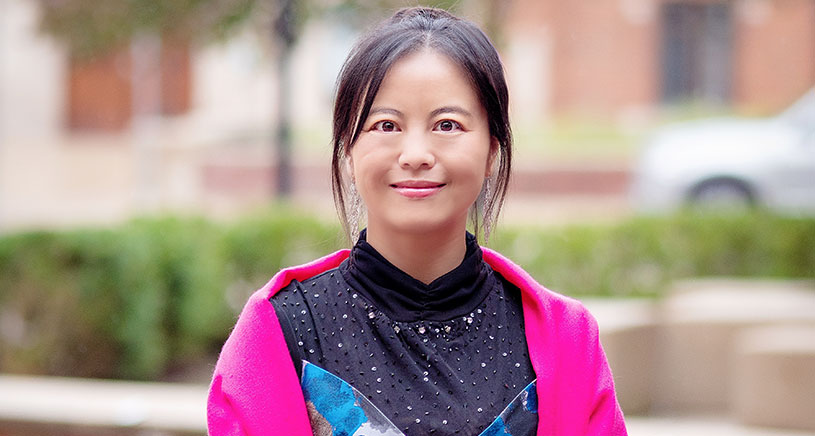
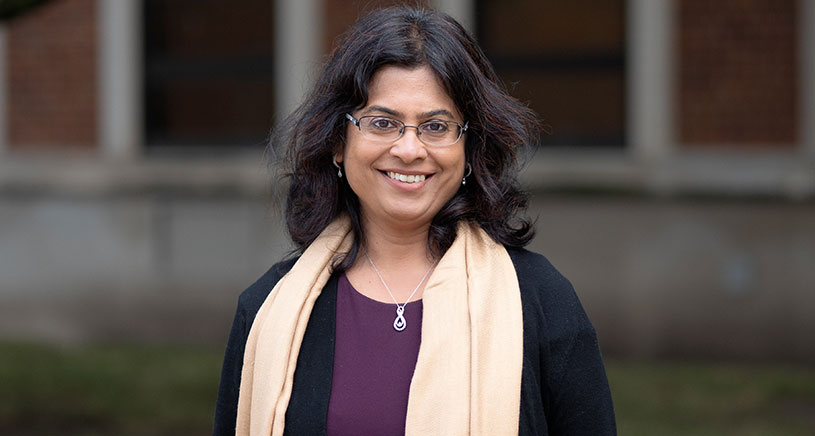
Department | Program | Degree | Competency | Specific course(s) that allow assessment | BIOSTAT | MS | Perform linear regression model fitting and diagnosis | BIOSTAT650 | BIOSTAT | PhD | Perform linear regression model fitting and diagnosis | BIOSTAT650 |
---|
BIOSTAT651: Theory and Application of Generalized Linear Models
- Graduate level
- Residential
- Winter term(s) for residential students;
- 3 credit hour(s) for residential students;
- Instructor(s): Veera Baladandayuthapani, Menggang Yu, (Residential);
- Prerequisites: BIOSTAT601 and BIOSTAT650
- Description: Introduction to maximum likelihood estimation; exponential family; proportion, count and rate data; generalized linear models; link function; logistic and Poisson regression; estimation; inference; deviance; diagnosis. The course will include application to real data.
- Syllabus for BIOSTAT651
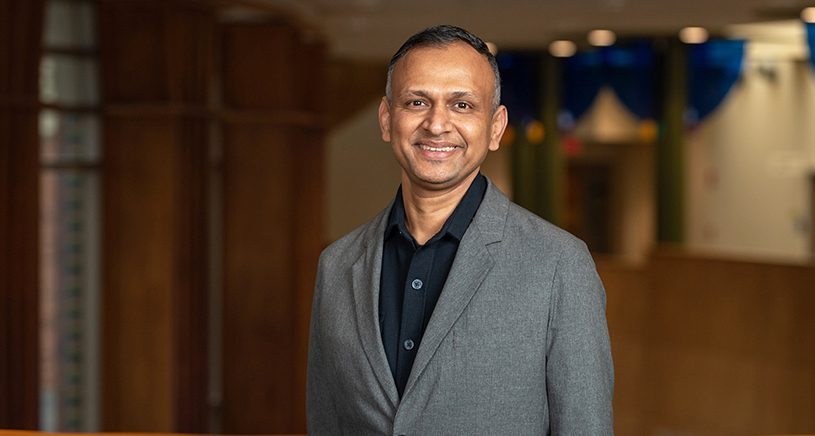
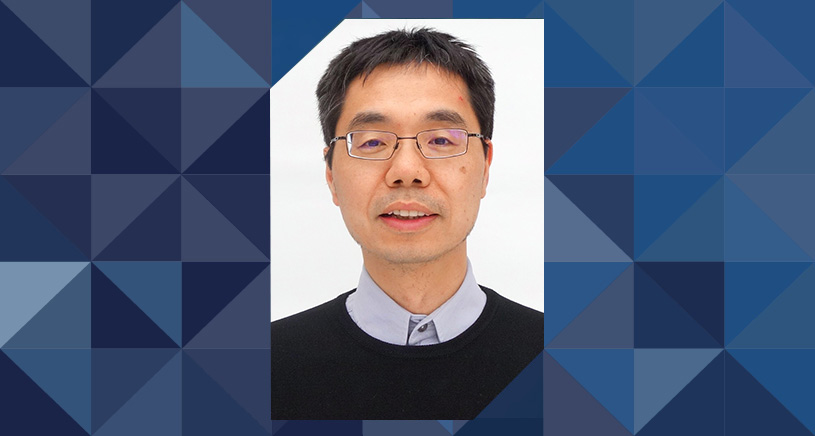
Department | Program | Degree | Competency | Specific course(s) that allow assessment | BIOSTAT | MS | Perform generalized linear regression model fitting and diagnosis | BIOSTAT651 | BIOSTAT | PhD | Perform generalized linear regression model fitting and diagnosis | BIOSTAT651 |
---|
BIOSTAT653: Theory and Application of Longitudinal Analysis.
- Graduate level
- Residential
- Fall term(s) for residential students;
- 3 credit hour(s) for residential students;
- Instructor(s): Zhenke Wu, Kevin (Zhi) He, (Residential);
- Prerequisites: BIOSTAT650 and concurrent enrollment in BIOSTAT651
- Description: This course overviews the statistical models and methodologies for analyzing repeated measures/longitudinal data. It covers general linear models and linear mixed models for analyzing correlated continuous data, as well as marginal (i.e. GEE), conditional (i.e. generalized linear mixed model) and transition models for analyzing correlated discrete data.
- Syllabus for BIOSTAT653
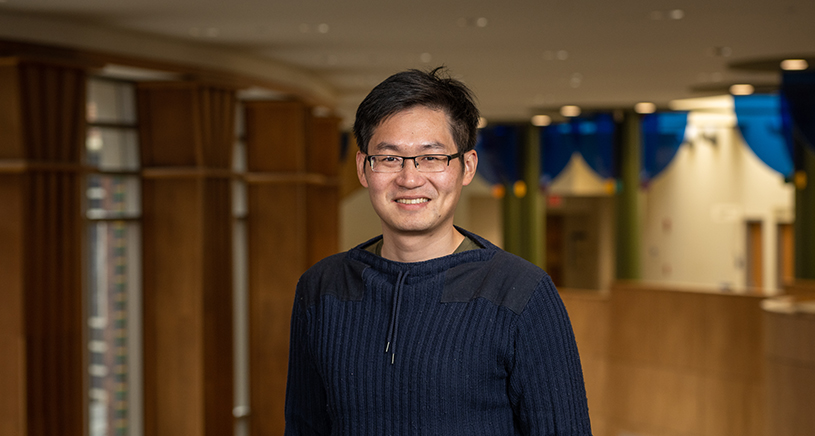
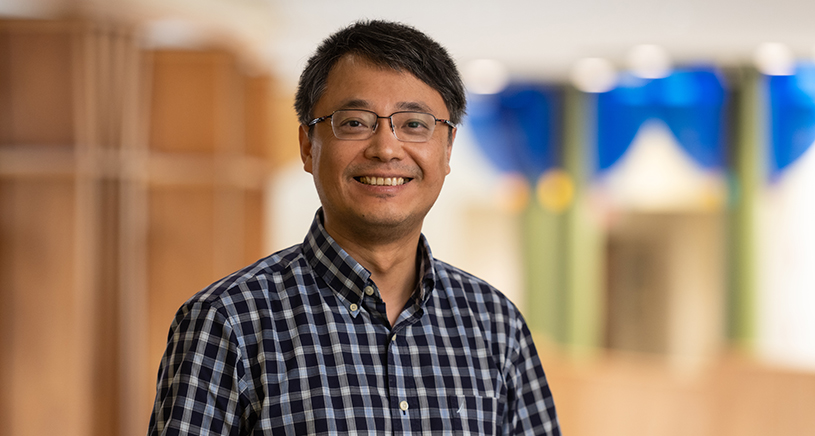
Department | Program | Degree | Competency | Specific course(s) that allow assessment | BIOSTAT | MS | Perform ANOVA analysis and longitudinal data analysis | BIOSTAT653 | BIOSTAT | PhD | Perform statistical analysis for longitudinal data and correlated data | BIOSTAT653 |
---|
BIOSTAT664: Special Topics in Biostastics
- Graduate level
- Residential
- Winter term(s) for residential students;
- 1-4 credit hour(s) for residential students;
- Instructor(s): Staff (Residential);
- Not offered 2024-2025
- Prerequisites: Permission of instructor
- Description: Master's level seminar designed to provide an extensive review of a number of substantive and methods and skill areas in biostatistics. Readings, discussion, and assignments are organized around issues of mutual interest to faculty and students. Reviews and reports on topics required in the areas selected. May be elected more than once.
