The Promises and Challenges of Big Data
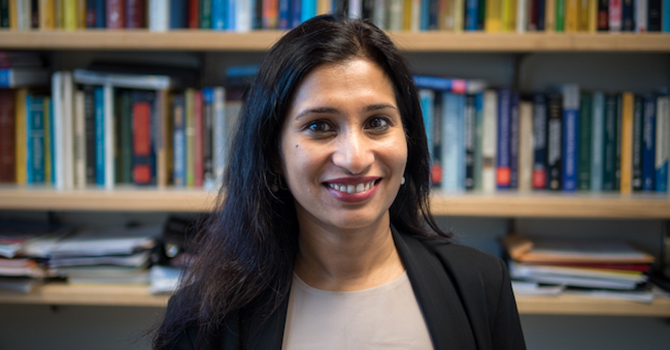
Bhramar Mukherjee
John D. Kalbfleisch Collegiate Professor of Biostatistics; Associate Chair of Biostatistics; Professor of Epidemiology and Global Public Health; Associate Director for Cancer Control and Population Sciences, The University of Michigan Comprehensive Cancer Center
The Promises and Challenges of Big Data
Collecting data from millions of patients and figuring out how to process and use it occupies much of Bhramar Mukherjee's time. The John D. Kalbfleisch Collegiate Professor of Biostatistics explains how modern data resources have shifted away from the traditional population-based method of recruiting patients for studies. "We are getting more and more self-selected, opt-in samples from hospital systems, insurance companies, even data collected from Facebook, Twitter, and other social media," she says. "This leads to vast amounts of patient data coming in at a very fast rate."
But this broader net for bringing in data means the data is often less refined, which introduces its own problems. First, biostatisticians have to account for biased samples and missing data structures. For example, in the recent biorepository effort with Michigan Medicine and the Michigan Genomics Initiative, patients were recruited prior to receiving anesthesia for surgery or interventional diagnostic tests. As you might expect, this sample had an overrepresentation of cancer patients, because cancer patients undergo surgery or invasive tests more often than the general public. And if you have a major condition like cancer, you tend to come to an academic medical center more often. "This gives you a rich collection of cancer cases to work with," says Mukherjee. "But you have to be careful with selection bias, finding spurious results and, of course, missing data."
A careful analysis must account for missing data. "There are periods when we do not see a patient in the database," she says. This can be due to the patient being healthy, or going to another healthcare facility or trying alternative, non-therapeutic treatment. "The times that you see a patient and the frequency at which you see a patient are not random. They usually are related to their health status and results of the tests physicians order. These factors present analytic challenges."
Big Data's Holy Grail
But the scientific community does not give up and, Mukherjee explains, can turn challenge into opportunity. In fact, the role of statistics is more important than ever in dealing with complicated studies. "My strategy has been to take on the imperfect projects with imperfect data and demonstrate the value of the solid statistical thinking and methods," Mukherjee says. "Integration and linkage of various sources of data allows us to combine big databases effectively with relatively small well-characterized designed studies."
The main reason for incomplete data, Mukherjee explains, is that we do not have a fully integrated system of obtaining biomedical data. The medical records at Michigan Medicine may not be linked with pharmacy claims or diagnostic tests done at another facility. "The Holy Grail for precision prevention or treatment," says Mukherjee, "is that we create a large national patient database with information that is broad and deep. A physician would be able to search millions of records to find anonymous patients similar to the one sitting in the exam room and could use that data to provide more accurate care. That's the dream of precision health."
The dream—the Holy Grail—seems agonizingly close some days, part of a distant future on others. The dream in its full form would be a comprehensive archive of patient-level data on multiple domains—health history, socioeconomic data, genes, exposure—from as many people as possible. The data would be "scrubbed"—quantified and disassociated from the individual contributor—for anonymity and confidentiality and would help provide everyone with improved health care. Modern technology seems so advanced, big data so ubiquitous. This large, integrated database could be just around the corner, but at the moment, ''we have access to silos of partial information, heterogeneous snapshots of data. So at the moment, we can solve only parts of the puzzle. But we certainly are moving rapidly toward our idealized target," says Mukherjee hopefully.
There are unexpected challenges with medical records data. "We might be able to access lab values from a patient visit itself, but it takes considerable effort to integrate that with the health history questionnaire—the one you fill out while you're in the waiting area—because of how the databases are structured and data stored," she explains. Another aspect of electronic health records is to extract information from the notes doctors and nurses take to capture a variety of patient interactions using text mining and natural language processing. "The structured and unstructured parts of electronic health-record data contain valuable information about patient characteristics, exposure, and health outcomes, and we are not there yet to have a clean, integrated, usable analytic dataset. But we will get there," Mukherjee says.
Technology and Life-Saving Interventions
While the Arthurian search for a more complete health archive continues, personalized biometric collections can provide remarkably simple interventions for some patients, a glimpse of the power of data in our daily lives.
"In this age of information revolutions, mobile health devices like the FitBit and the AppleWatch really come into play," Mukherjee says. "These tools can give us continuous data on diet, physical activity, and so on." There are addiction studies that used GPS tracking devices to improve the timing and impact of interventions, a technique called "geofencing." For example, in a smoking cessation study, when participants were located near a store that sold cigarettes, they would receive a text message reminding them of their intention to quit. These text messages are often crafted with input from the participants themselves for an effective tailored or personalized prevention.
Precision intervention studies like this show remarkable potential for using mobile technology and tailored communication in behavioral therapy. Changes in patterns of activity, diet, and sleep can predict depression and other mental health diseases, and help can be sought and offered preemptively. "What can seem like an invasive use of technology," says Mukherjee, "actually has great power to help people and save lives."
The Power of Precision Prevention
Even without finding the Holy Grail, precision health can make big impacts relatively quickly. "As population health experts," says Mukherjee, "we don't treat individual patients. We try to create healthier populations. Precision prevention is a powerful tool. If you can reduce the risk of cancer with diet and behavior, it is better for patients, who avoid the trauma of fighting cancer and undergoing invasive therapies. And it is better for the overall community, which is relieved of the expenses and familial burdens associated with treating cancer."
Using data on genes and patient environments, Mukherjee develops customized risk prediction models and targeted prevention strategies for cancer. National or local, comprehensive or personalized, big data interventions offer creative, proven solutions for improved population health. Mukherjee and her peers in biostatistics won't see you in the clinic, but they might just save your life or the life of someone you love.
- Find out more about Biostatistics.
- Interested in public health? Learn more here.