The future is here
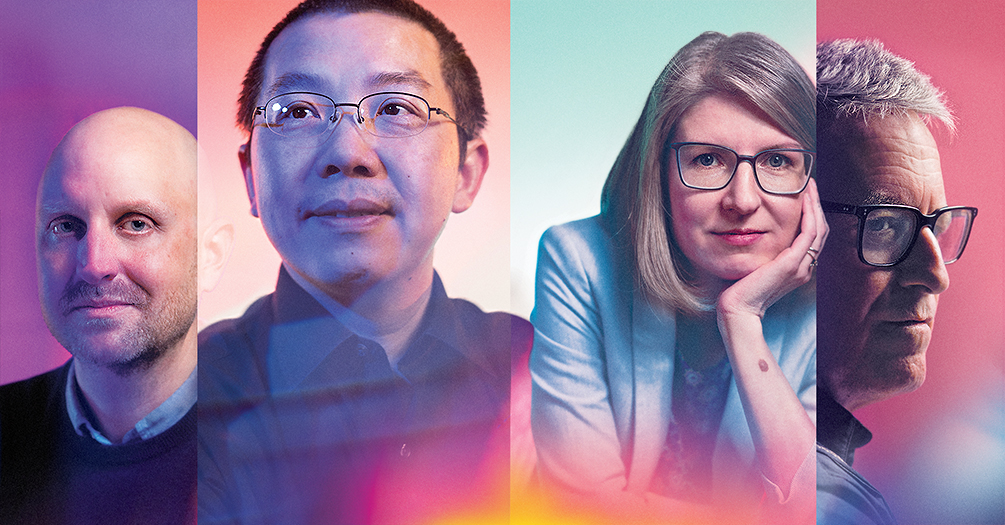
Michigan Public Health is taking bold steps with AI
By Bob Cunningham
Photos by Daryl Marshke
Within the dynamic environment of artificial intelligence (AI), its various forms and applications are being woven into educational and research activities. This integration signals a transformative shift in how we educate the next generation of experts and serve high-priority communities worldwide.
AI is not only enhancing the way knowledge is generated and shared, but it’s also redefining the potential for healthcare and public health advancements. Behind its research might, the University of Michigan School of Public Health leads the charge in this transformative paradigm shift.
Technological advancement, including AI, is a key component of Vision 2034, a 10-year strategic plan announced last year by University of Michigan President Santa J. Ono.
One of the key motivations behind this push is the potential of AI to enhance educational methods and research impact. AI offers a transformative approach to learning by providing dynamic, interactive and personalized educational experiences. This potential extends into research, where AI can dramatically accelerate data analysis and expand the boundaries of traditional methodologies, pioneering new pathways for exploration and discovery.
“We understand that AI is not just a tool of tomorrow—it’s a necessity for today,” said Sharon Kardia, senior associate dean for Education at Michigan Public Health. “With President Ono’s leadership, we’re not just reacting to the changes AI brings, we’re actively shaping them to create more impactful educational and research outcomes.”
INTERESTED IN A DEGREE from Michigan Public Health? Learn more about our programs.
We understand that AI is not just a tool of tomorrow—it’s a necessity for today.”
— Sharon Kardia
This proactive stance underscores the commitment of the University of Michigan to not only incorporate AI technology into our work, but to do so responsibly and ethically, ensuring it serves the broader goals of societal advancement and knowledge dissemination.
A pivotal move has been the creation of a suite of generative AI tools, including U-M GPT. This adaptation means that the university community can explore and master AI technologies safely within a controlled environment, protecting research work and data from external risks while fostering rapid, creative and analytical growth.
“It’s like having a password-protected digital workspace,” Kardia said. “We can learn more safely and efficiently, and be better equipped to serve our staff, students and the future workforce.”