- Syllabus for BIOSTAT664
BIOSTAT665: Statistical Population Genetics
- Graduate level
- Residential
- Winter term(s) for residential students;
- 3 credit hour(s) for residential students;
- Instructor(s): Staff (Residential);
- Not offered 2024-2025
- Prerequisites: None
- Description: The first half of the course concentrates on classical population genetics. We introduce topics such as Hardy-Weinberg equilibrium, models of selection for populations of infinite size and population subdivision. The second half of the course focuses on coalescent theory, covering migration, changes in population size and recombination. We provide guidelines how these models can be used in to infer population genetic parameters. Finally, some recent results and methods from the population genetic literature are discussed.
- Syllabus for BIOSTAT665
BIOSTAT666: Statistical Models and Numerical Methods in Human Genetics
- Graduate level
- Residential
- Winter term(s) for residential students;
- 3 credit hour(s) for residential students;
- Instructor(s): Sebastian Zoellner (Residential);
- Prerequisites: Biostat 602 or Perm. Instr.
- Description: Introduction to current statistical methods used in human genetics. Topics will include sampling designs in human genetics, gene frequency estimation, the coalescent method for simulation of DNA sequences, linkage analysis, tests of association, detection of errors in genetic data, and the multi-factorial model. The course will include a simple overview of genetic data and terminology and will proceed with a review of numerical techniques frequently employed in human genetics.
- Syllabus for BIOSTAT666
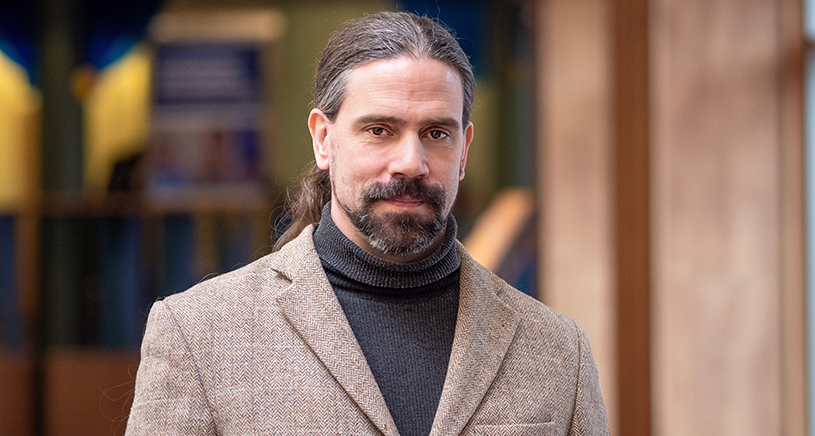
BIOSTAT675: Survival Time Analysis
- Graduate level
- Residential
- Winter term(s) for residential students;
- 3 credit hour(s) for residential students;
- Instructor(s): Yi Li (Residential);
- Prerequisites: Biostat 602 and Biostat 650
- Description: Concepts and methods for analyzing survival time data obtained from following individuals until occurrence of an event or their loss to follow-up. Survival time models, clinical life tables, survival distributions, mathematical and graphical methods for evaluating goodness of fit, comparison of treatment groups, regression models, proportional hazards models, censoring mechanisms.
- Syllabus for BIOSTAT675
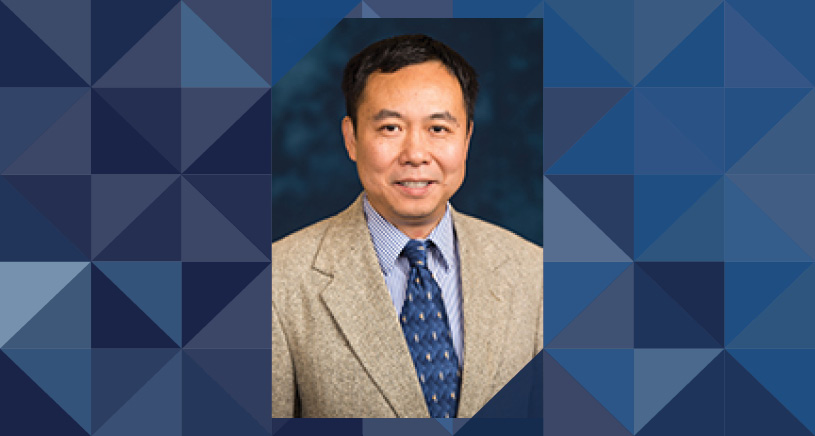
BIOSTAT680: Applications of Stochastic Processes I
- Graduate level
- Residential
- Fall term(s) for residential students;
- 3 credit hour(s) for residential students;
- Instructor(s): William Wen (Residential);
- Prerequisites: Biostat 601 and Math 450 or equiv
- Description: Conditional distributions, probability generating functions, convolutions, discrete and continuous parameter, Markov chains, medical and health related applications.
- Syllabus for BIOSTAT680
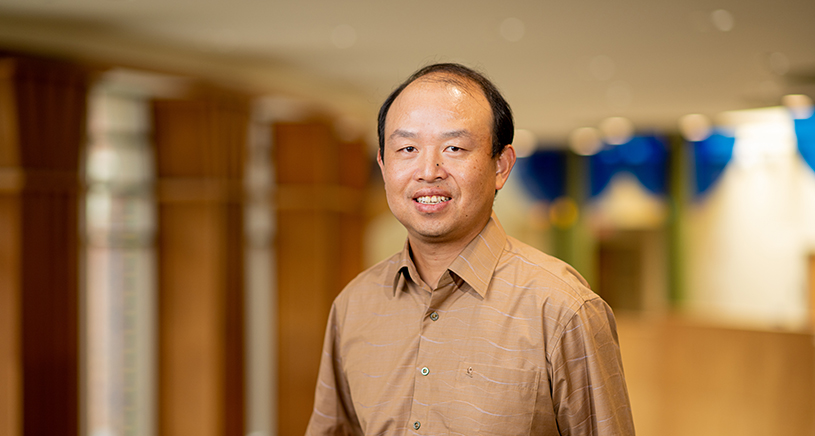
BIOSTAT681: Introduction to Causal Inference
- Graduate level
- Residential
- Fall term(s) for residential students;
- 3 credit hour(s) for residential students;
- Instructor(s): Michael Elliot (Residential);
- Prerequisites: None
- Advisory Prerequisites: Biostats 601, 602, 650, and 651
- Description: This course is designed to introduce students to basic of causal inference, including potential outcomes, counterfactuals, confounding, mediation, and instrumental variables. We will explore the identification and estimation of causal effects via the use of principle stratification, marginal structural models, and directed acyclic graphs.
- Learning Objectives: At the end of the course, students should be able to -Define concepts including potential outcomes, confounding, and mediation. -Explain the purpose of randomization for causal inference. -Explain the concept of principal stratification/casual association and use the relevant statistical methods to make causal inference under the casual association paradigm. -Explain the concepts of direct and indirect effects/casual effects and use the relevant statistical methods to make causal inference under the casual effects paradigm. -Understand how instrumental variables can be related to other causal inference approaches. -Consider the use of directed acyclic graphs (DAGs) to define causal concepts and derive conditions of identifiability.
- Syllabus for BIOSTAT681
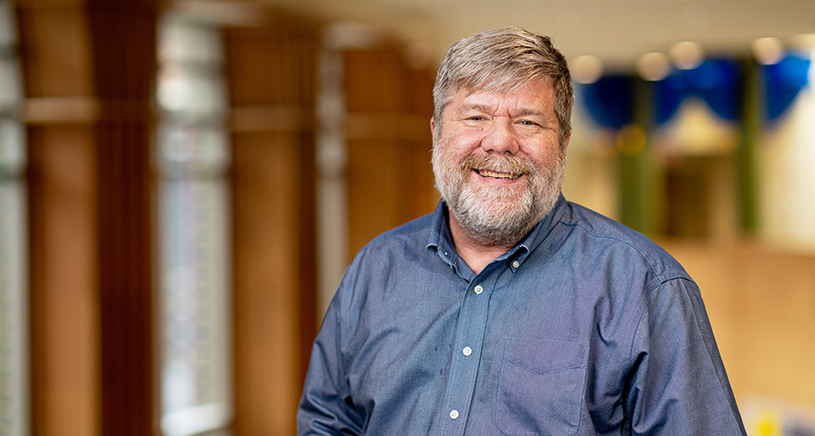
BIOSTAT682: Applied Bayesian Inference
- Graduate level
- Residential
- Fall term(s) for residential students;
- 3 credit hour(s) for residential students;
- Instructor(s): Jian Kang (Residential);
- Prerequisites: Biostat 602, Biostat 650 and Biostat 651
- Description: Introduction to Bayesian Inference. Bayesian large sample inference, relationship with maximum likelihood. Choice of model, including prior distribution. Bayesian approaches to regression generalized linear models, categorical data, and hierarchical models. Empirical Bayes methods. Comparison with frequentist methods. Bayesian computational methods. Assessment of sensitivity to model assumptions. Emphasis on biomedical applications.
