Beyond the Hype, What Are the Risks and Rewards of Artificial Intelligence in Health Care?
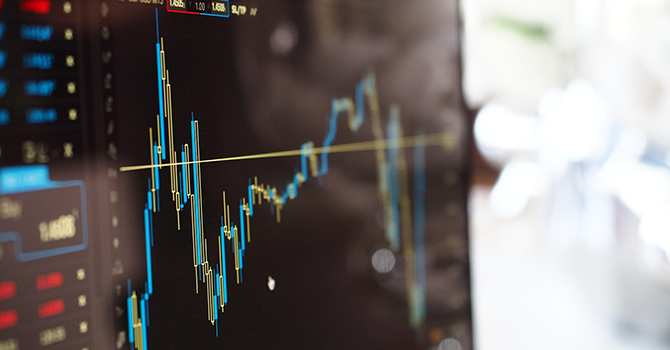
Kasvhi Gupta
Master’s Student in Global Health Epidemiology, Gelman Global Scholar
Artificial Intelligence (AI) has received tremendous attention for its ability to transform how health care uses Electronic Health Records (EHR). What might this look like in clinical settings and what are the risks and rewards of using such powerful technology?
Electronic Health Records (EHR) are already an invaluable tool for organizing a patient’s demographic information and their past and current medical data. EHR can support physician decision-making through notifications on abnormal lab values, drug interactions, and disease outbreaks, and many other factors that can impact a patient’s health. EHR help increase the efficiency of administrative processes like scheduling appointments, tracking surgical cases, and discharging patients. And EHR make clinical data available to researchers for advancing clinical trials and improving health outcomes metrics.
More and more is expected of providers, who are experiencing burnout in greater numbers than ever before.
But the way we currently work with EHR has created a multitude of unintended consequences. Patients are dissatisfied with providers spending increasing time in front of a screen instead of engaging them in conversation during clinical examinations. More and more is expected of providers, who are experiencing burnout in greater numbers than ever before.1 Researchers are surrounded by data that is unusable because it is inconsistently organized across different health systems.
Patients and providers are having difficulty establishing human connections as their brief time together is diluted by layers of regulatory requirements, including feeding data into the EHR system. Meanwhile, the quality of that data constrains researchers whose work could improve the health of individuals and populations.
Is AI the Solution?
Health care providers use an array of data sources to diagnose and manage a patient’s health. From AI being used to predict infection in critical-care patients to helping surgeons objectively measure performance during surgery, the possibilities for using AI in health care are many.2
Machines can be programmed to “learn” as they work through data, but we are long way from consistently accurate predictions.
But AI has its challenges. It is notorious for introducing bias and reporting errors. Data fed into computers and the algorithms used to process that data are only as good as the quality of those data sets and programmers who created the algorithm. Machines can be programmed to “learn” as they work through data, but we are long way from consistently accurate predictions.
The best algorithms are those built to serve a specific outcome of interest using clear inputs that support meaningful conclusions. For now, we must use caution while training machines to support medical decision-making. Once an algorithm has been validated broadly, it has the potential to be an invaluable tool for providing personalized care to thousands or even millions of patients.
Precision Health in Populations and for Individuals
Public health and health care in general are always holding two questions in tension.
- How do we personalize treatment to improve an individual’s health?
- How can we improve health and prevent disease and injury at a population level?
Precision health sets individual and population health together in a direct relationship of increasing quality. Better clinical care of individuals leads to better data and data organization, which leads to better population data, which leads to better individual care.
Along with data collected in clinical settings, data that encompasses social determinants of health must also become a standard part of EHR. Broad personal information would allow healthcare providers to personalize care for patients based a fuller picture of the factors affecting health—the neighborhood a person lives in, educational background, proximity to a health system, full medical history including mental health, drugs they are taking, family history including diseases—all fed into algorithms that would provide personalized information about particular health risks.
Better clinical care leads to better data and data organization, which leads to better population data, which leads to better individual care.
A three-way partnership between AI, provider, and patient holds much promise. Providers will be able to engage with patients, establish human connection, and practice the art of medicine. In the background, AI will be computing and organizing relevant data for the provider to establish diagnoses and provide care.
Beyond the hype around AI, the next critical steps in developing precision health are to understand our role in contributing data to health systems and ensuring machine algorithms are ethical and representative of populations. The more knowledgeable we are about AI, the more effective our interactions will be with our health care providers, who are collecting the very data that will help us and the communities around us get healthy and stay healthy.
References
- Rotenstein LS, Torre M, Ramos MA, et al. Prevalence of Burnout among Physicians: A Systematic Review. JAMA. 2018;320(11):1131–1150. doi:10.1001/jama.2018.12777
- Topol E. High-Performance Medicine: The Convergence of Human and Artificial Intelligence. Nat Med. 2019;25(1):44-56. doi:10.1038/s41591-018-0300-7
ABOUT THE AUTHOR
Dr. Kashvi Gupta graduated from medical school in India and is studying to become
a cardiothoracic surgeon. She is pursuing an MPH in Global Health Epidemiology at Michigan Public Health and is a Gelman Global Scholar.
She is also president of the Students Engaged in Global Health student organization. Her research focuses on the impact of sleep and diet on cardiovascular
outcomes. Read more about Gupta's path to public health at We Are Michigan Public Health.