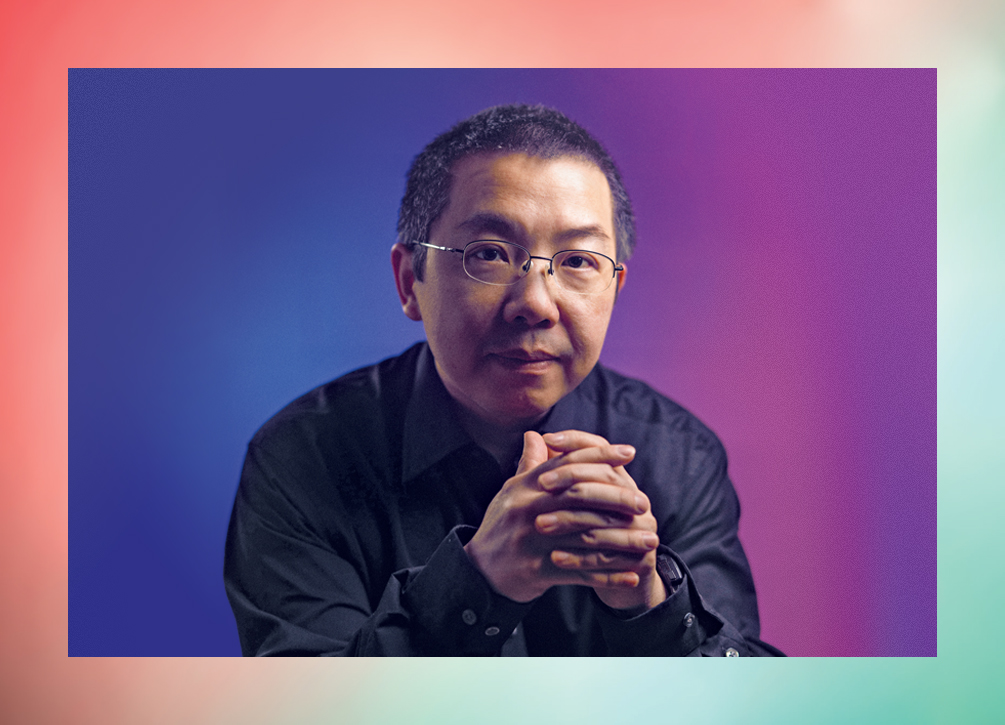
Advancing genomics with AI
Xiang Zhou, professor of Biostatistics and assistant director of Data Solutions for AI & Digital Health Innovation at the university, leads the charge at Michigan Public Health, leveraging AI to enhance genetic and genomic research.
“The appearance of ChatGPT really opened our eyes,” said Zhou, whose work reflects a broader institutional effort to embrace AI. “We saw an opportunity with AI to drastically improve health outcomes.”
With more than half of his current research projects focused on AI, Zhou uses machine learning algorithms to analyze large-scale genomic data. His aim is to decode complex genetic patterns, hoping to uncover disease mechanisms and potentially identify cures.
One significant development is Zhou’s pilot AI project within the Michigan Genomics Initiative, a vast cohort of more than 100,000 individuals. The initiative gathers detailed data such as genetic information, electronic health records and data from wearable devices. Zhou’s project employs AI to extract useful phenotypic information from complex data sets such as text-based clinical notes, which traditionally have been challenging to manage and analyze.
“AI tools can identify which parts of DNA are associated with diseases, like type 1 diabetes,” Zhou said, highlighting how his research seeks to untangle the genetic origins of diseases. “If we understand these associations, we can develop better treatments and potentially discover new drug targets.”
READ MORE about faculty, students, alumni and staff.
Our goal is to make health discoveries accessible to underrepresented groups. With AI, we’re developing methods that can benefit all populations, regardless of ancestry.”
— Xiang Zhou
AI also aids in promoting health equity, a core mission of Michigan Public Health. By using machine learning algorithms, Zhou and his team strive to create fairer algorithms that consider diverse populations.
“Our goal is to make health discoveries accessible to underrepresented groups,” Zhou said. “With AI, we’re developing methods that can benefit all populations, regardless of ancestry.”
Despite these advances, Zhou is cautious about AI’s limitations. While the technology offers powerful tools for discovery, it is not foolproof. Probabilistic modeling and statistical thinking remain crucial to safeguard against AI’s pitfalls, such as inaccurate predictions or “made-up” answers when AI’s knowledge base is limited—an AI phenomenon called “hallucination.”
Zhou advocates for more resources, particularly graphics processing units (GPUs), which are essential for running advanced AI algorithms. Highlighting the long wait times and high costs to acquire GPUs, he said that increased investment in computational infrastructure is necessary for continued progress.
“Having the support from the top really inspires us and makes what we do possible,” Zhou said.