- Syllabus for BIOSTAT682
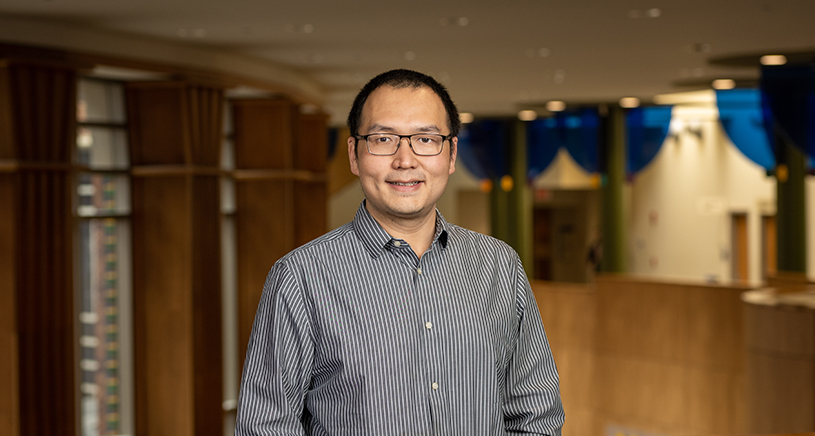
BIOSTAT685: Elements of Nonparametric Statistics
- Graduate level
- Residential
- Winter term(s) for residential students;
- 3 credit hour(s) for residential students;
- Instructor(s): Thomas Braun (Residential);
- Prerequisites: Biostat 602 or STAT 511, and Biostat 650 or Perm. Instr
- Description: First half covers theory and applications of rank and randomization tests: sampling and randomization models, randomization t-test, Wilcoxon rank sum and signed rank tests, Kruskal-Wallis test, asymptotic result under randomization, relative efficiency; second half covers theory and applications of nonparametric regression: smoothing methods, including kernel estimators, local linear regression, smoothing splines, and regression splines, methods for choosing the smoothing parameter, including unbiased risk estimation and cross-validation, introduction to additive models.
- Syllabus for BIOSTAT685
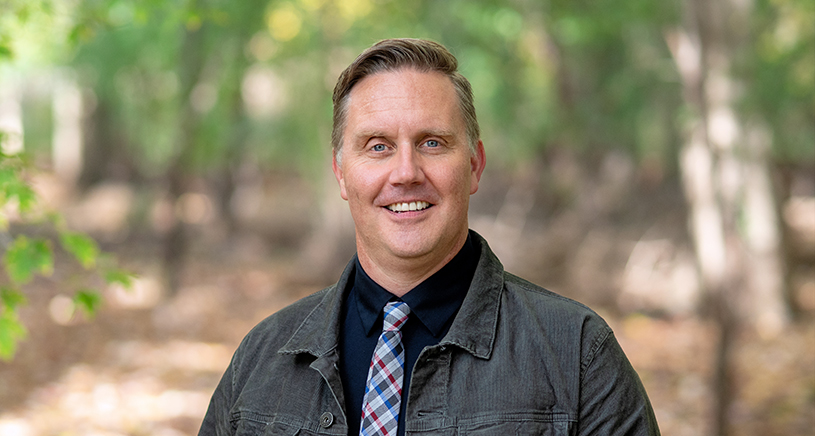
BIOSTAT687: Statistical Methods For Health Disparity Research
- Graduate level
- Residential
- Fall term(s) for residential students;
- 3 credit hour(s) for residential students;
- Instructor(s): Trivellore Raghunathan (Residential);
- Prerequisites: Biostat 601, 602, 650 and 651 or equivalents
- Advisory Prerequisites: Biostat 682 or Biostat 675
- Description: Healthy People initiatives over the past several decades have been developed to eliminate health disparities. The objective of this course is to consider several statistical methodological issues in assessing and understanding the reasonings behind health disparities. There is a strong need for bringing together a set of statistical methods to address design, measurement and analysis tuned to health disparity research.
- Learning Objectives: .1. Understand historical perspectives on health disparities and determinants of health 2. Understand various measures of health disparity and methods for constructing inferences about them 3. Understand methods for analyzing data from complex surveys 4. Understand methods for assessing psychometric properties of measurements used in health disparity research across subpopulations through measurement error and Item response theory modeling 5. Understand statistical techniques to assess the reasons for health disparity using regression, propensity score and causal inferential techniques 6. Understand small area/domain estimation techniques to understand geographical variation in health disparities
- Syllabus for BIOSTAT687
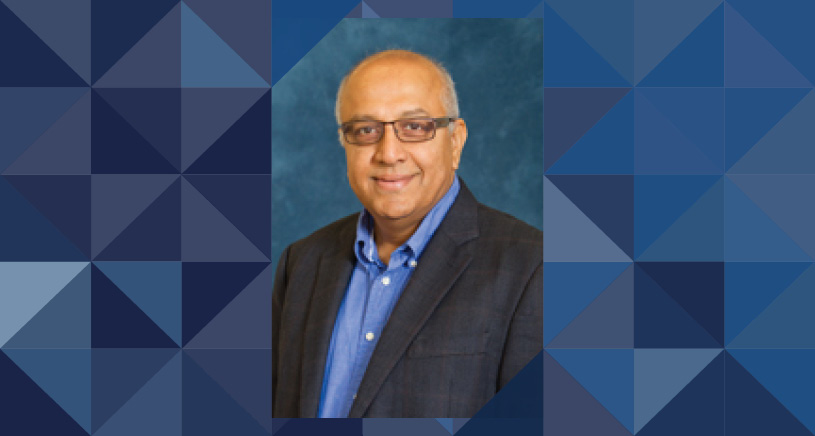
BIOSTAT690: Health Applications of Multivariate Analysis
- Graduate level
- Residential
- Fall, Winter term(s) for residential students;
- 3 credit hour(s) for residential students;
- Instructor(s): Staff (Residential);
- Not offered 2024-2025
- Prerequisites: Biostat 650 and Biostat 651 and Math 417 or Perm. Instr.
- Description: Techniques of multivariate analysis related to health and biomedical problems. Emphasis on computational techniques and programs with health examples. Tests of significance for one, two or more populations; general linear model; multivariate analyses of variances and covariances; correlation procedures; principal components and discriminant analyses.
- Syllabus for BIOSTAT690
BIOSTAT695: Analysis Of Categorical Data
- Graduate level
- Residential
- Winter term(s) for residential students;
- 3 credit hour(s) for residential students;
- Instructor(s): Timothy Johnson (Residential);
- Prerequisites: Biostat 602, 650. Biostat 651 corequisite
- Description: Regression models for the analysis of categorical data: logistic, probit and complementary log-log models for binomial random variables; log-linear models for cross-classifications of counts; regression models for Poisson rates; and multinomial response models for both nominal and ordinal responses. Model specification and interpretation are emphasized, and model criticism, model selection.
- Syllabus for BIOSTAT695
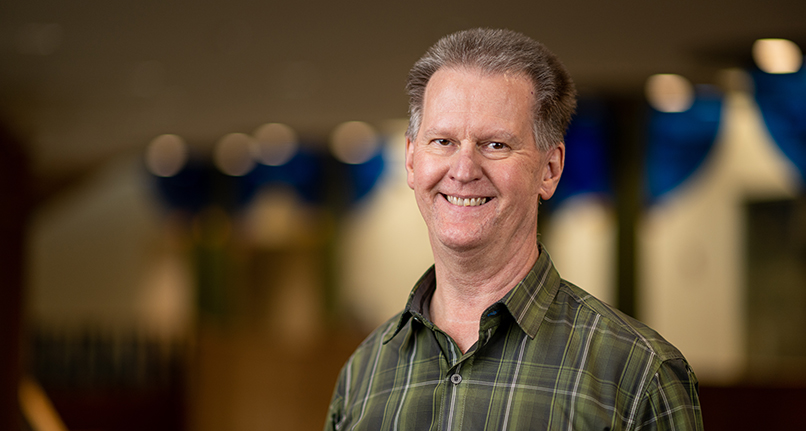
BIOSTAT696: Spatial Statistics
- Graduate level
- Residential
- Winter term(s) for residential students;
- 3 credit hour(s) for residential students;
- Instructor(s): Michele Peruzzi (Residential);
- Prerequisites: BIOSTAT 601, BIOSTAT 602, BIOSTAT 650, BIOSTAT 653
- Description: This course will introduce the theory and methods of spatial and spatio-temporal statistics. It will present spatial and spatio-temporal statistical models and will discuss methods for inference on spatial processes within a geostatistical and a hierarchical Bayesian framework.
- Syllabus for BIOSTAT696
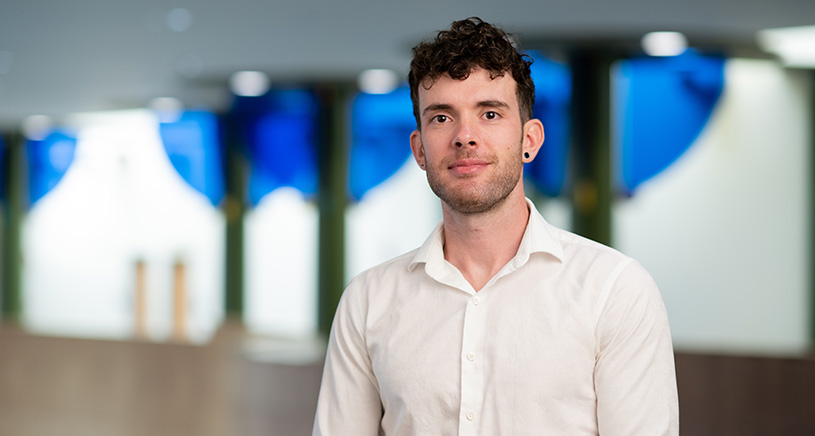
BIOSTAT698: Modern Statistical Methods in Epidemiologic Studies
- Graduate level
- Residential
- Fall term(s) for residential students;
- 4 credit hour(s) for residential students;
- Instructor(s):
- Not offered 2024-2025
- Prerequisites: EPID600, BIOSTAT522 and BIOSTAT523 for epid students; BIOSTAT650, BIOSTAT651 for biostat students
- Advisory Prerequisites: EPID 798 for epid students; BIOSTAT 695 for Biostat students
- Description: The goal of this pilot course is to create an interdisciplinary educational experience for Ph.D. students in Epidemiology (also available as an optional elective for Masters students in Biostatistics) through a uniquely designed course that contains lectures on advanced biostatistical methods, but places them in the context of epidemiological applications.
- This course is cross-listed with EPID815.
