What’s a Biobank, and How Can My Health Record Support Research?
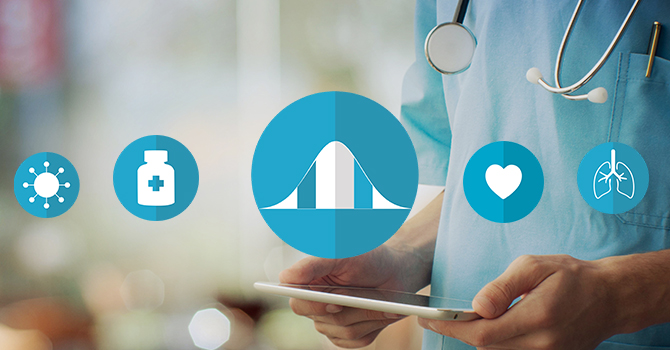
Max Salvatore and Lauren Beesley
Picture it. A patient goes to their provider’s office and is invited to participate in a research study. The patient signs a form and has his or her blood drawn. What happens next?
In the past, samples often were collected for a single study with a specific exposure and outcome in mind. For example, the study may have been looking at blood samples to understand various kinds of blockages in the arteries. Today, blood draws and other patient samples are collected on a much larger scale and stored in massive biobanks, which can then be used by many researchers to study many different outcomes and questions.
Biobanks allow many researchers to study many different outcomes and questions.
With advances in genetic analysis, biobanks like the Michigan Genomics Initiative enroll participants and turn DNA from participants’ blood samples into data. Often, these data are also linked with a patient’s electronic health record (EHR), which provides information about the patient like body measurements, lab results, and diseases they have.
These large, broad datasets can be used to study the connection between genes and diseases, response to drugs and treatments, and other outcomes related to understanding disease. This makes biobank research very promising and exciting, and the availability of so much data can increase the rate at which we can ask and answer crucial health questions.
But there are problems. Data in EHRs (lab values, diagnoses, and so on) are not collected for research purposes.The resources are there, but the methods to draw meaningful conclusions are still very much a work in progress. Due to the increasing research interest in biobanks, the scientific community needs to discuss and address some of the challenges in analyzing and interpreting results using EHR data. In a paper we hope to publish,* we detail many of these challenges.
Accurate Diagnosis
The usefulness of biobank data depends on the accuracy of the diagnosis. A popular approach for deciding whether an individual does or does not have a particular disease involves looking at the insurance diagnosis codes (ICD) recorded in the patient’s EHR. These diagnosis codes, which were developed for patient treatment and billing purposes, are a messy way to measure diseases we want to study. Additionally, the EHR may not capture all of a patient’s diseases. Patients may have only recently moved nearby or may be visiting a particular hospital for specialized care such as surgery. Moreover, diagnosis decisions may vary from doctor to doctor. Researchers are developing methods to account for inaccurate disease diagnosis so they can obtain unbiased results.
Population of Interest
We want to use biobank and EHR data to learn about health in a large, diverse population. To get results that apply to people from different backgrounds, we need to analyze data from an equally diverse group of individuals. However, the pool of patients who provide data to biobanks may not be a good representation of the general population. We have some methods—including statistical approaches—that can make research results as applicable as possible to different people. Ensuring accuracy when data are missing and comparing results across different biobanks also complicate doing good biobank-based research and ensuring our conclusions are valid.
Studies using biobanks can help us better understand disease, which can ultimately improve the health of the community.
In addition to discussing certain challenges to research using biobank data, our paper maps out different types of biobanks around the globe to help researchers better understand biobank data and how to use it. Using data from the Michigan Genomics Initiative (a large EHR-linked biobank enriched with cancer patients), the Genes for Good initiative (an innovative user-initiated biobank through social media), and the world-renowned UK Biobank, we illustrate some of these challenges. We also summarize papers that have been published using biobank data to date and outline some exciting future directions we see for biobank research.
EHRs and biobanks present promising opportunities for the research community. Studies using these large datasets can help us better understand disease, which can ultimately improve the health of the community. With the many challenges to this work, researchers across many disciplines will have to work together in overcoming these barriers so that we can better utilize these valuable data resources.
* At the time of this publication, the paper is being peer-reviewed.
- Discover the Michigan Genomics Initiative, Genes for Good, and Precision Health at Michigan.
Lauren Beesley, PhD, is a postdoctoral research fellow in Biostatistics at the University of Michigan.
She received her PhD in Biostatistics from the University of Michigan in 2018. Her research focuses on
developing statistical methods related to missing data, variable selection, multivariate
survival outcomes, and individualized risk prediction in cancer research.
Maxwell Salvatore is a Research Area Specialist in Biostatistics working with Bhramar
Mukherjee. He earned an MPH in Epidemiology in 2017 and a BA in Economics in 2014, both from the University of
Michigan. His current work involves creating an epidemiologic survey for the Michigan Genomics Initiative. He is passionate about leveraging large, existing data sources, including using
biobank data to stratify individuals for cancer risk and survival.