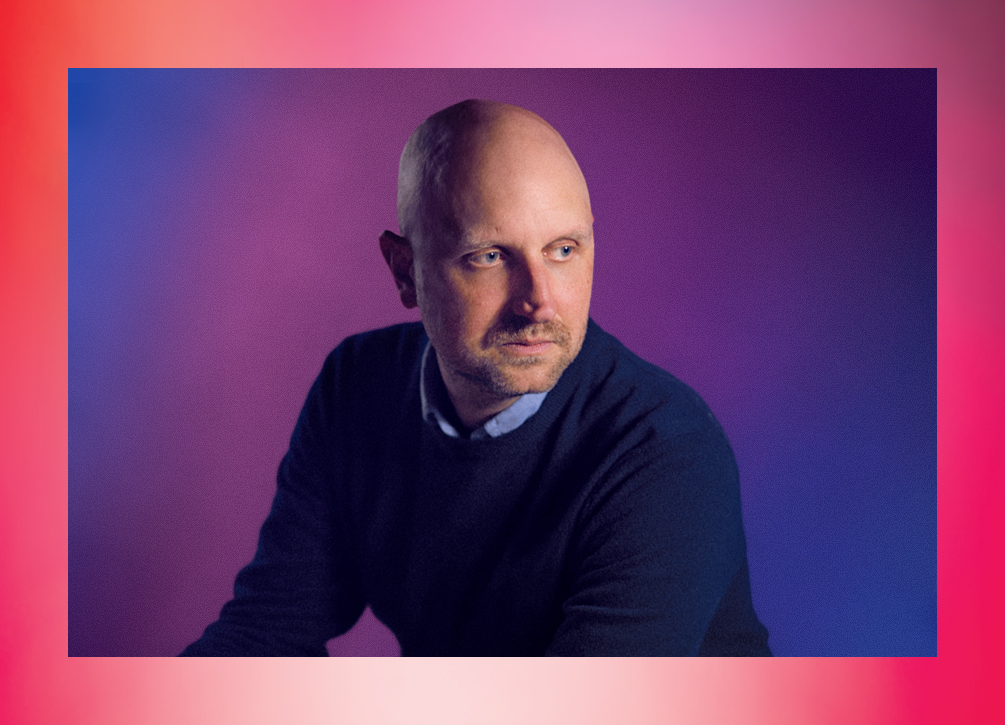
Revolutionizing environmental health
Specialized uses of AI are also emerging at Michigan Public Health.
Justin Colacino, associate professor of Environmental Health Sciences and Nutritional Sciences, is deploying AI to tackle some of the most pressing environmental health challenges of our time. His innovative use of machine vision to analyze cellular responses to pollutants is illuminating new pathways for public health and environmental safety.
Colacino’s lab focuses on how pollution contributes to diseases such as cancer and Alzheimer’s.
“There’s so much we don’t know about the chemicals we’re exposed to, and AI lets us screen these compounds efficiently,” he said.
Using a sophisticated setup of robotic microscopes and high-content imaging, Colacino can quickly assess how different chemicals affect cell behavior.
High-content imaging is a transformative tool in Colacino’s lab, allowing for rapid screening of chemical exposure effects on cells.
“We expose cells to various compounds and use fluorescent markers to visualize cellular changes,” he said.
The AI system processes thousands of images, recognizes cellular patterns, and predicts how specific chemicals might impact human health—all at speeds unattainable by manual analysis.
However, while Colacino celebrates AI’s remarkable capabilities, he also voices concerns about its environmental footprint. He stressed the need for sustainability in AI, given its substantial energy demands.
SUPPORT research and engaged learning at Michigan Public Health.
There’s so much we don’t know about the chemicals we’re exposed to, and AI lets us screen these compounds efficiently.”
— Justin Colacino
“If U-M is going full bore on AI, I hope we can commit to using renewable energy sources,” he said.
Beyond its environmental applications, Colacino explores AI’s potential in drug development. Collaborating with Jonathan Sexton, a pharmacologist at Michigan Medicine, whose research uses similar AI-driven methodologies to discover new drugs for diseases such as diabetes and COVID-19, Colacino’s team is exploring ways to reduce pharmaceutical waste.
“The AI [system we’ve developed] can screen thousands of compounds to pinpoint those with therapeutic potential,” Colacino said.
Colacino’s work demonstrates AI’s transformative potential in public health, enabling faster decision-making and broader reach.
“AI can process data at scales that humans simply can’t match,” he said.