- Syllabus for BIOSTAT698
BIOSTAT699: Analysis of Biostatistical Investigations
- Graduate level
- Residential
- Winter term(s) for residential students;
- 4 credit hour(s) for residential students;
- Instructor(s): Jeremy Taylor, Irina Gaynanova, Jean Morrison, Philip Boonstra, (Residential);
- Prerequisites: Registration for last term of studies to complete MS or MPH
- Description: Identifying and solving design and data analysis problems using a wide range of biostatistical methods. Written and oral reports on intermediate and final results of case studies required.
- Syllabus for BIOSTAT699
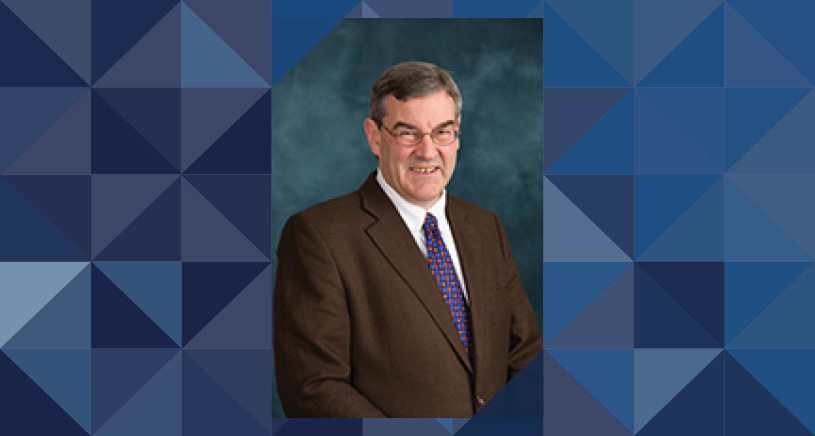
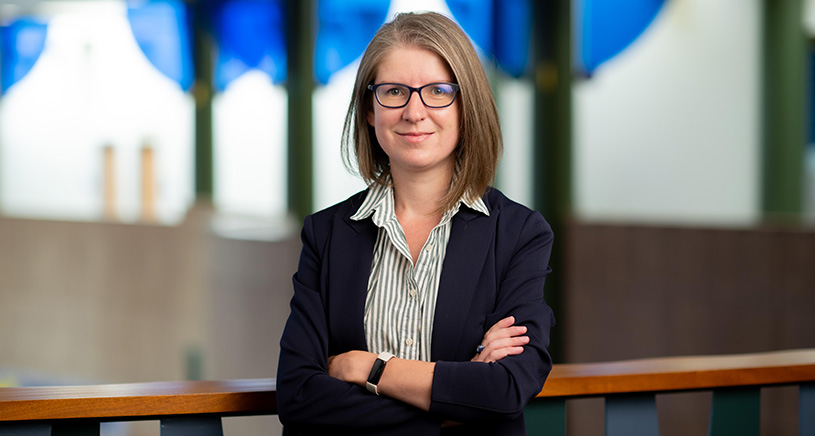
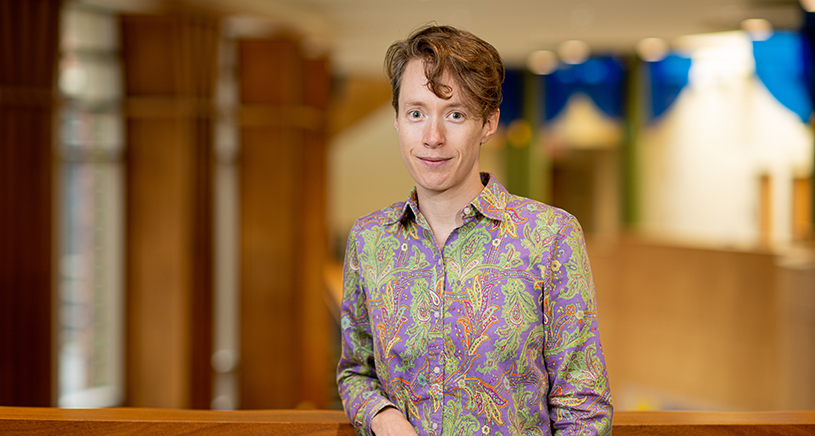
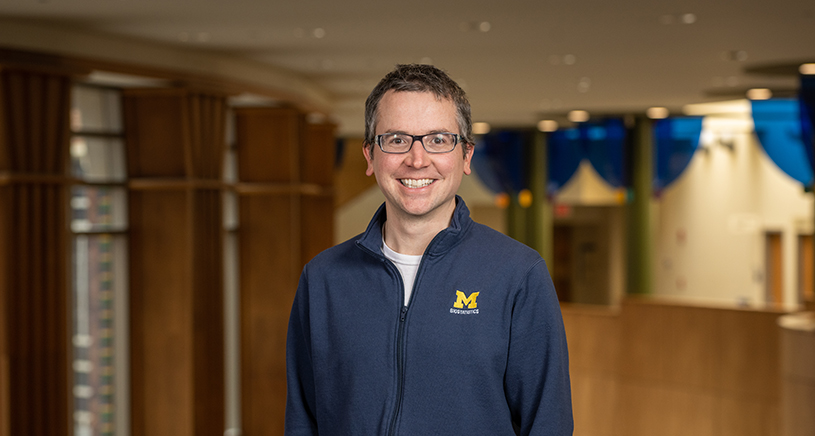
Department | Program | Degree | Competency | Specific course(s) that allow assessment | BIOSTAT | MS | Interpret the results of statistical analysis in a variety of health-related areas (e.g. public health, medicine, genetics, biology, psychology, economics, management and policy, nursing, or pharmacy) for the broad scientific community | BIOSTAT699 | BIOSTAT | MS | Communicate statistical analysis through written scientific reporting for public health, medical, and basic scientists, and/or educated lay audiences | BIOSTAT699 | BIOSTAT | PhD | Communicate through written and oral presentation based on statistical analysis for audience from a variety of health-related areas (e.g. public health, medicine, genetics, biology, psychology, nursing, or pharmacy) and for the broad scientific community | BIOSTAT699 |
---|
BIOSTAT800: Seminar in Biostatistics
- Graduate level
- Residential
- Fall, Winter term(s) for residential students;
- 0.5 credit hour(s) for residential students;
- Instructor(s): Fan Bu, Michele Peruzzi, (Residential);
- Prerequisites: Graduate level Biostatistics students only
- Description: Presentations and discussions of current consulting and research problems. Enrollment limited to biostatistics majors. Students must attend 2/3 of all seminars offered during the semester to receive credit. Maximum credit is 0.5 per semester. No more than 1 credit total allowed. May only be taken a maximum of 2 semesters.
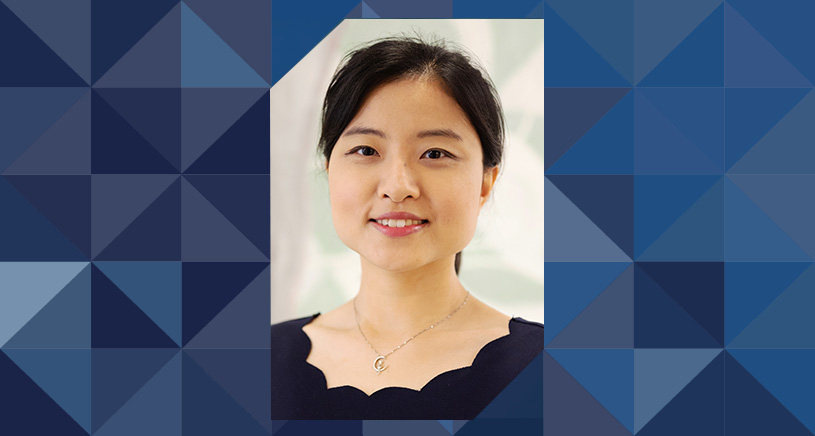
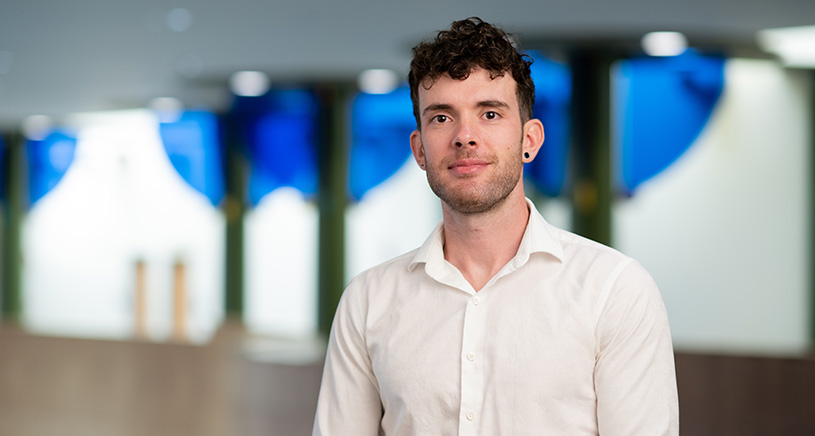
BIOSTAT801: Advanced Inference I
- Graduate level
- Residential
- Fall term(s) for residential students;
- 3 credit hour(s) for residential students;
- Instructor(s): Alexander Tsodikov (Residential);
- Prerequisites: Biostat 601, Biostat 602, and MATH 451 or equivalent
- Description: This is the first course of the sequence that covers advanced topics in probability theory, theory of point estimation, theory of hypothesis testing, and related large sample theory. This sequence replaces STAT 610/611 as biostatistics Ph.D. requirements.
