Biostatisticians use machine learning approach to improve risk prediction for recurrent health events
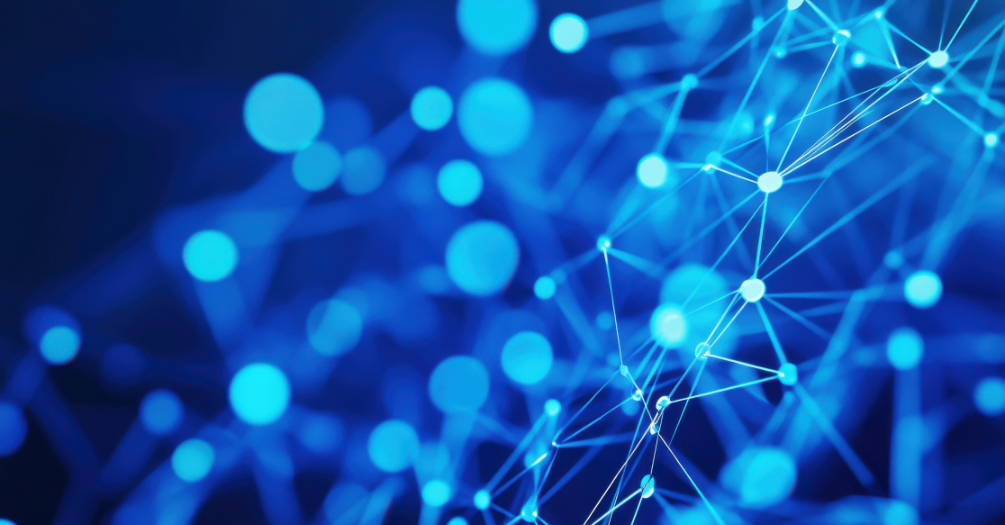
"Random forest" algorithm outperforms traditional methods for predicting patient flare-ups, even with incomplete medical histories
A new machine learning approach developed by University of Michigan School of Public Health researchers better predicts when patients might experience recurring health events like disease flare-ups or hospitalizations, even when patient follow-up data is incomplete. The method, detailed in the Biostatistics paper "Random forest for dynamic risk prediction of recurrent events: a pseudo-observation approach," shows how machine learning can be another valuable tool in the public health toolbox, addressing challenges that traditional statistical methods struggle with. This approach, called "random forest," can identify patterns that are predictive of recurring events of interest using large datasets from genetics, electronic health records, and other sources—providing insights that were previously hard to discover.
"Medical data today comes from countless sources and contains complex dynamic relationships that traditional methods simply can't capture effectively," explains Abigail Loe, doctoral student in the Department of Biostatistics at the University of Michigan School of Public Health and first author of the paper. "Our random forest algorithm gives healthcare providers a more powerful tool to predict which patients might experience recurring health problems and when, allowing for earlier and more targeted interventions."
The research team tested their algorithm, called RFRE.PO (Random Forest for Recurrent Events based on Pseudo-Observations), using data from patients with chronic obstructive pulmonary disease (COPD) who experience recurring flare-ups. The algorithm outperformed other models and approaches, particularly when there was moderate to high correlation between recurrent events in the same patient—a common pattern in COPD where one exacerbation often predicts future ones. The study identified several important predictors of exacerbation risk, including hospitalization history, corticosteroid use, pulmonary function metrics, and various patient-reported symptoms.
RFRE.PO is one of the first machine learning methods to work with recurrent event data to make dynamic risk predictions—a tool the researchers hope could extend beyond COPD to other conditions with recurring events. By applying this machine learning approach to health data, medical teams can make better decisions about patient care, potentially leading to better health outcomes through early intervention and more personalized treatment plans.
“Our approach bridges machine learning and traditional statistics,” says Zhenke Wu, associate professor of Biostatistics at Michigan Public Health and a co-author of the paper. “By transforming traditional data into a format that works with random forest algorithms and incorporating partial patient event histories, we’ve created a method that paves the way for more personalized clinical decision-making.”
The teams says they hope it inspires further research that unites the exciting predictive capabilities of artificial intelligence and machine learning with the robust statistical principles that have long underpinned scientific inquiry,
In addition to Loe and Wu, Susan Murray, professor of Biostatistics at Michigan Public Health, is a co-author of the paper.
Study: “Random forest for dynamic risk prediction of recurrent events: a pseudo-observation approach.” Biostatistics. https://doi.org/10.1093/biostatistics/kxaf007
Media Contact
Destiny Cook
PR and Communications ManagerUniversity of Michigan School of Public Health734-647-8650