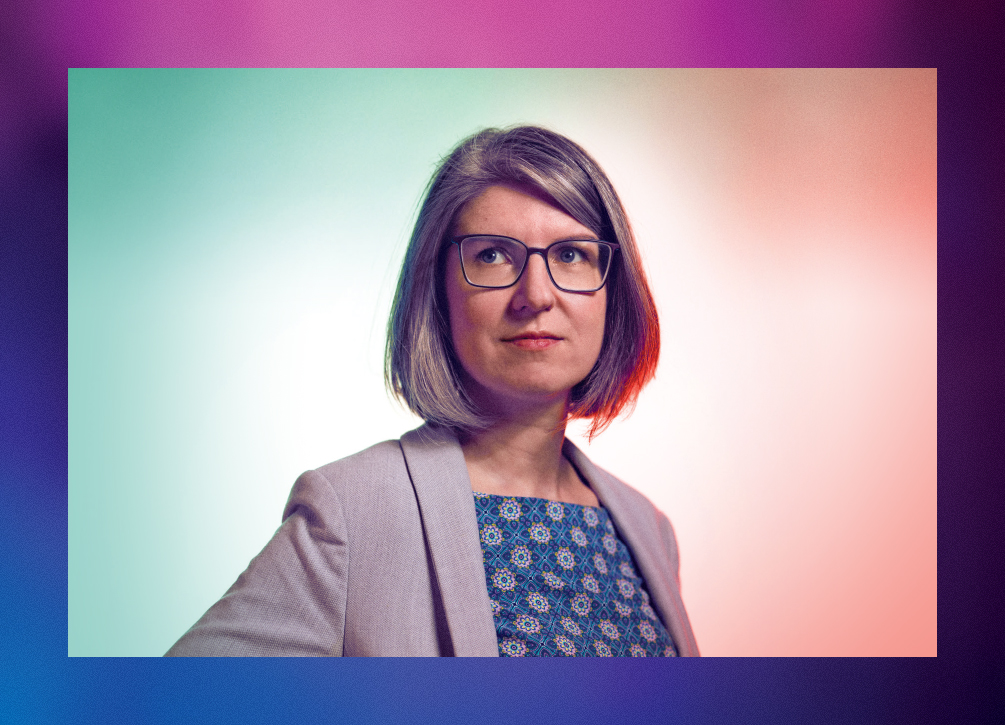
Balancing promise with caution
Irina Gaynanova, associate professor of Biostatistics, explores AI’s capability to transform public health systems by leveraging wearable health data.
Wearable devices such as glucose monitors, heart rate trackers and actigraphy monitors provide invaluable real-time insights into personal health, offering objective monitoring without the need for constant clinical visits. This data-centric approach expedites the diagnostic and intervention processes, potentially impacting a larger population more efficiently.
“These technologies allow us to collect data remotely, reaching people beyond the confines of traditional healthcare settings,” Gaynanova said.
Due to the influx of information generated by these devices, there is a need for innovative, quantitative approaches and models to help healthcare providers identify health trends more swiftly, allocate resources more wisely, and tailor interventions to individual needs.
“This can be very impactful if we can leverage that data effectively,” Gaynanova said.
And AI provides great promise in meeting this goal.
“We can’t ignore the fact that AI is here and that it can be very powerful,” Gaynanova said.
AI is a tool in our toolbox—one that holds promise, but it doesn’t replace the need for comprehensive understanding and training.”
— Irina Gaynanova
Gaynanova’s research leverages AI-based models to predict future glycemic values using data from continuous glucose monitors. She also explores AI-driven methods to uncover latent structures in wearable device data, aiming to enhance their utility and interpretability.
However, her work underscores the promise and pitfalls of deploying AI in health-related research. In exploring AI applications, Gaynanova differentiates between areas where AI excels such as prediction, and others where it falls short: understanding causality.
“AI can predict, but it’s not well-suited to answer what causes what,” she said, emphasizing the limitation of AI’s “black box” nature and the need for interpretability in predictive models—particularly in biomedical domains where understanding causative factors is crucial.
She also raises a cautionary flag about the potential biases and pitfalls that emerge when AI models are trained on unrepresentative data.
Gaynanova stresses the importance of rigorous statistical training and methodological caution in leveraging AI. She argues that AI’s openness and accessibility create the potential for overconfidence, which can lead to dangerous assumptions, especially when applying predictive models across different populations.
To mitigate these risks, Gaynanova advocates for a careful evaluation of training datasets and the use of techniques such as regularization, validation and independent testing to avoid overfitting models.