- Syllabus for BIOSTAT801
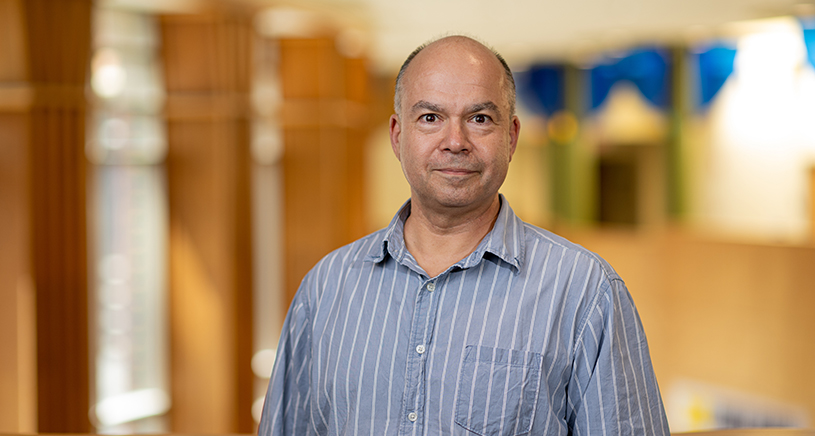
Department | Program | Degree | Competency | Specific course(s) that allow assessment | BIOSTAT | PhD | Apply the advanced probability theory and distribution theory | BIOSTAT801 |
---|
BIOSTAT802: Advanced Inference II
- Graduate level
- Residential
- Winter term(s) for residential students;
- 3 credit hour(s) for residential students;
- Instructor(s): Walter Dempsey (Residential);
- Prerequisites: Biostat 601, Biostat 602, and MATH 451 or equivalent
- Description: This sequence covers advanced topics in probability theory, theory of point estimation, theory of hypothesis testing, and related large sample theory. This sequence replaces STAT 610/611 as biostatistics Ph.D. requirements.
- Syllabus for BIOSTAT802
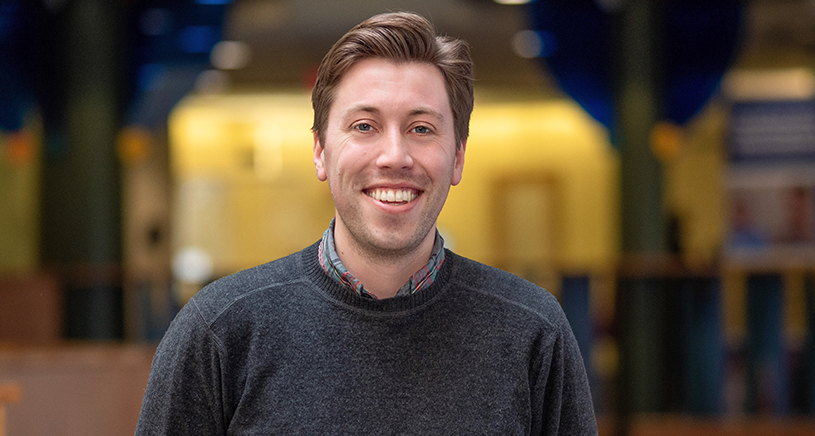
Department | Program | Degree | Competency | Specific course(s) that allow assessment | BIOSTAT | PhD | Derive the advanced theoretical mathematics of statistical inferences | BIOSTAT802 |
---|
BIOSTAT803: Biostatistics in Cancer Seminar
- Graduate level
- Residential
- Fall term(s) for residential students;
- 1 credit hour(s) for residential students;
- Instructor(s): Jeremy Taylor (Residential);
- Prerequisites: Perm. Instr.
- Description: The purpose of this research seminar class is to describe biostatistical research that is occurring in cancer researcher. The course will consist of presentations by speakers describing their research.
- Syllabus for BIOSTAT803
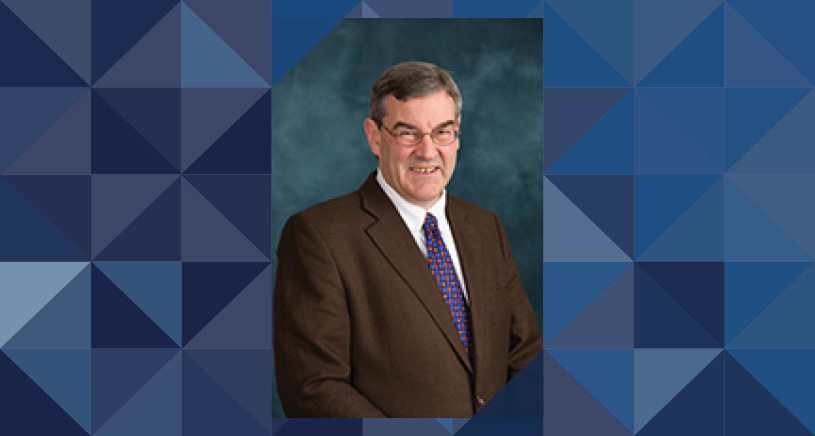
BIOSTAT810: Approaches to the Responsible Practice of Biostatistics
- Graduate level
- Residential
- Fall term(s) for residential students;
- 1 credit hour(s) for residential students;
- Instructor(s): Xiang Zhou (Residential);
- Prerequisites: None
- Description: This course will cover a series of topics that encompass Responsible Conduct of Research and Scholarship (RCRS) as defined by the National Institutes of Health (NIH), as well as focus upon the written and oral communication skills necessary for effective collaboration with public health investigators.
- Syllabus for BIOSTAT810
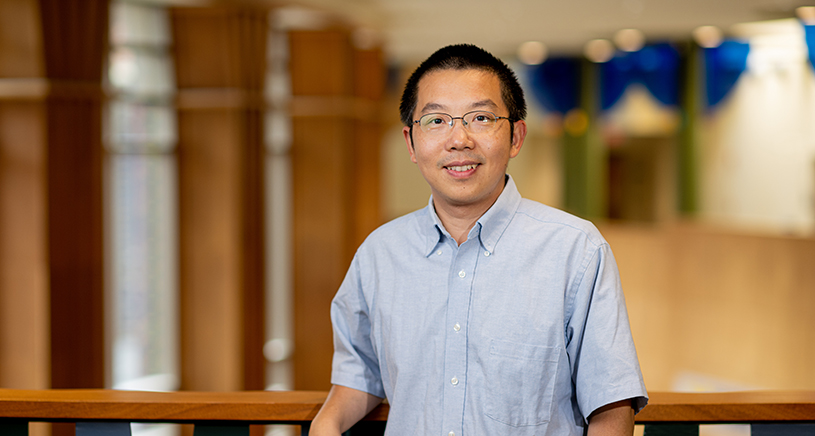
BIOSTAT815: Advanced Topics in Computational Statistics
- Graduate level
- Residential
- Winter term(s) for residential students;
- 3 credit hour(s) for residential students;
- Instructor(s): Michele Peruzzi (Residential);
- Prerequisites: BIOSTAT601, BIOSTAT602 and BIOSTAT615 or equiv and proficiency in C++ and R
- Description: Modern numerical analysis for statisticians. Combination of theory and practical computational examples illustrating the current trends in numerical analysis relevant to probability and statistics. Topics choose from numerical linear algebra, optimization theory, quadrature methods, splines, and Markov chains. Emphasis on newer techniques such as quasi-random methods of integration, the EM algorithm and its variants, and hidden Markov chains. Applications as time permits to areas such as genetic and medical imaging.
- Syllabus for BIOSTAT815
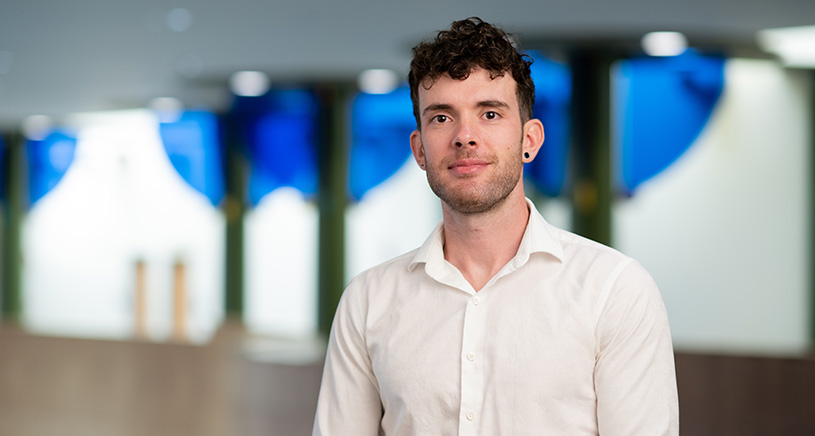
BIOSTAT820: Readings in Biostatistics
- Graduate level
- Residential
- Fall, Winter, Spring-Summer term(s) for residential students;
- 1-4 credit hour(s) for residential students;
- Instructor(s): Staff (Residential);
- Prerequisites: None
- Description: Students assigned special topics for literature study under guidance of individual faculty members. May be elected more than once. Enrollment limited to biostatistics majors.
BIOSTAT830: Advanced Topics in Biostatistics
- Graduate level
- Residential
- Fall term(s) for residential students;
- 1-4 credit hour(s) for residential students;
- Instructor(s): Xu Shi (Residential);
- Prerequisites: course/instructor dependent
- Description: Advanced training in biostatistical methods primarily for doctoral students. Format will include lectures, readings, presentations and discussions in an area of special interest to students and faculty, such as stopping rules and interim analysis in clinical trials, conditional and unconditional inference and ancillarity, or nonparametric regression.
