Opening Up US Will Trigger More COVID-19 Cases, but Disease Models Suggest How to Avoid a Second Peak
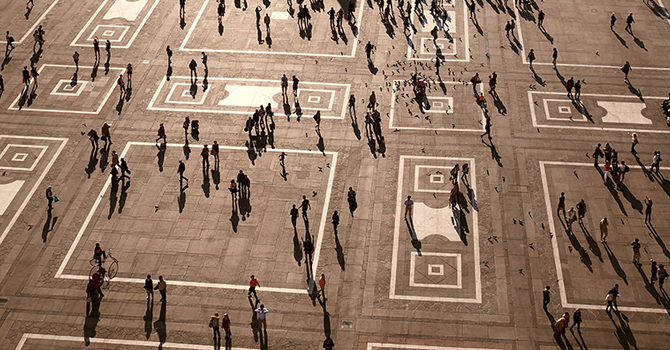
Joseph Eisenberg
Professor and Chair of Epidemiology
This article was originally published on The Conversation.
According to a widely cited forecasting study, relaxed social distancing rules will lead to a two-fold increase in deaths from COVID-19. Predicting how much the mortality will increase, however, is fraught with huge uncertainties, as epidemiologists like me well know. That’s because the forecast relies on assumptions about what will happen in the future.
To make sense of that prediction, then, we must have a clear sense of how the model is constructed, specifically the assumptions used. All forecast models require assumptions to simulate future circumstances, and a key one is how the relaxation of social distancing is characterized. For example, what types of social interactions are changing? Is increased crowding occurring in indoor venues (malls and restaurants) or outdoor venues (the beach)? How quickly is it happening across time? Details like these can dramatically influence mortality forecasts.
The power of epidemiological models is not like a crystal ball, identifying the exact timing of an epidemic. Instead, models are more like a compass, showing the direction of an outbreak within a range of possibilities.
Understanding the degree to which assumptions drive model predictions reveals a reality: The power of epidemiological models is not like a crystal ball, identifying the exact timing of an epidemic. Instead, models are more like a compass, showing the direction of an outbreak within a range of possibilities.
Even without being precise, models are still useful because they offer what military leaders would call boots on the ground. Model results provide insights to guide state and federal strategies and policymaking to control transmission.
Rather than focusing on the two-fold increase in deaths per se, the message to policymakers is that relaxing social distancing too much and too early will result in a significant rise in deaths.
Disease Dynamics
The structure of a model comes with an array of assumptions. Some models are statistical, where mathematical expressions are used to describe the shape of the data. Others are mechanistic, where the mathematical expressions describe the well-understood patterns of disease transmission. Statistical models, like the often-cited study that predicted a two-fold increase in deaths, are adequate for short-term predictions, whereas mechanistic models are preferable for longer-term predictions.
Another strength of mechanistic models is their ability to provide general guidance because the structure of the model reflects a hypothesized disease process. There are assumptions about the rate and intensity of contact, the proportion of those exposed who remain asymptomatic or become symptomatic, and the degree to which those that recover are protected from reinfection, as well as those who were vaccinated.
Since many states have passed their peak, people are now interested in understanding how decisions about social distancing will affect the shape of the epidemic curve. The goal is to decrease the probability of a resurgence in cases and avoid overwhelming the hospital system. At the same time, society cannot sustain high levels of social distancing indefinitely.
The Centers for Disease Control and Prevention has compiled a number of predictions based on different group models to forecast deaths, cases, and projections in the weeks ahead. Source: CDC
This leads to a number of pressing questions: How can the country keep daily cases low to minimize the chances of a resurgence? When might the US expect to see benefits from large portions of the population becoming immune? Mechanistic models can help answer these questions.
Mechanistic models show that by relaxing social distancing too quickly, the US is at high risk of a second peak. This is because we have not built sufficient population immunity. Models can guide society on how to prevent this second peak by balancing slow relaxation of social distancing while implementing more efficient and targeted interventions through testing, contact tracing, and isolation.
Models can also reinforce the need to relax social distancing slowly. It takes time to observe the effect of an intervention, such as imposing stay-at-home orders, and the basic principles of the progression from infection to onset of symptoms to full-blown disease is understood and codified in the model. Therefore, we can use the model to estimate the delay between the onset of an infection and when a case is identified through testing.
Predicting Herd Immunity
Likewise, these models describe herd immunity, the level of population immunity that results in elimination of transmission. Simple models suggest that to attain herd immunity, society will likely need upward of 60% of the population to have protection from reinfection. This is concerning because recent data have suggested that to date only 10-20% of the population has been exposed in some regions.
These models suggest that we must make changes slowly and relax social distancing measures incrementally.
However, this 60% number is predicated on people interacting more than they actually are in a more homogeneous way. Mechanistic models can account for heterogeneity in contact between people which is closer to our reality right now. It turns out when you account for the increased heterogeneity, then the herd immunity threshold is lower than 60%. Mechanistic models can also show that even prior to attaining herd immunity, increasing population immunity can slow transmission.
Moving forward, instead of focusing on precise forecasts, the US should use mechanistic models to help strategically plan. These models suggest that we must make changes slowly and relax social distancing measures incrementally. Using this approach, transmission will continue at a low level and the country will slowly build population immunity, while minimizing the chances of a resurgence and thereby minimizing mortality.
About the Author
Joseph Eisenberg is the John G. Searle endowed Chair and Professor of Epidemiology and Professor of Global Public Health at the University of Michigan School of Public Health. Eisenberg studies infectious disease epidemiology with a focus on waterborne and vectorborne diseases. His broad research interests integrate theoretical work in developing disease transmission models and empirical work in designing and conducting epidemiology studies. He is especially interested in the environmental determinants of infectious diseases.
- Interested in public health? Learn more today.
- Read more articles about Epidemiology alumni, faculty, students, and staff.
- Support research at Michigan Public Health.