“It’s crucial to consider who you are training on and ensure that predictions are robust and reliable,” she said.
Her commitment to these principles reflects a broader concern for ethical AI deployment in public health.
Despite AI’s vast potential, Gaynanova remains prudent in her assessment.
“AI is a tool in our toolbox—one that holds promise, but it doesn’t replace the need for comprehensive understanding and training,” she said.
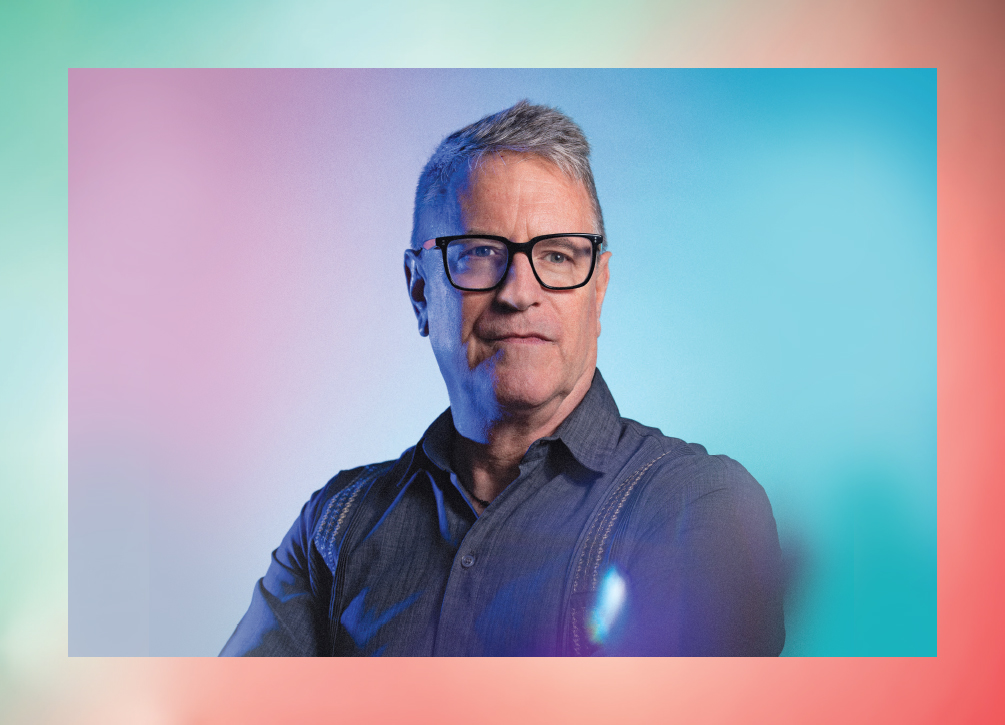
Transforming resource allocation and equity
John Piette, professor emeritus of Health Behavior & Health Equity, is enthusiastic about AI’s potential to transform healthcare delivery, especially in resource-constrained settings.
His focus is on optimizing healthcare resources to improve outcomes without unnecessary burdens of time and cost. This entails using AI to target interventions more precisely, thereby maximizing the impact of available healthcare services and resources.
“In chronic pain management and psychotherapy, we’ve shown that AI can decide who needs to talk to a therapist each week, which can cut clinician hours in half while achieving the same outcomes,” Piette said. “In the end, that could mean we can serve twice as many patients with the same amount of resources.”
AI-driven models assess patients’ changing needs and treatment response, allowing for tailor-fit healthcare solutions. Such an approach holds promise for extending quality healthcare to underserved areas, such as rural regions in the United States and developing countries like Honduras, where Piette is actively involved in collaborative projects with governmental and community partners.
A cornerstone of Piette’s initiatives is employing AI to tackle resource scarcity, a significant barrier in healthcare. By using AI algorithms to determine the necessity of clinician intervention, Piette’s solution not only saves time, but also allows for reallocation of resources to those in dire need.
This is particularly critical in Piette’s international work, where healthcare systems often have far fewer resources and even greater patient demand than in the US.
“Our AI approach is figuring out who really needs a conversation this week and who can reach their goals with a lighter-touch approach to self-care support,” Piette said.
Despite AI’s potential, there are significant challenges concerning its implementation, particularly around equity and fairness.
There’s a real concern that AI could lead to biased decisions that don’t reflect healthcare goals or community values. Our job is to recognize and address these biases, to ensure that AI doesn’t harm but enhances care.”