- Syllabus for BIOSTAT830
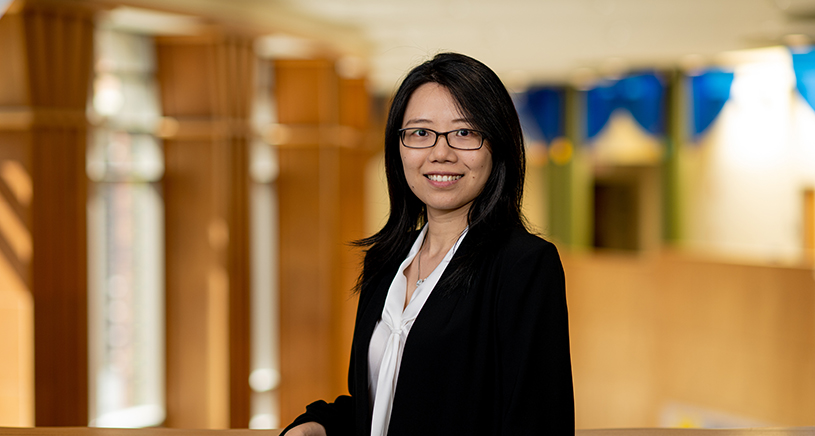
BIOSTAT834: Pedagogical Methods For Biostatistics Courses
- Graduate level
- Residential
- Winter term(s) for residential students;
- 2 credit hour(s) for residential students;
- Instructor(s): Matt Zawistowski (Residential);
- Prerequisites: None
- Advisory Prerequisites: Biostatistics PhD Candidacy or permission of instructor
- Description: Biostatistics faculty teach a wide range of courses over diverse student backgrounds, presenting distinct challenges and considerations. This course will develop ideas and skills for building biostatistics courses tailored for specific student groups and course levels. It will prepare PhD students for their first teaching assignment in a faculty position.
- Learning Objectives: 1. Understand how the audience of a specific course impacts course design, content and learning objectives. 2. Implement the concepts of the Guidelines for Assessment and Instruction in Statistics Education (GAISE) College Report into course planning. 3. Become familiar with technology resources to increase active learning techniques (e.g. Poll Everywhere) and improve course management (Canvas). 4. Develop teaching skills that transcend biostatistics: inclusive teaching practices, mentoring and conflict resolution.
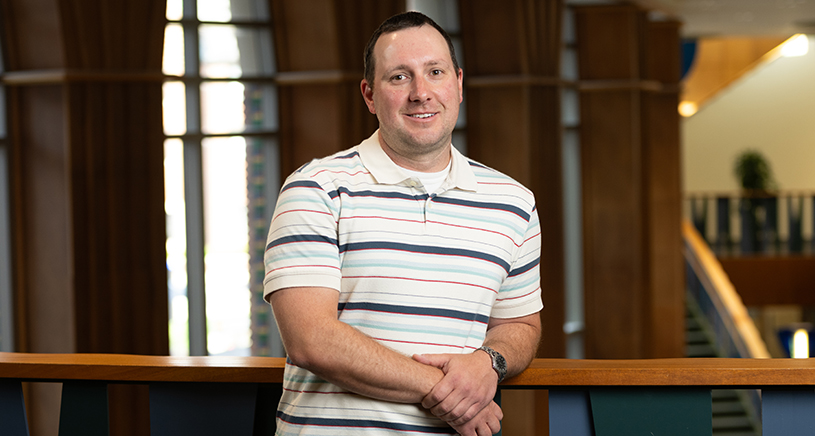
BIOSTAT840: Advanced Topics in Data Analysis
- Graduate level
- Residential
- Fall, Winter, Spring-Summer term(s) for residential students;
- 3 credit hour(s) for residential students;
- Instructor(s): Staff (Residential);
- Not offered 2024-2025
- Prerequisites: Biostat 650 and Biostat 651
- Description: Alternate methods of data analysis useful when data do not fulfill unusual assumptions of statistical tests. Using articles from the literature, students learn methods of data analysis more robust than usual methods and how to choose among them. Focuses on comparison of groups, ANOVA and regression.
BIOSTAT842: Seminal Ideas and Controversies in Statistics
- Graduate level
- Residential
- Fall term(s) for residential students;
- 3 credit hour(s) for residential students;
- Instructor(s): Roderick Little (Residential);
- Prerequisites: Ph.D. students in Biostatistics, Statistics or related field (e.g. Survey Methodology)
- Advisory Prerequisites: None
- Description: Statistics has developed as a field through seminal papers and fascinating controversies. Seminal ideas and controversies in statistics will be reviewed and discussed. Students will be assigned to present and discuss key papers, with the aid of later commentaries in the literature that help elucidate the issues. The goal is to expand student's knowledge of the statistics literature and encourage a historical perspective. A draft list of papers, arranged below by topic, is provided; in additional to original papers there are some more recent commentaries that provides a modern perspective. Topics are arranged in three groupings: (a) philosophy of statistics; (b) seminal problems in statistical analysis (c) design topics, focusing on the role of randomization. The instructor will also present summaries of the topics covered. Students will be assigned homework with a few basic discussion questions about the assigned paper or papers. Also, one "lead presenter" student or students will prepare and deliver a presentation summarizing each topic and paper(s). For the class to work it is essential that students read the assigned material, participate in class discussions, and express their own opinions on the homework questions - often there is not a "right" answer.
- Learning Objectives: After completing this class, students are expected to be able to attain the following competencies: (a) Demonstrate effective written, oral and thinking skills Biostatistics Competencies: (1) To learn some key ideas and concepts in statistics concerning philosophy of inference, statistical methods and statistical design, through seminal articles (b) To learn how to read a research paper and understand the key concepts (c) To learn how to develop clear and logical written and oral presentations based on reading seminal articles in statistics (c) To start to develop a personal philosophy for statistical practice
- Syllabus for BIOSTAT842
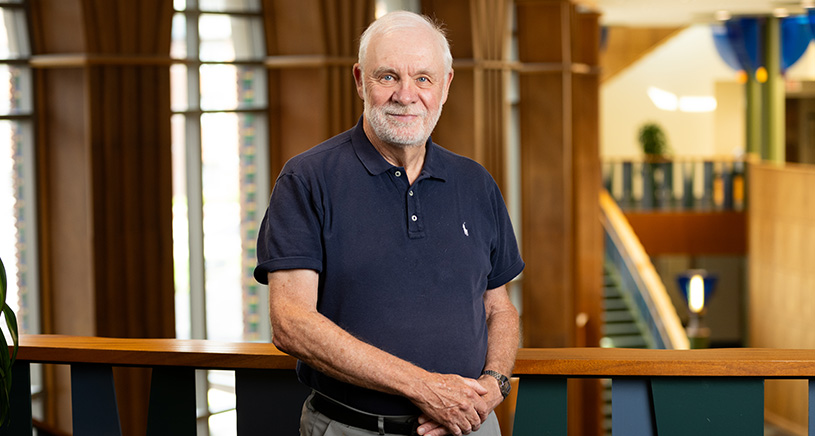
BIOSTAT845: Advanced Topics in Times Series Analysis
- Graduate level
- Residential
- Winter term(s) for residential students;
- 3 credit hour(s) for residential students;
- Instructor(s): Staff (Residential);
- Not offered 2024-2025
- Prerequisites: Biostat 645, Stat 531 or Perm. Instr.
- Description: Advanced theory of stationary univariate and multivariate time series. Additional advanced topics such as analysis of non-stationary, non-linear, and/or categorical time series; time-frequency analysis; and statistical methods based on the wavelet transform or related transforms. Application of methods to time series data sets from health research.
BIOSTAT851: Linear Statistical Models (Stat 642)
- Graduate level
- Residential
- Fall term(s) for residential students;
- 3 credit hour(s) for residential students;
- Instructor(s): Staff (Residential);
- Not offered 2024-2025
- Prerequisites: Biostat 602 and Biostat 651 or Perm. Instr.
- Description: Theory of multivariate normal distribution, distribution of quadratic forms, Cochran's theorem, Gauss-Markov theorem, general linear hypothesis, experimental design models, Wishart distribution.
BIOSTAT865: Advanced Statistical Population Genetics
- Graduate level
- Residential
- Winter term(s) for residential students;
- 3 credit hour(s) for residential students;
- Instructor(s): Sebastian Zoellner (Residential);
- Not offered 2024-2025
- Prerequisites: None
- Description: It is an exciting time for research in population genetics. Technological advances are making it increasingly possible to obtain large numbers of genotypes from individuals in a population, and theoretical and algorithmic advances are improving the prospects for obtaining detailed inferences about populations and their evolutionary history. To make use of these dramatic advances in the field, it is important to understand the processes that act on populations and affect the properties of the genotypes that will eventually be drawn from these populations. In this course, by learning the mathematical models used in population genetics, students will learn how various population-genetic phenomena influence the properties of genetic variation. Students will also gain an understanding of the statistical methods used for analysis of population-genetic data. The course is split into two major sections. The first section covers classical population genetics, including subjects first introduced by RA Fisher and S Wright. We cover Hardy-Weinberg equilibrium, natural selection in infinite and finite populations, stochastic effects in finite populations (drift), recombination and linkage disequilibrium, and admixture and population subdivision. Moreover, we cover the most commonly used models of mutation, such as the infinite sites model and the infinite alleles model. The goal of this section is to give students a broad understanding of the statistical principles underlying population genetics and to provide a connection between these classical results and modern challenges in statistical genetics. In the second section of the course we cover coalescent theory. We introduce the basic coalescent model for constant Wright-Fisher populations. We then introduce commonly used extensions of this model to scenarios with recombination, population expansion and population subdivision. We introduce methods of parameter inference based on these models, including both simple method-of-moments estimates as well as more sophisticated Monte-Carlo based estimation methods. The goal of this section is to give students the ability to design realistic simulation algorithms and perform population genetic inference. Classes on population structure and population admixture (~4) will be taught by Noah Rosenberg. In the biweekly homeworks, we expect the students to be able to apply and extend the presented theory. Early in the course, each student will select a topic for a project; the student is expected to work on this project throughout the semester and to give at the end of the semester a written project report and a 20-minute presentation on the results of his analysis. Typical projects are " Simulate a model of rare variants under mutation-selection balance and estimate power for rare variants testing methods. " Calculate the contribution of low frequency variants to heritability in structured populations " Perform a principal components analysis on genetic data " Explore recent resequencing data for signs of natural selection.
- Learning Objectives: See course description
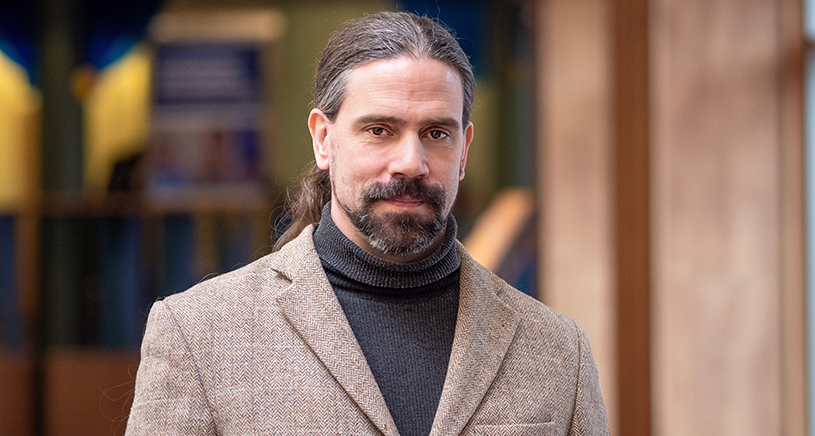
BIOSTAT866: Advanced Topics in Genetic Modeling
- Graduate level
- Residential
- Winter term(s) for residential students;
- 3 credit hour(s) for residential students;
- Instructor(s): Hyun Min Kang (Residential);
- Prerequisites: Biostat 601, Biostat 602, Biostat 666 or Perm. Instr.
- Description: Advanced topics in quantitative genetics with emphasis on models for gene mapping, pedigree analysis, reconstruction of evolutionary trees, and molecular genetics experiments, computational mathematics, and statistical techniques such as Chen-Stein Poisson approximations, hidden Markov chains, and the EM algorithm introduced as needed.
- Syllabus for BIOSTAT866
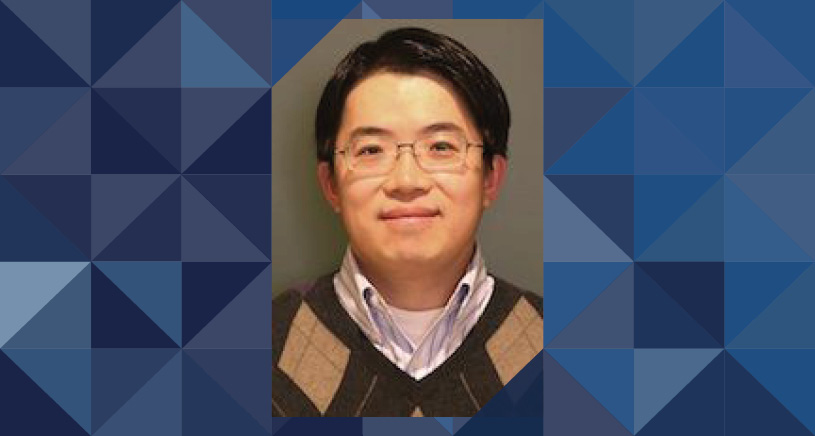
BIOSTAT870: Analysis of Repeated Measurements
- Graduate level
- Residential
- Winter term(s) for residential students;
- 3 credit hour(s) for residential students;
- Instructor(s): Peisong Han (Residential);
- Not offered 2024-2025
- Prerequisites: Math 417, Biostat 602, Biostat 651 and one of Biostat 690, Biostat 851, or Biostat 890
- Description: Mixed model analysis of variance; multivariate profile analysis; linear mixed effects models with unbalanced designs, time-varying covariates, and structured covariance matrices; maximum likelihood (ML), restricted maximum likelihood (REML), and Bayes estimation and inference; nonlinear mixed effects models.
- Syllabus for BIOSTAT870
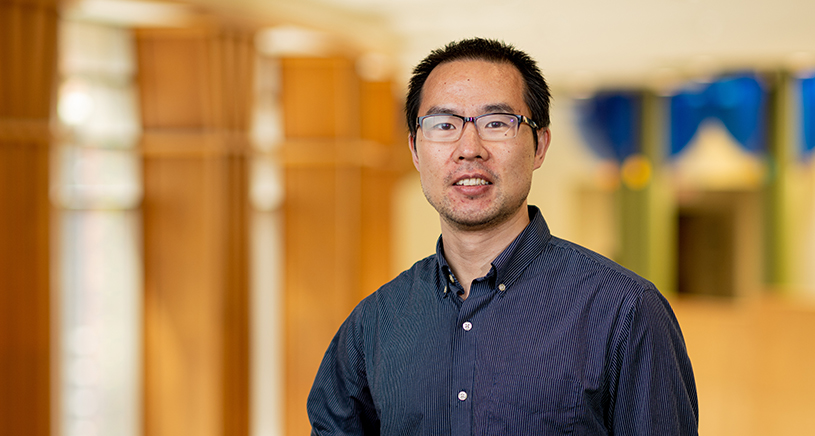
BIOSTAT875: Advanced Topics in Survival Analysis
- Graduate level
- Residential
- Winter term(s) for residential students;
- 3 credit hour(s) for residential students;
- Instructor(s): Susan Murray (Residential);
- Not offered 2024-2025
- Prerequisites: Biostat 675
- Description: Lectures and readings from the literature on advanced topics in survival analysis. Covers regression for censored data, general event-history data and models, competing risks. Statistical, mathematical, and probabilistic tools used in survival analysis are extended for these general problems.
- Syllabus for BIOSTAT875
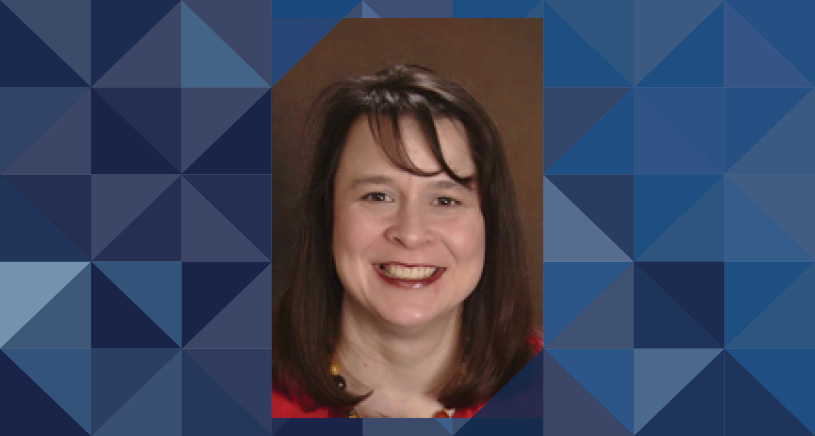
BIOSTAT880: Statistical Analysis With Missing Data
- Graduate level
- Residential
- Fall term(s) for residential students;
- 3 credit hour(s) for residential students;
- Instructor(s): Donglin Zeng (Residential);
- Prerequisites: Biostat 602 and 651, and at least one of Biostat 690, Biostat 851, Biostat 890, or Biostat 895 or Perm Inst.
- Description: Statistical analysis of data sets with missing values. Pros and cons of standard methods such as complete-case analysis, imputation. Likelihood-based inference for common statistical problems, including regression, repeated-measures analysis, and contingency table analysis. Stochastic censoring models for nonrandom nonresponse. Computational tools include the EM algorithm, the Gibbs' sampler, and multiple imputation.
- Syllabus for BIOSTAT880
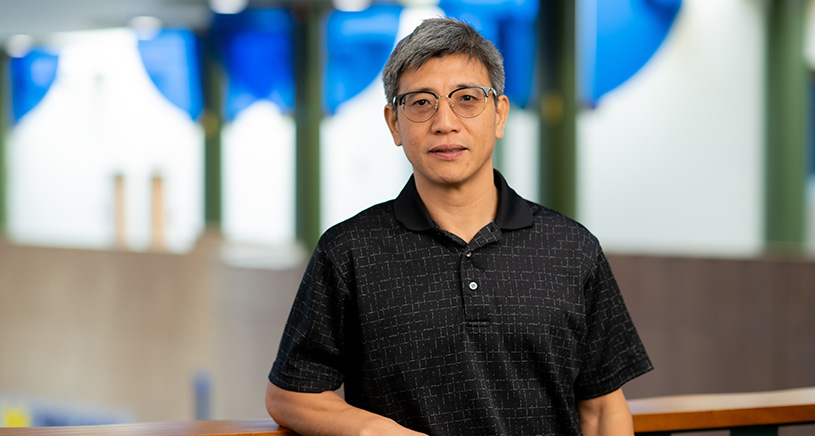
BIOSTAT881: Topics In Advanced Causal Inference
- Graduate level
- Residential
- Winter term(s) for residential students;
- 3 credit hour(s) for residential students;
- Instructor(s): Jean Morrison (Residential);
- Prerequisites: BIOSTAT 601, BIOSTAT 602, BIOSTAT 650, BIOSTAT 651, BIOSTAT 653, BIOSTAT 801, and BIOSTAT 802 (concurrent also accepted).
- Description: This course covers statistical theory and methodology for drawing causal conclusions from observational and experimental data. We will cover theoretical foundations including DAGs and SEMs, followed by special topics, which may include instrumental variable analysis, causal inference in high dimensions, and causal inference with longitudinal data.
- Learning Objectives: At the end of the course the students will be able to: 1. Translate a scientific question into a causal contrast to be estimated. 2. Derive graphical models for investigating the conditions under which the causal contrasts of interest are identified from data collected under specific study designs. 3. Formulate adequate structural models for making inference about the causal contrasts of interest. 4. Implement simulations appropriate for investigating the properties of causal estimators.