— John Piette
Piette is acutely aware of the dangers AI can pose if not properly implemented. He points out that AI systems, if not carefully managed, can exacerbate existing disparities in healthcare. AI models might inadvertently utilize biased data, leading to decisions that could deepen existing inequalities or even create new ones.
“There’s a real concern that AI could lead to biased decisions that don’t reflect healthcare goals or community values,” Piette said.
For instance, if AI systems are trained predominantly on data from privileged groups, their recommendations for marginalized communities may be flawed, potentially resulting in subpar care for those who might need it most.
Piette’s experiences underpin the necessity of rigorous AI oversight and training to ensure algorithms do not inadvertently create discriminatory outcomes. By integrating stringent evaluation processes and employing diverse datasets, Piette aims to develop AI systems that are both equitable and effective.
Beyond technical challenges, Piette emphasizes a pressing ethical concern: the potential for AI to substitute machine-derived recommendations for human judgment, especially in nuanced medical decisions. This raises critical questions about the boundaries of AI in healthcare and whether it should override clinicians’ expertise.
“Our job is to recognize and address these biases, to ensure that AI doesn’t harm but enhances care,” Piette said.
Ethical AI usage requires continuous assessment and adaptation, for which Piette and his team actively advocate.
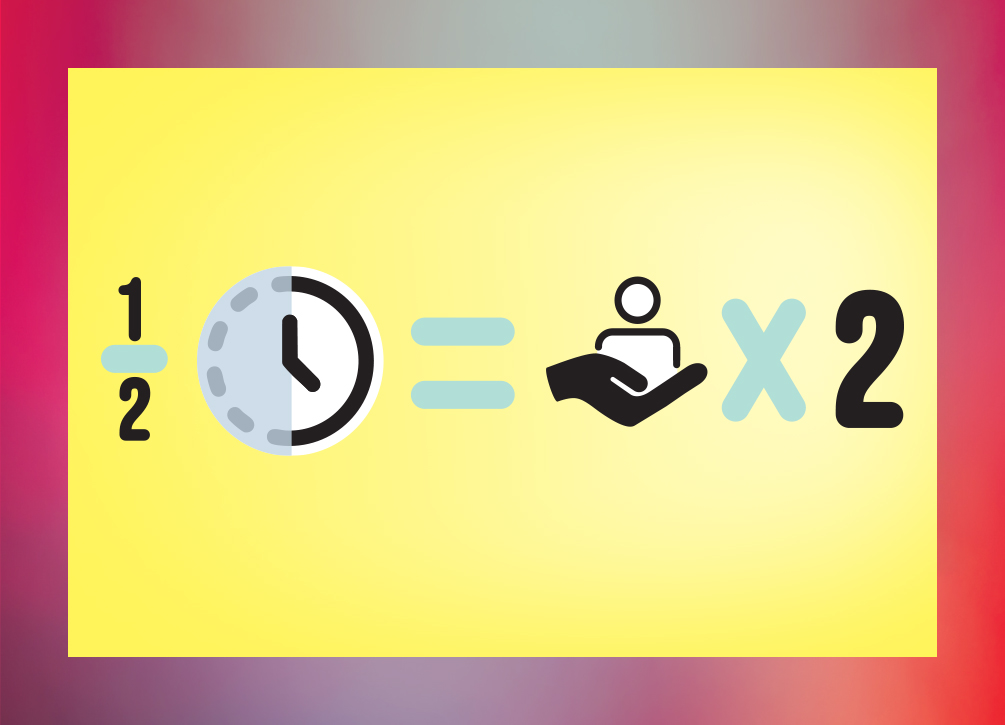
Navigating AI’s future
As Michigan Public Health integrates AI into its core strategies, it stands poised to innovate public health delivery. The potential to accelerate processes, enhance diagnostics and personalize interventions is becoming a tangible reality.
AI’s ability to swiftly process vast healthcare data means that responses can be quicker and more effective, reaching wider populations in less time. However, these advancements must be balanced with ethical considerations and a commitment to equity.
While AI promises enhanced efficiency, it must be deployed mindfully to ensure it complements rather than replaces human expertise. The School of Public Health is leading this nuanced approach, marrying technology while remaining dedicated to ethical practice and health equity.
“As AI propels public health into the future,” Kardia said, “Michigan Public Health continues to be committed to harnessing its full potential for the greater good.”
Read More in This Issue of Findings