- Syllabus for BIOSTAT881
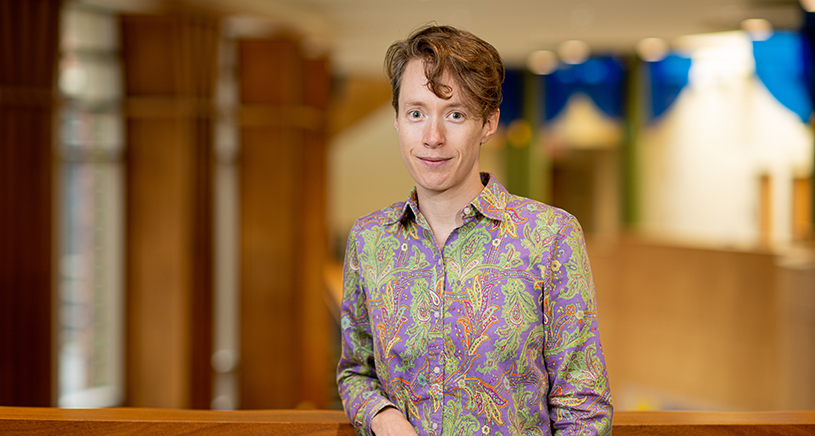
BIOSTAT882: Advanced Bayesian Inference
- Graduate level
- Residential
- Winter term(s) for residential students;
- 3 credit hour(s) for residential students;
- Instructor(s): Timothy Johnson (Residential);
- Prerequisites: N/A
- Advisory Prerequisites: Biostatistics 682 or an equivalent course covering the basic Bayesian methods and theory. Previous experience in programming in R or C/C++ is required.
- Description: This course focuses on advanced Bayesian theory and nonparametric Bayes methods including Gaussian processes, Dirichlet processes, deep neural networks, variable selection, and shrinkage priors, along with modern posterior computation algorithms including gradient based Markov chain Monte Carlo and variational Bayesian methods.
- Learning Objectives: This course focuses on the advanced Bayesian inference methods including modeling, theory and computation. The target audience is the PhD candidates in Biostatistics who are interested in working on their research topics related to Bayesian statistics. R and C++ will be used for illustrations and practices.
- Syllabus for BIOSTAT882
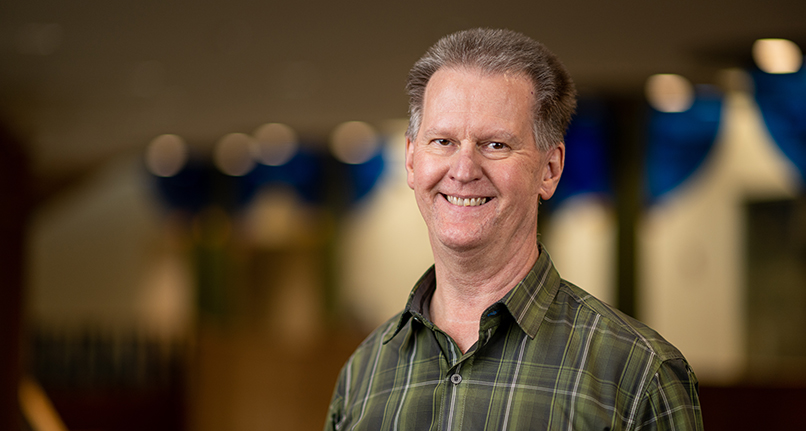
BIOSTAT885: Nonparametric Statistics
- Graduate level
- Residential
- Fall term(s) for residential students;
- 3 credit hour(s) for residential students;
- Instructor(s): Peter Xuekun Song (Residential);
- Prerequisites: Biostat 601/602 or Perm. Instr.
- Description: Theory and techniques of nonparametrics and robustness. M-estimation, influence function, bootstrap, jackknife, generalized additive models, smoothing techniques, penalty functions, projection pursuit, CART.
- Syllabus for BIOSTAT885
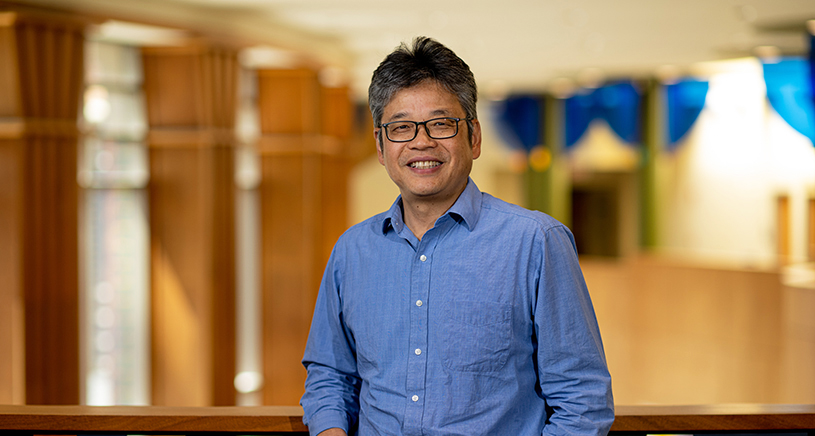
BIOSTAT890: Multivariate Statistical Models (Stat 640)
- Graduate level
- Residential
- Winter term(s) for residential students;
- 3 credit hour(s) for residential students;
- Instructor(s): Staff (Residential);
- Not offered 2024-2025
- Prerequisites: Math 417 and either Stat 511 or Biostat 602 and Perm. Instr.
- Description: Derivation of multivariate techniques: multivariate estimation, T, criteria for testing linear hypothesis, test for additional information, testing covariance matrices, factor analysis, growth curves and elementary time series.
- Syllabus for BIOSTAT890
BIOSTAT895: Analysis of Multivariate Categorical Data
- Graduate level
- Residential
- Fall term(s) for residential students;
- 3 credit hour(s) for residential students;
- Instructor(s): Peter Xuekun Song (Residential);
- Not offered 2024-2025
- Prerequisites: Biostat 651 and Biostat 695 or Perm. Instr.
- Description: Probability models for two-way tables; multi-factor, multi-response framework; product multinomial distribution theory; Taylor series estimates of variance, weighted least squares and Wald statistics; constraint equations; models for characterizing interactions; step-wise variable selection; factorial designs with multinomial responses; repeated measurement experiments; log-linear models; paired-choice and bioassay experiments; life-table models.
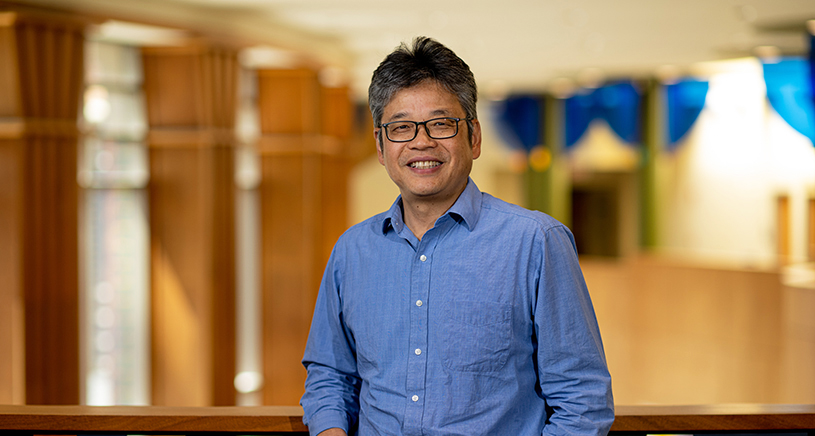
BIOSTAT896: Spatial Statistics
- Graduate level
- Residential
- Winter term(s) for residential students;
- 3 credit hour(s) for residential students;
- Instructor(s): Michele Peruzzi (Residential);
- Prerequisites: BIOSTAT 601, BIOSTAT 602, BIOSTAT 650, BIOSTAT 653
- Description: This course will introduce the theory and methods of spatial and spatio-temporal statistics. It will present spatial and spatio-temporal statistical models and will discuss methods for inference on spatial processes within a geostatistical and a hierarchical Bayesian framework.
- Syllabus for BIOSTAT896
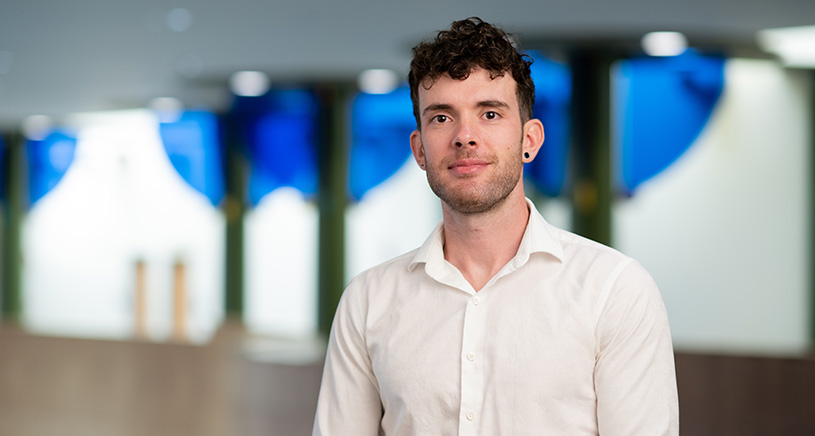
BIOSTAT990: Dissertation/Pre-Candidacy
- Graduate level
- Residential
- Fall, Winter, Spring-Summer term(s) for residential students;
- 1-8 credit hour(s) for residential students;
- Instructor(s): Staff (Residential);
- Prerequisites: (1-8 Full term, 1-4 Half term)
- Description: Election for dissertation work by doctoral student not yet admitted to status as a candidate.
BIOSTAT995: Dissertation Research for Doctorate in Philosophy
- Graduate level
- Residential
- Fall, Winter, Spring-Summer term(s) for residential students;
- 1-8 credit hour(s) for residential students;
- Instructor(s): Staff (Residential);
- Prerequisites: Admission to Doctoral Program(1-8 Full term, 1-4 Half term)
- Description: Election for dissertation work by doctoral student who has been admitted to status as a candidate.